Defects identification in raw jute fibre using convolutional neural network models
JOURNAL OF THE TEXTILE INSTITUTE(2024)
摘要
Defects in the raw jute are the second most important parameter in the jute grading system, as it affects the quality of yarn. Raw fibre with more defects tends to bring its price down and seriously affects the selling price of the product. Besides, it affects the process of fibre i.e. carding and spinning during yarn preparation. Currently, visual methods are in vogue in the market to assess the defects. Traditional approach of detection of defects is time consuming and needs specialized knowledge. In the present study, the feasibility of four state of art CNN models (GoogLeNet, AlexNet, ResNet50 and DarkNet53) were evaluated in defect detection with different hyper parameter settings. Models were evaluated for accuracy, precision, and F1-score. The accuracy of selected models ranged from 76.9% to 97.4%. Among all the models, DarkNet53 performed better than other models with an accuracy of 97.4%, precision 97.5% and recall 97.5% at 20 epochs and 16 batch sizes. DarkNet53 was tested again for random different defects images taken from the test set and found that the model can identify various defects effectively. The results of the present study showed that the pre-trained model coupled with a measuring approach can be used to measure the percentage of defects in raw jute fibre.
更多查看译文
关键词
Computer vision,CNN,defect identification,DarkNet53
AI 理解论文
溯源树
样例
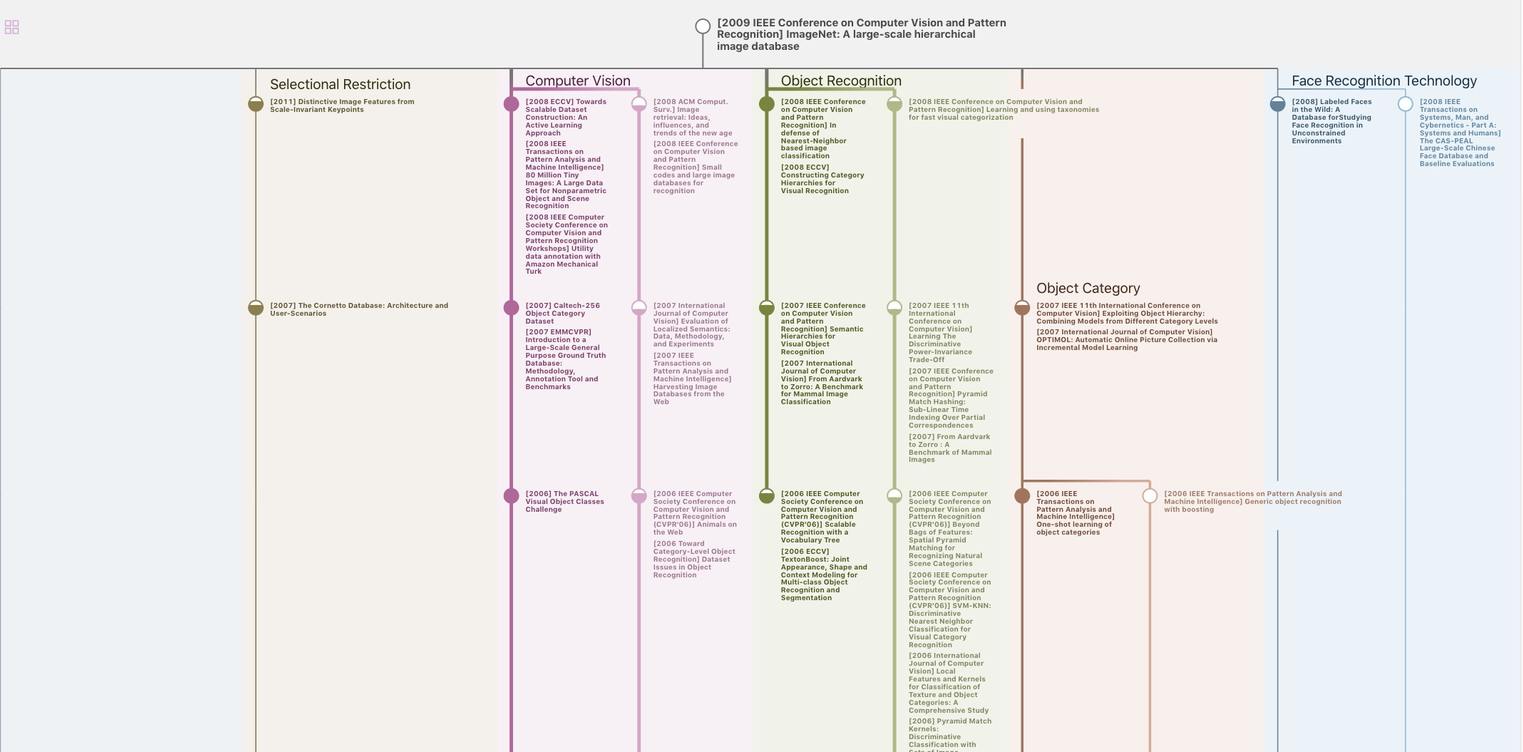
生成溯源树,研究论文发展脉络
Chat Paper
正在生成论文摘要