Random forest method for analysis of remote sensing inversion of aboveground biomass and grazing intensity of grasslands in Inner Mongolia, China
INTERNATIONAL JOURNAL OF REMOTE SENSING(2023)
摘要
The quantification of grassland above-ground biomass (AGB) and grazing intensity (GI) and their distribution in space is of great significance to grassland management and eco-conservation. Remote-sensing technology is widely applied, but it is difficult to measure accurately when monitoring GI. In this study, the neural network, random forest and statistical function models of the relationship between Landsat NDVI and AGB were constructed by field survey and literature data collection in Inner Mongolia grassland, China. By comparing the accuracy among the three models, we constructed a remote-sensing retrieving model of grass AGB. We also estimated the grassland AGB during the peak growing season (August) for Inner Mongolia. Frequency histograms were then made to identify AGB thresholds under four GI levels (light or ungrazed, moderate grazing, overgrazing and extreme grazing) for each of three grassland types (meadow steppe, typical steppe and desert steppe). This study shows that the random forest model simulates grass AGB more accurately than other models. The spatial distribution of AGB in Inner Mongolia grasslands showed a tendency of decreasing from southeast to northwest, with an increasing trend in the last 10 years. The four GI levels in 2021 accounted for 18%, 25%, 36% and 21% of the grasslands in Inner Mongolia, respectively, and over the last 10 years the GI first improved and then deteriorated. This study provides a guideline to remote monitoring for grassland AGB and GI, and supplies scientific support for sustainable management and grassland restoration of large-scale grasslands.
更多查看译文
关键词
Inner Mongolia Grassland, Aboveground biomass, Grazing intensity, Neural Networks, Random Forest
AI 理解论文
溯源树
样例
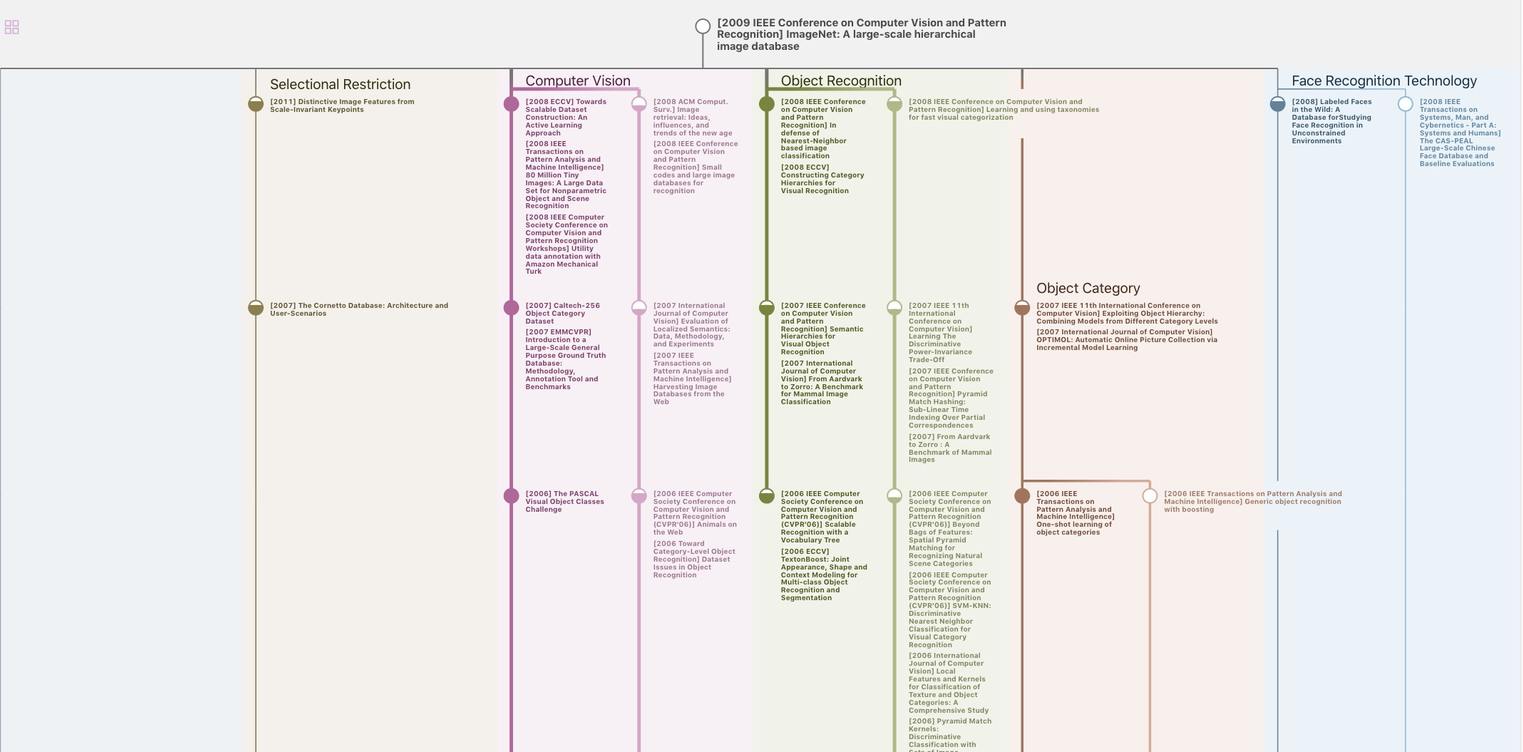
生成溯源树,研究论文发展脉络
Chat Paper
正在生成论文摘要