A Siamese CNN + KNN-Based Classification Framework for Non-intrusive Load Monitoring
JOURNAL OF CONTROL AUTOMATION AND ELECTRICAL SYSTEMS(2023)
摘要
Through the development of smart grids, programs such as demand side response, have been presented as auxiliary services to the real-time operation of distributed networks. In order to provide consumers information on their energy consumption, so that a modulation in consumption is possible, non-intrusive load monitoring has been introduced as an solution to this pattern recognition problem. Non-intrusive load monitoring enables the modeling of electrical loads connected to the low-voltage system, considering only a single measurement point. Presented state-of-the-art solutions though, consider availability of data as well as representation of all possible classes of the environment. This is of course a most conservative hypothesis, since in real-life applications availability of such data is much difficult, as well as the dynamic behavior of models is implicitly evolving in time. In this work a framework that uses neural Siamese networks with k -nearest neighbor clustering is presented toward non-intrusive load monitoring. Online learning feature is implemented, which relaxes the hypothesis of data requirements as well addresses the evolving nature of load profile. k -nearest clustering allows nonlinear characteristic space modelling. Test results using synthetics and real-life data show that the solution, besides obtaining a good generalizability in the classification, also obtained results with an accuracy of 95.77%.
更多查看译文
关键词
Non-intrusive load monitoring,Demand response,Siamese neural networks,k-nearest neighbor
AI 理解论文
溯源树
样例
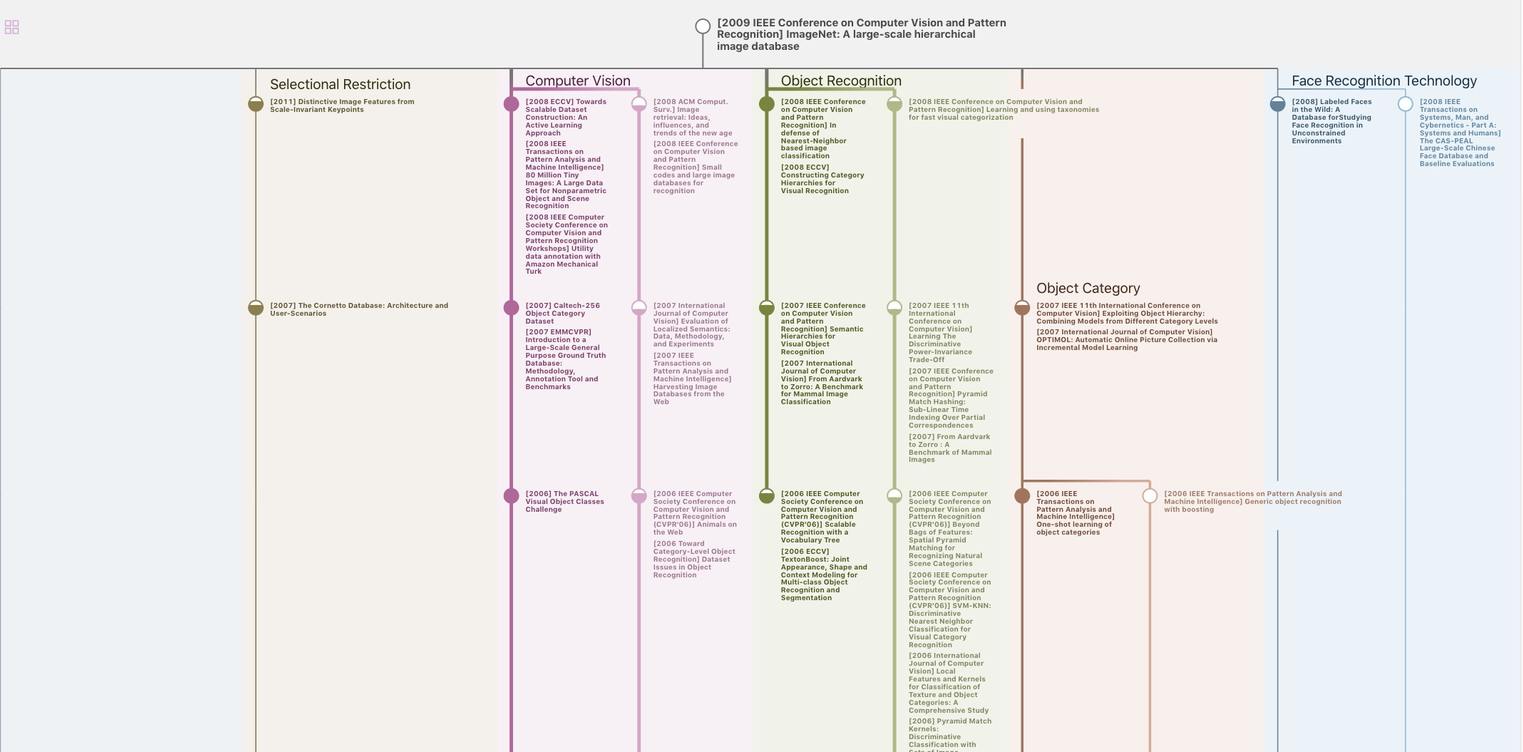
生成溯源树,研究论文发展脉络
Chat Paper
正在生成论文摘要