Metric-Based Meta-Learning for Cross-Domain Few-Shot Identification of Welding Defect
JOURNAL OF COMPUTING AND INFORMATION SCIENCE IN ENGINEERING(2023)
摘要
With the development of deep learning and information technologies, intelligent welding systems have been further developed, which achieve satisfactory identification of defective welds. However, the lack of labeled samples and complex working conditions can hinder the improvement of identification models. This paper explores a novel method based on metric-based meta-learning for the classification of welding defects with cross-domain few-shot (CDFS) problems. First, an embedding module using convolutional neural network (CNN) is applied to perform feature extraction and generate prototypes. The embedding module only contains one input layer, multiple convolutions, max-pooling operators, and batch normalization layers, which has the advantages of low computational cost and high generalization of images. Then the prototypical module using a prototypical network (PN) is proposed to reduce the influence of domain-shift caused by different materials or measurements using the representations in embedding space, which can improve the performance of few-shot welding defects identification. The proposed approach is verified on real welding defects under different welding conditions from the Camera-Welds dataset. For the K-shot classification on different tasks, the proposed method achieves the highest average testing accuracy compared to the existing methods. The results show the proposed method outperforms the model-based meta-learning (MAML) and transfer-learning method.
更多查看译文
关键词
metric-based,meta-learning,cross-domain,few-shot
AI 理解论文
溯源树
样例
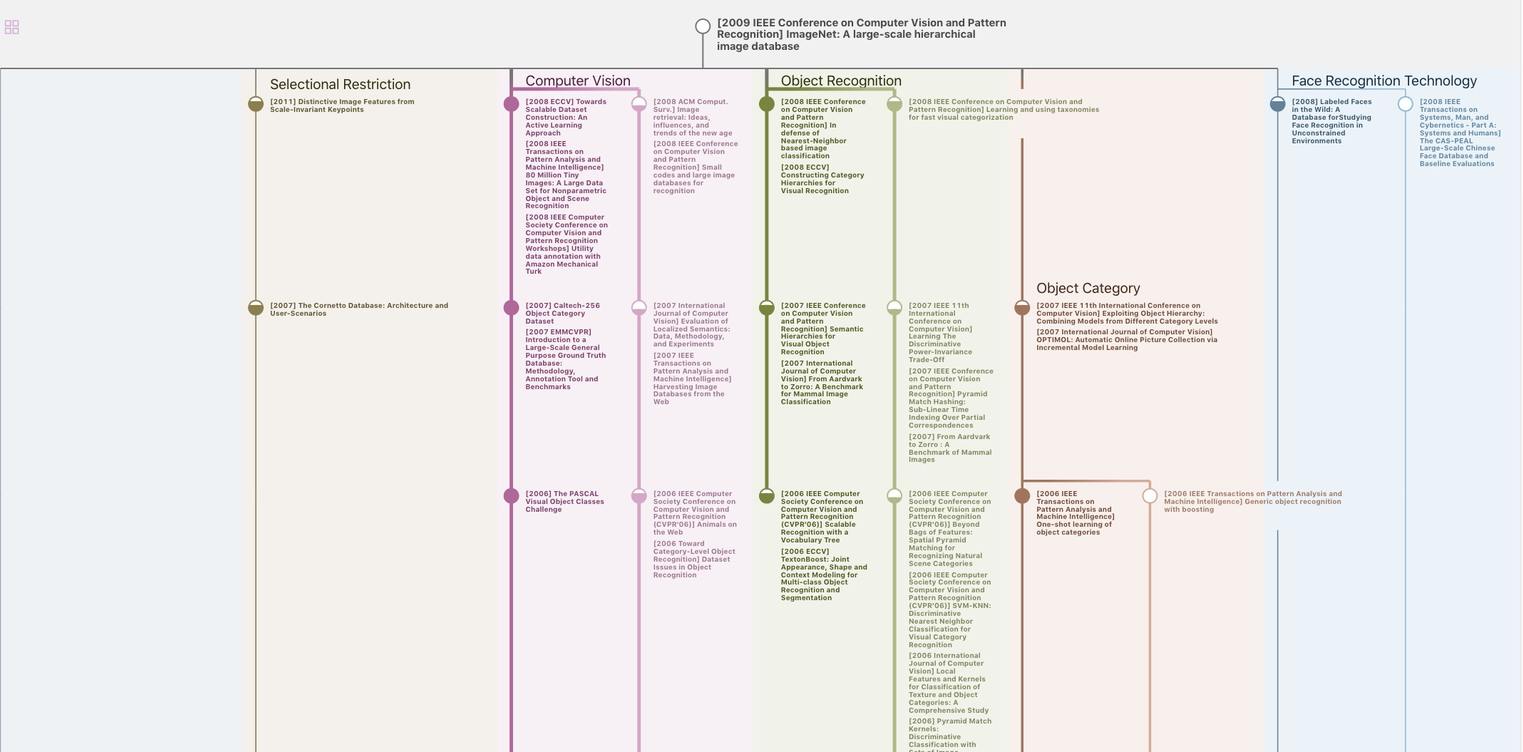
生成溯源树,研究论文发展脉络
Chat Paper
正在生成论文摘要