MAIM: a mixer MLP architecture for image matching
VISUAL COMPUTER(2024)
摘要
Recent advances in multilayer perceptron (MLP) models have provided new effective network architecture designs for computer vision tasks. Compared with convolutional neural networks (CNNs) and visual transformers, MLP-based visual backbones have less induction bias, which can improve the sample utilization efficiency and reduce computational costs. Therefore, we designed the Mixer MLP Architecture for Image-Matching (MAIM), which is a coarse to fine-level detector-free image-matching scheme. Accordingly, we constructed a mixer MLP architecture called Mixer-WMLP, which evenly divides the feature map into non-overlapping windows, spreads each window as a token, achieves the exchange of token information between spatial locations, channels features through a two-layer MLP structure in the coarse-level model, and then feeds the windows with dense fine-level matching, thereby producing the final matches. Furthermore, the implemented global field-of-view mixer MLP framework for image-matching incurs a low computational cost. By conducting experiments with indoor and outdoor relative poses, our MLP architecture is compared with CNN and transformer-based image-matching methods. Our method has significant advantages in terms of real-time performance and largely reduces computational cost, proving its effectiveness in image-matching tasks.
更多查看译文
关键词
Detector-free,Global field-of-view,Image-matching,Mixer MLP,Real-time
AI 理解论文
溯源树
样例
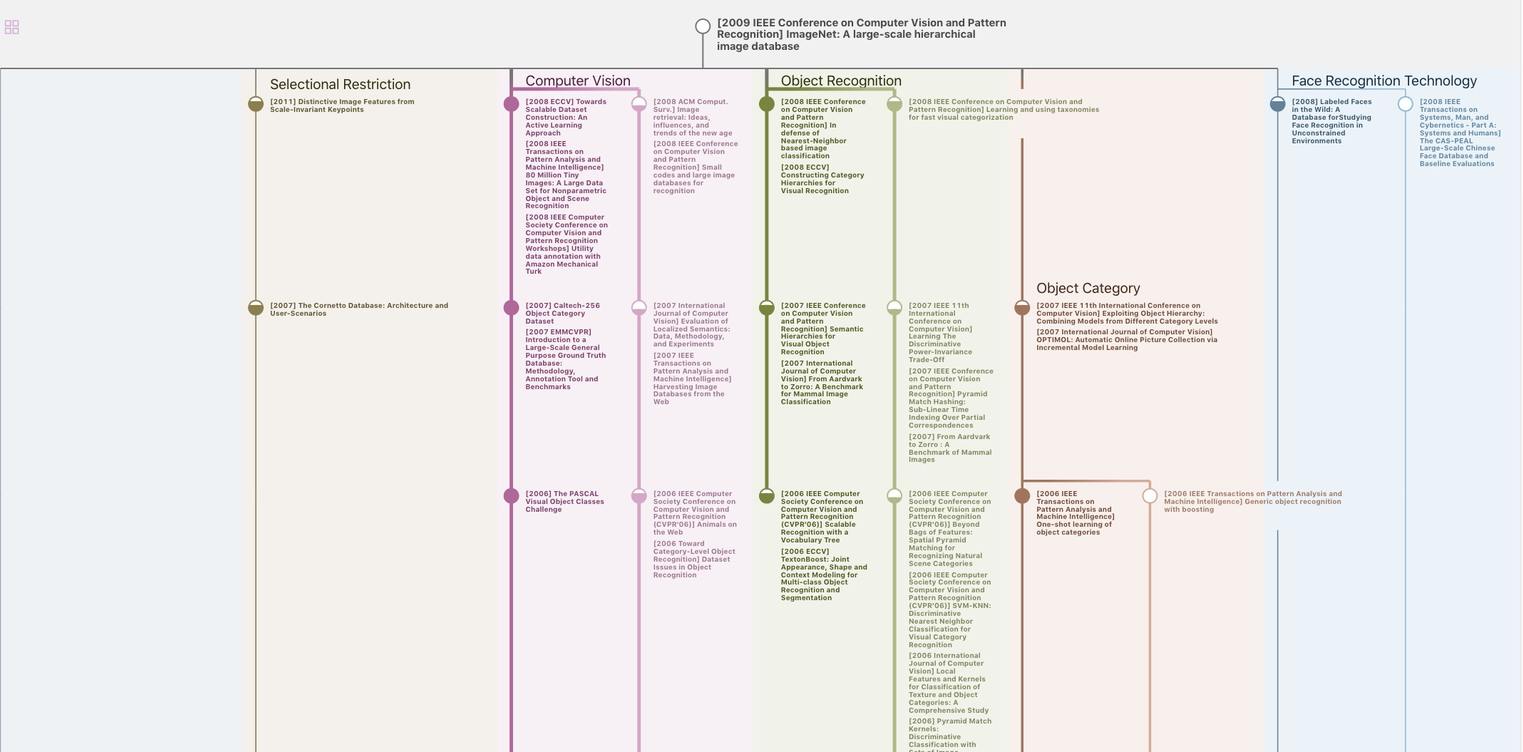
生成溯源树,研究论文发展脉络
Chat Paper
正在生成论文摘要