Quantile-Frequency Analysis and Deep Learning for Signal Classification
JOURNAL OF NONDESTRUCTIVE EVALUATION(2023)
摘要
This paper proposes a new method for signal classification based on a combination of deep-learning (DL) image classifiers and recently introduced nonlinear spectral analysis technique called quantile-frequency analysis (QFA). The QFA method converts a one-dimensional signal into a two-dimensional representation of quantile periodograms (QPER) which represent the signal’s oscillatory behavior in the frequency domain at different quantiles. With a moving window, the QFA method can also covert a signal into a sequence of such two-dimensional representations, called short-time quantile periodograms, that are localized in time to represent the signal’s time-dependent or nonstationary properties. The DL image classifiers take these representations as input for signal classification. The benefit of this QFA-DL classification method in comparison with the traditional frequency-domain method based on the power spectrum and spectrogram is demonstrated by a numerical experiment using real-world ultrasound signals from a nondestructive evaluation application.
更多查看译文
关键词
Discriminant analysis,Neural network,Power spectrum,Quantile periodogram,Quantile regression,Spectrogram,Time series
AI 理解论文
溯源树
样例
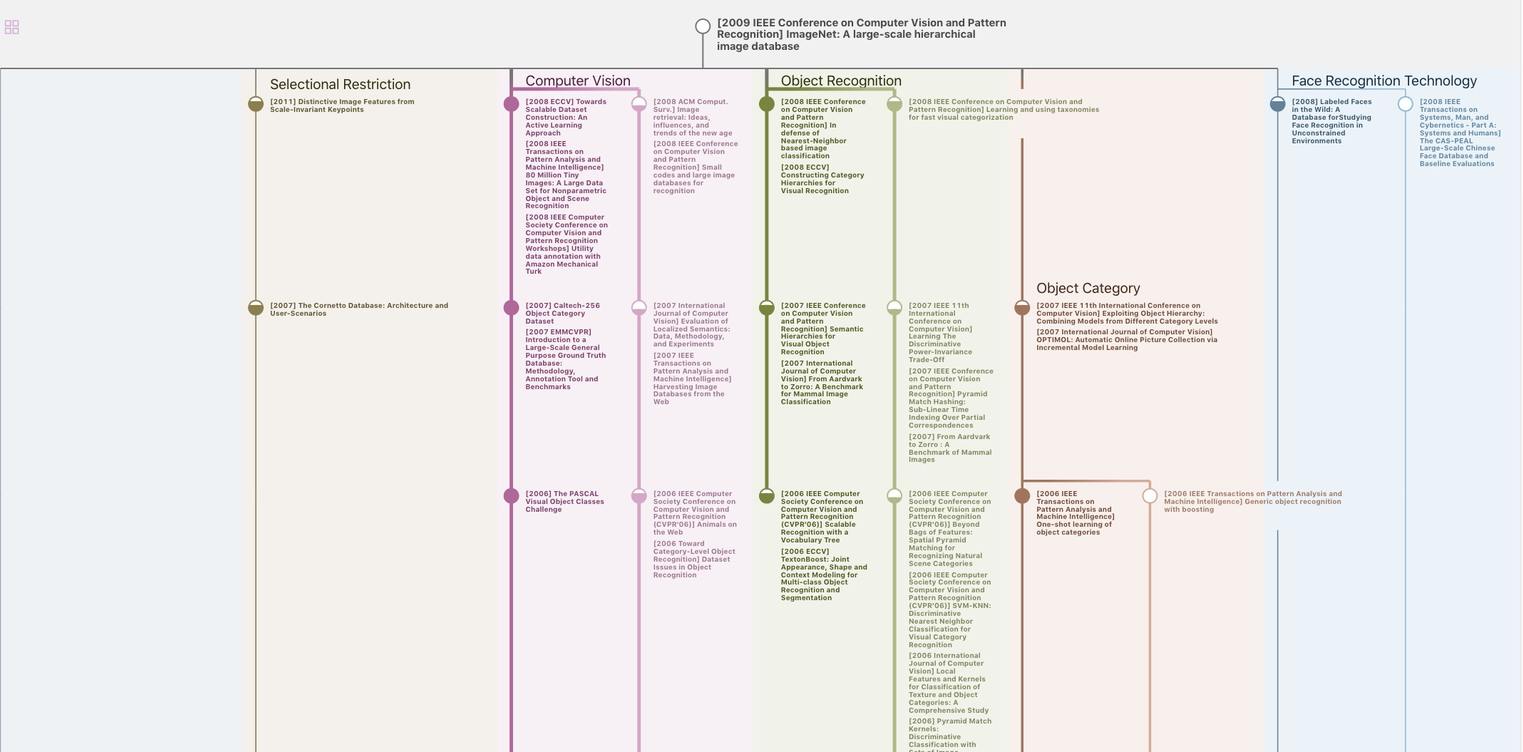
生成溯源树,研究论文发展脉络
Chat Paper
正在生成论文摘要