Multi-scale spectral-spatial dual-transformer network for hyperspectral image classification
INTERNATIONAL JOURNAL OF REMOTE SENSING(2023)
摘要
Deep learning methods have shown great advantages in hyperspectral image (HSI) classification tasks. In particular, convolutional neural network (CNN)-based methods for HSI classification have made great progress. However, it is difficult for CNN to process long-range spatial and spectral information. Recently, a novel deep learning model called transformer has demonstrated its potential to replace CNN in various classification tasks with its amazing performance. In this letter, a multi-scale spectral-spatial dual-transformer network ((MSDT)-D-3) is proposed to deeply consider the spectral-spatial features via transformer. Specifically, (MSDT)-D-3 consists of a feature pyramid network (FPN), a spectral transformer subnetwork (SPECT) and a spatial transformer subnetwork (SPAT). To utilize the complementary multi-scale characteristics, we introduce FPN to capture shallow-to-deep and spectral-spatial features. To improve the representational capacity in spatial and spectral domains, SPECT is exploited to extract long-range spectral correlation over local spectral features, and SPAT is designed to explore the contextual information for better refinement. Therefore, (MSDT)-D-3 is able to adaptively recalibrate the nonlinear interdependence of shallow-to-deep spectral-spatial features by merging the two subnetworks. Experiments on two widely used HSI datasets show that the (MSDT)-D-3 can outperform the state-of-the-art (SOTA) algorithm. The source codes will be available at .
更多查看译文
关键词
classification,multi-scale,spectral-spatial,dual-transformer
AI 理解论文
溯源树
样例
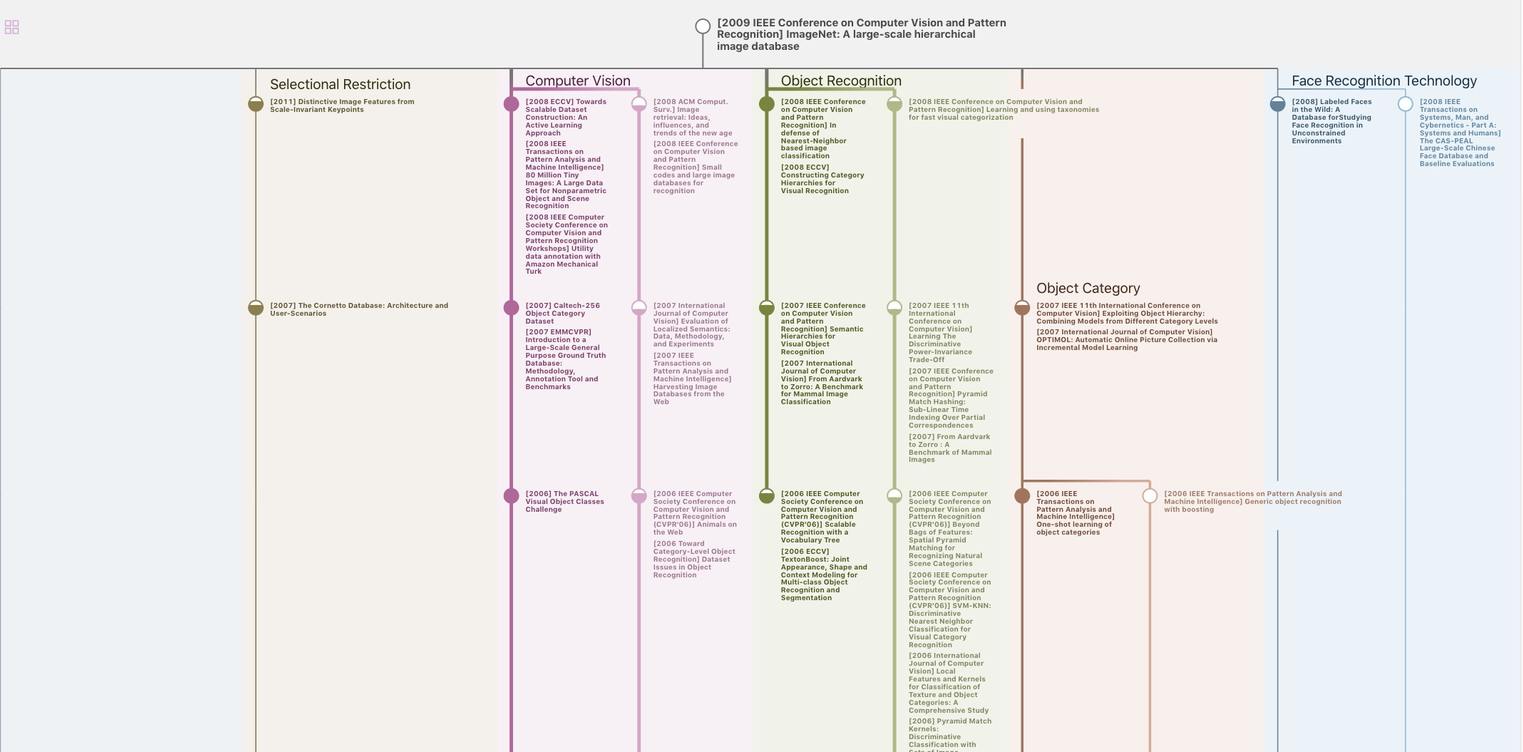
生成溯源树,研究论文发展脉络
Chat Paper
正在生成论文摘要