Deterministic sampling based on Kullback–Leibler divergence and its applications
STATISTICAL PAPERS(2023)
摘要
This paper introduces a new way to extract a set of representative points from a continuous distribution, which focuses on a method where the selection of points is essentially deterministic, with an emphasis on achieving accurate approximation when the size of points is small. These points are generated by minimizing the Kullback–Leibler divergence, which is an information-based measure of the disparity between two probability distributions. We refer to these points as Kullback–Leibler points. Based on the link between the total variation and the Kullback–Leibler divergence, we prove that the empirical distribution of Kullback–Leibler points converges to the target distribution. Additionally, we illustrate that Kullback–Leibler points have advantages in simulations when compared with representative points generated by Monte Carlo or other representative points methods. In addition, to prevent the frequent evaluation of complex functions, a sequential version of Kullback–Leibler points is proposed, which adaptively updates the representative points by learning about the complex or unknown functions sequentially. Two potential applications of Kullback-Leibler points in simulation of complex probability densities and optimization of complex response surfaces are discussed and demonstrated with examples.
更多查看译文
关键词
Bayesian computation,Computer experiments,Gaussian process model,Representative points,Space-filling design
AI 理解论文
溯源树
样例
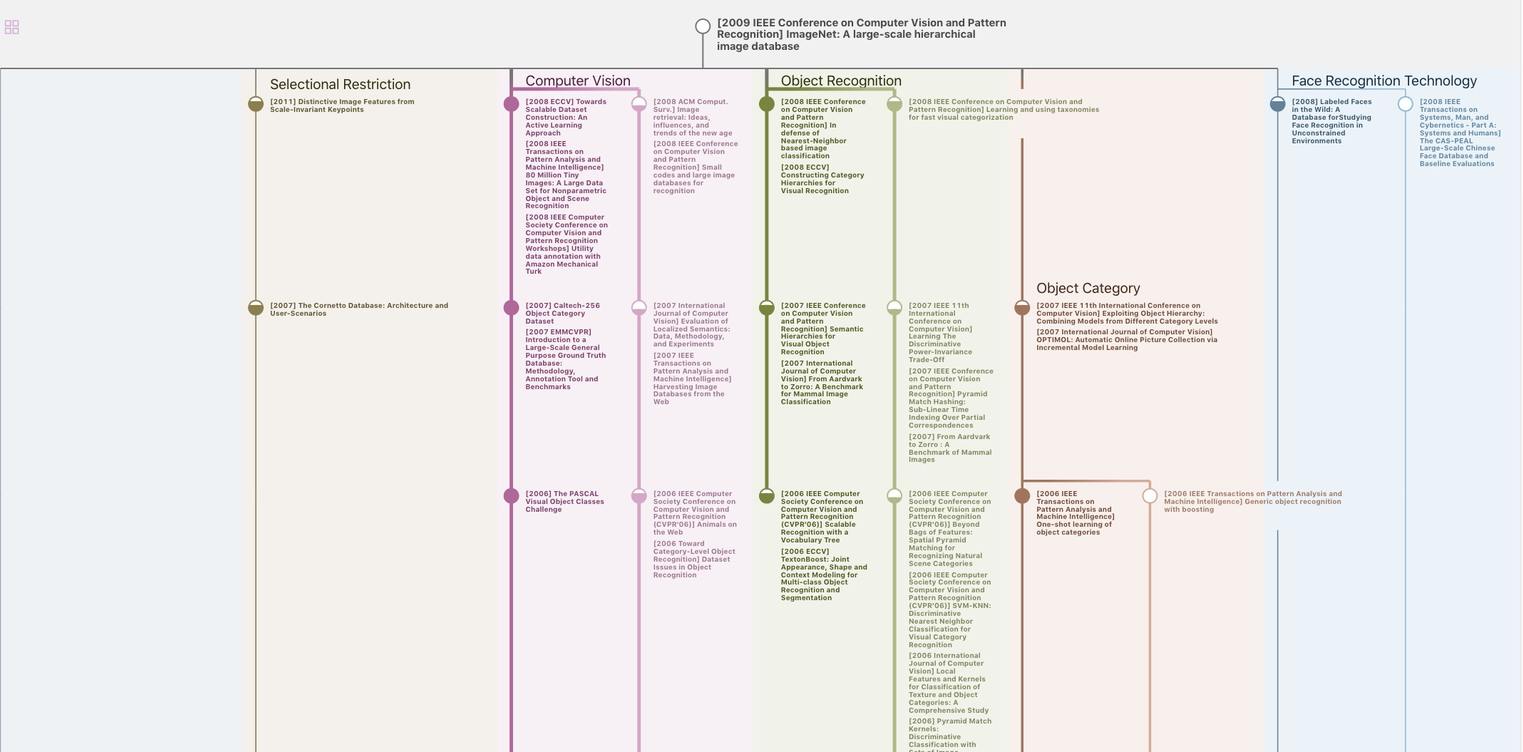
生成溯源树,研究论文发展脉络
Chat Paper
正在生成论文摘要