An ensemble method with DenseNet and evidential reasoning rule for machinery fault diagnosis under imbalanced condition
MEASUREMENT(2023)
摘要
Fault diagnosis is of significant importance for intelligent manufacturing as it can increase production efficiency and decrease the uncertain breakdown risk of machines. Previous studies have extensively utilized different types of shallow statistical features as well as deep representation features to comprehensively describe the fault in-formation for achieving better performances of fault diagnosis. However, making full use of the combination of such shallow statistical features and deep representation features under the class-imbalance situation in real -world applications becomes a challenge in current research of fault diagnosis. To remedy the above issue, an Imbalanced Ensemble Method with DenseNet and Evidential Reasoning Rule, namely IEMD-ER, is proposed to incorporate both human experience and machine wisdom for machinery fault diagnosis under the class -imbalance situation. To this end, the shallow statistical features with human experience are extracted by several signal processing techniques, while the modified DenseNet is adopted to extract the deep representation features with machine wisdom. Based on these features, multiple diverse base classifiers are produced by leveraging the importance of different features. The outputs of each base classifier are adaptively fused using the Evidential Reasoning Rule as the final fault diagnosis results. Extensive experiments are conducted on the real -world bearing datasets collected by Paderborn University to validate the proposed method. The experimental results proved the superiority of the proposed IEMD-ER.
更多查看译文
关键词
Fault Diagnosis, Deep Learning, Imbalance Learning, Evidential Reasoning Rule, DenseNet
AI 理解论文
溯源树
样例
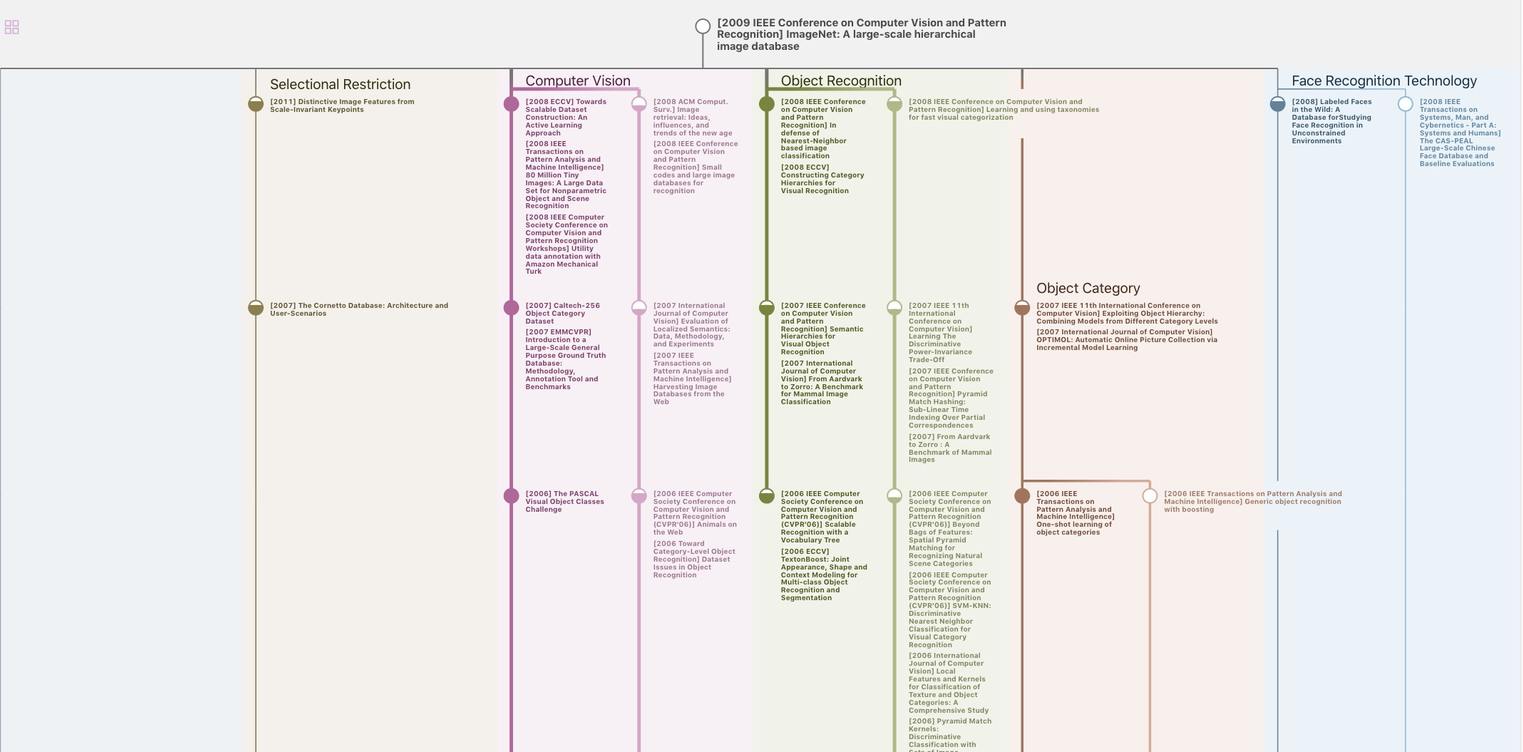
生成溯源树,研究论文发展脉络
Chat Paper
正在生成论文摘要