Improvement of accuracy and resilience in FHR classification via double trend accumulation encoding and attention mechanism
BIOMEDICAL SIGNAL PROCESSING AND CONTROL(2023)
摘要
Fetal heart rate monitoring based on fetal heart rate (FHR) is an important diagnostic tool for evaluating fetal health in late pregnancy. Currently, intelligent cardiotocography (CTG) classification based on FHR signals is a challenging area of research that can assist doctors in making clinical decisions to improve the efficiency and accuracy of pregnancy management. Most of the existing methods focus on converting FHR signals into image signals or classifying them based on the discreteness of the original signal without consideration of long-term characteristics and continuity of the signal, which causes scattered and incomplete signal feature extraction. This paper focuses on two major bottlenecks of deep learning algorithms for FHR time series classification: continuity of the FHR signal, and long-term features of the FHR signals. In order to solve these two problems, we propose a position encoding method called Double Trend Accumulation (DTA) through establish the big and small trends to extract the long-term features of FHR signals. In addition, we introduce timestamp scaling and value scaling to fusion the two long-term features after extraction of big trend and small trend. Based on DTA, we also design an improved model named Double Trend Accumulation Former (DTAF), which uses the structure of attention mechanism to classify the FHR signals. Finally, the ablation experiments, comparison experiments, and resilience experiments are used to verify the superiority and validity of the DTA method.
更多查看译文
关键词
Fetal heart rate, Cardiotocography monitoring, Deep learning, Time series, Trend
AI 理解论文
溯源树
样例
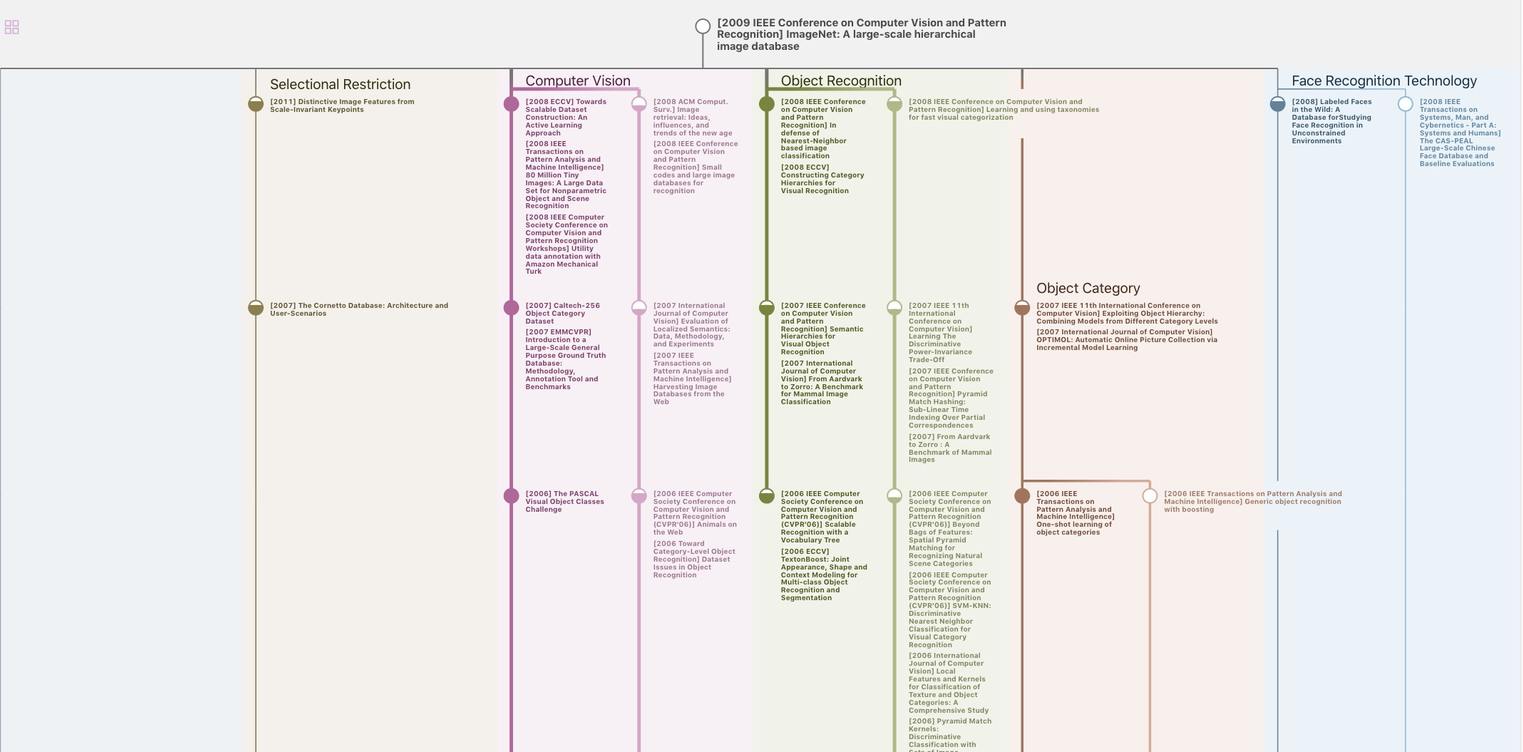
生成溯源树,研究论文发展脉络
Chat Paper
正在生成论文摘要