Development and validation of a deep learning model for prediction of intracranial aneurysm rupture risk based on multi-omics factor
EUROPEAN RADIOLOGY(2023)
摘要
Objective The clinical ability of radiomics to predict intracranial aneurysm rupture risk remains unexplored. This study aims to investigate the potential uses of radiomics and explore whether deep learning (DL) algorithms outperform traditional statistical methods in predicting aneurysm rupture risk. Methods This retrospective study included 1740 patients with 1809 intracranial aneurysms confirmed by digital subtraction angiography at two hospitals in China from January 2014 to December 2018. We randomly divided the dataset (hospital 1) into training (80%) and internal validation (20%). External validation was performed using independent data collected from hospital 2. The prediction models were developed based on clinical, aneurysm morphological, and radiomics parameters by logistic regression (LR). Additionally, the DL model for predicting aneurysm rupture risk using integration parameters was developed and compared with other models. Results The AUCs of LR models A (clinical), B (morphological), and C (radiomics) were 0.678, 0.708, and 0.738, respectively (all p < 0.05). The AUCs of the combined feature models D (clinical and morphological), E (clinical and radiomics), and F (clinical, morphological, and radiomics) were 0.771, 0.839, and 0.849, respectively. The DL model (AUC = 0.929) outperformed the machine learning (ML) (AUC = 0.878) and the LR models (AUC = 0.849). Also, the DL model has shown good performance in the external validation datasets (AUC: 0.876 vs 0.842 vs 0.823, respectively). Conclusion Radiomics signatures play an important role in predicting aneurysm rupture risk. DL methods outperformed conventional statistical methods in prediction models for the rupture risk of unruptured intracranial aneurysms, integrating clinical, aneurysm morphological, and radiomics parameters. Key Points • Radiomics parameters are associated with the rupture risk of intracranial aneurysms. • The prediction model based on integrating parameters in the deep learning model was significantly better than a conventional model. • The radiomics signature proposed in this study could guide clinicians in selecting appropriate patients for preventive treatment.
更多查看译文
关键词
Intracranial aneurysm,Stroke,Deep learning
AI 理解论文
溯源树
样例
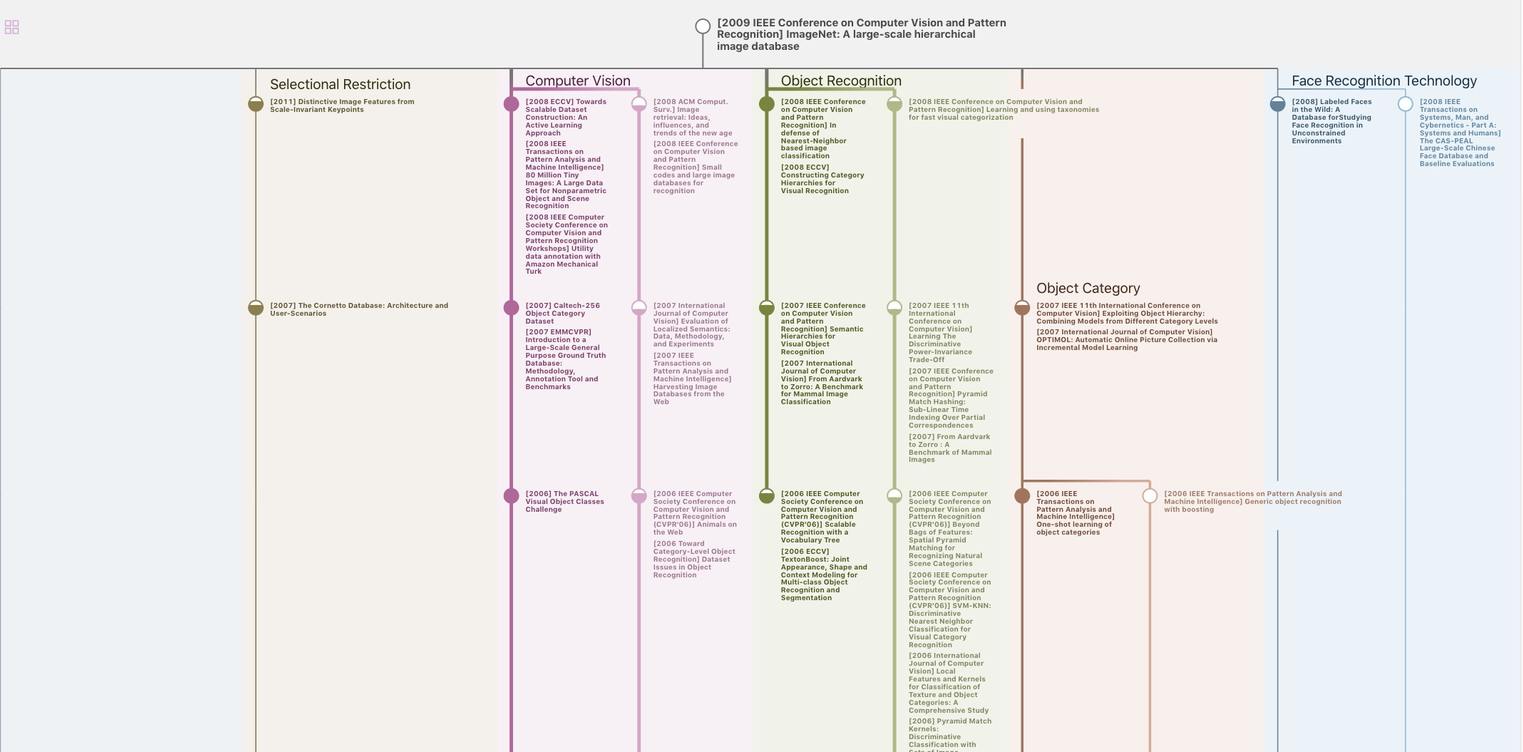
生成溯源树,研究论文发展脉络
Chat Paper
正在生成论文摘要