Surface roughness prediction using multi-source heterogeneous data and Bayesian quantile regression in milling process
JOURNAL OF MANUFACTURING PROCESSES(2023)
摘要
To quantify the influence of cutting parameters on surface roughness and improve the prediction accuracy, a novel method is proposed for surface roughness prediction in milling process. A quantile regression (QR) model is constructed using multi-source heterogeneous (MSH) data comprising monitoring signals and cutting pa-rameters within a Bayesian framework. The model parameters are then estimated using Gibbs sampling, and the predicted roughness and confidence interval (CI) can be obtained using the proposed Bayesian quantile model (BQR). Experimental results show that the proposed method has a prediction error of 15.05 %, which is 13.11 %, 0.01 %, and 1.7 % lower than least squares regression (LSR), back propagation network (BPN), and Bayesian linear regression (BLR), respectively. Through the comparison between predictions and experimental measure-ments, the proposed method has no great advantages over BPN in terms of prediction error, and is even 2.39 % higher than the prediction error of Support Vector Machine (SVM). However, the advantage of the proposed method compared with the above methods is that it can provide the analysis results of the influence of cutting parameters on surface roughness, which has practical significance in providing guidance for operators to reduce surface roughness in the milling process.
更多查看译文
关键词
surface roughness,bayesian quantile regression,multi-source
AI 理解论文
溯源树
样例
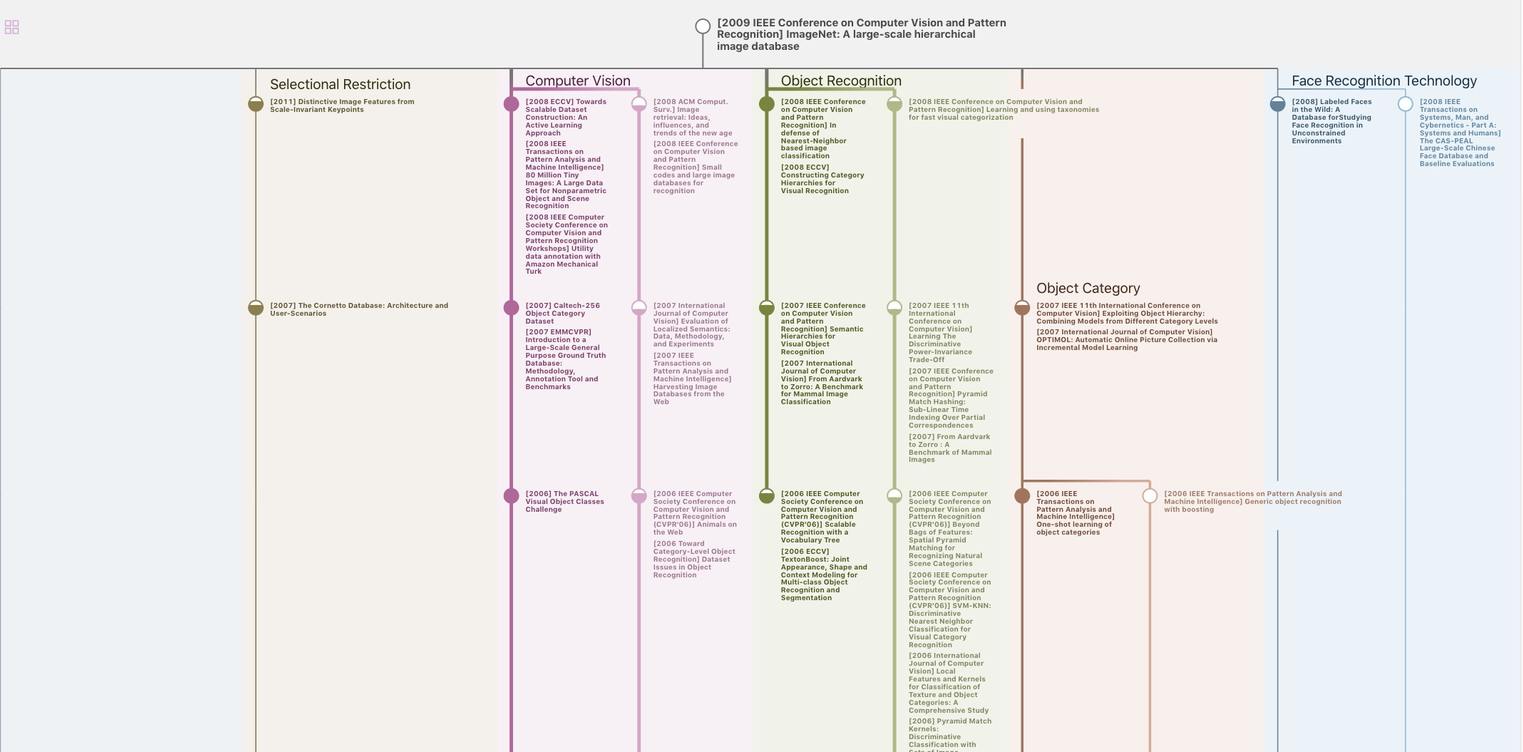
生成溯源树,研究论文发展脉络
Chat Paper
正在生成论文摘要