Spectroscopic characterisation of feedstock for copper smelters by machine-learning
CANADIAN METALLURGICAL QUARTERLY(2023)
摘要
A flame-emission spectrometer was built to determine the elemental composition of powdered minerals that are important in copper smelting processes. The feedstock, consisting of milled concentrate, was fed into an oxyacetylene flame without sample preparation. The elemental composition (Cu, Fe, S, Si, and Zn) was determined by applying an artificial neural network (ANN) to a set of emission spectra obtained from Cu and Fe pure elemental powders, five pure mineral powders of known composition and 30 binary mixtures of these mineral samples. The ANN model was able to accurately predict the Cu and Fe content of these mineral powders within better than 2% of the value obtained from ICP-OES. The analysis was repeated on 12 industrial samples with well-known compositions. Spectra from these samples were analyzed both in isolation of the reference minerals and together with the reference minerals, giving similar results.
更多查看译文
关键词
Machine learning, artificial neural network, copper flash furnace, feedstock analysis, flame emission spectroscopy, process control
AI 理解论文
溯源树
样例
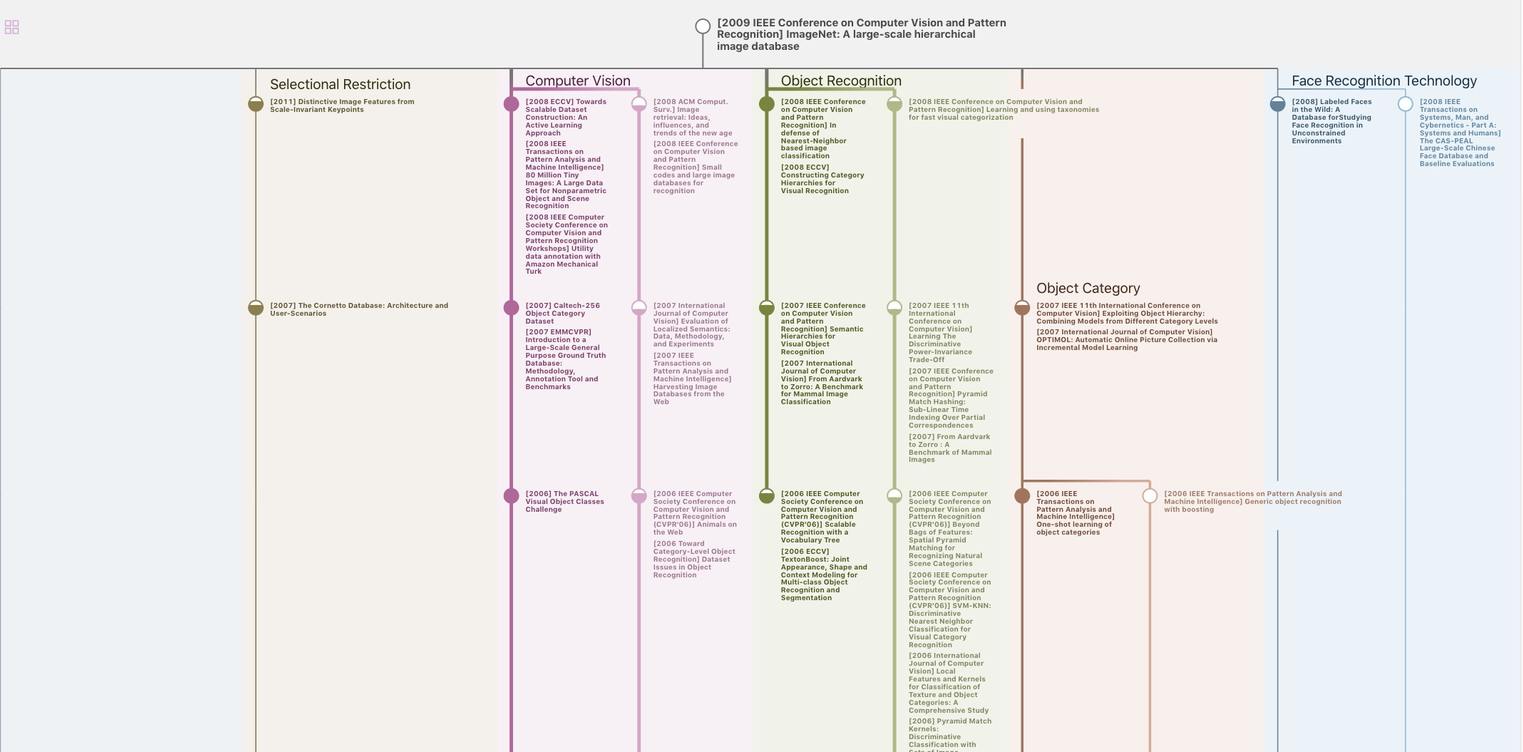
生成溯源树,研究论文发展脉络
Chat Paper
正在生成论文摘要