Unsupervised rail vehicle running instability detection algorithm for passenger trains (iVRIDA)
MEASUREMENT(2023)
摘要
Intelligently identifying rail vehicle faults instigating running instability from carbody floor acceleration is essential to ensure operational safety and reduce maintenance costs. However, the vehicle-track interaction's nonlinearities and scarcity of running instability occurrences complicate the task. The running instability is an anomaly in the vehicle-track interaction. Thus, we propose unsupervised anomaly detection and clustering al-gorithms based iVRIDA framework to detect and identify running instability and corresponding root cause. We deploy and compare the performance of the PCA-AD (baseline), Sparse Autoencoder (SAE-AD), and LSTM-Encoder-Decoder (LSTMEncDec-AD) model to detect the running instability occurrences.Furthermore, we deploy a k-means algorithm on latent space to identify clusters associated with root causes instigating instability. We deployed the iVRIDA framework on simulated and measured accelerations of European high-speed rail vehicles where SAE-AD and LSTMEncDec-AD models showed 97% accuracy. The proposed method contributes to smart maintenance by intelligently identifying anomalous vehicle-track interaction events.
更多查看译文
关键词
Vehicle hunting,Unsupervised machine learning,Sparse autoencoder,LSTM encoder decoder,Worn wheel,Failed yaw damper
AI 理解论文
溯源树
样例
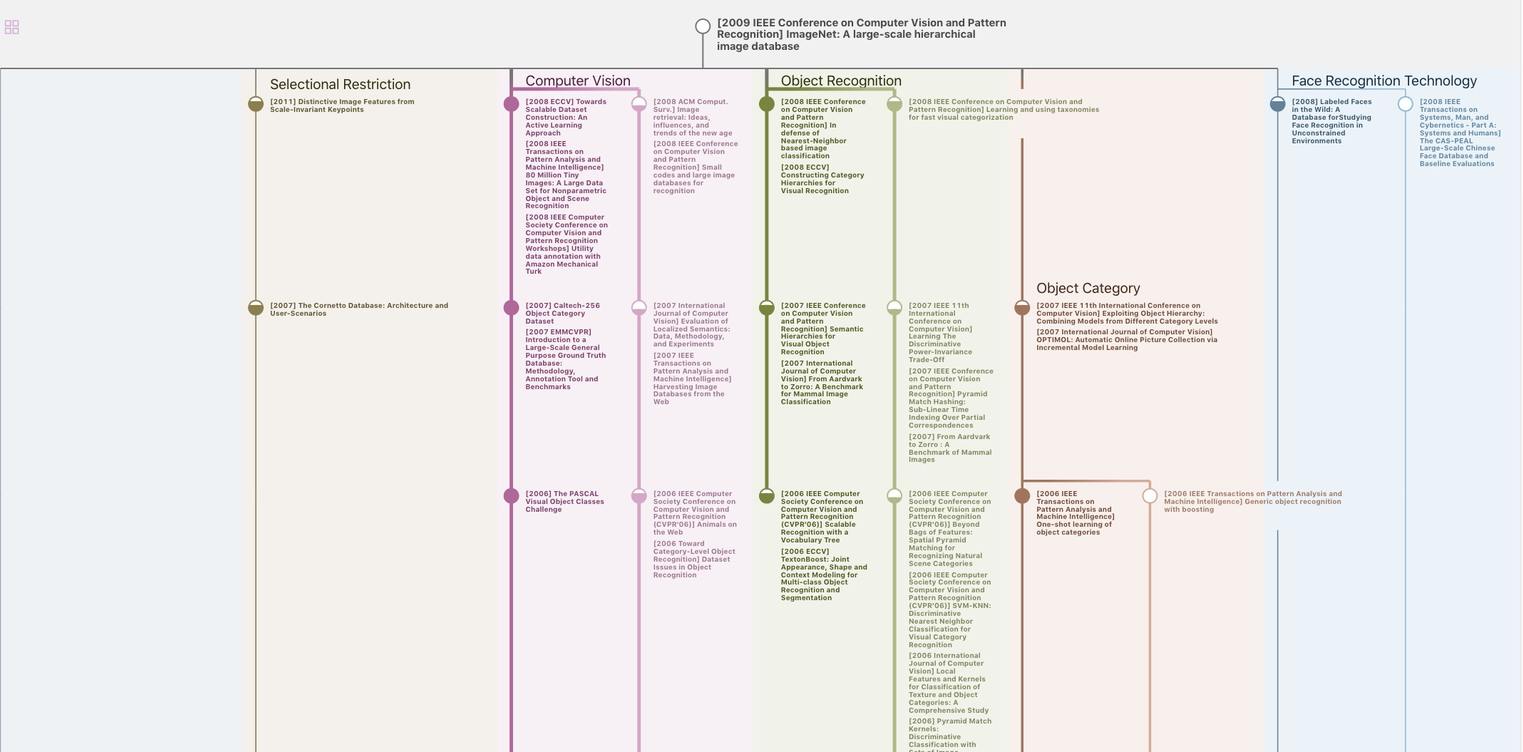
生成溯源树,研究论文发展脉络
Chat Paper
正在生成论文摘要