Scalable recursive state estimation for nonlinear two-dimensional complex networks with switching topology
INTERNATIONAL JOURNAL OF ROBUST AND NONLINEAR CONTROL(2023)
摘要
This paper investigates a scalable recursive state estimation method for nonlinear two-dimensional (2-D) stochastic complex networks. In these networks, the switching topology is modelled by random variables generated by the Bernoulli distribution. Considering the Extended Kalman Filter structure, we employ the Taylor series expansion to handle the nonlinear functions in the model. Hence, high-order terms emerging in the linearization process are modelled by norm-bounded parameter uncertainties. Due to the uncertainty of such a system, we obtain an upper bound for the estimation error covariance. Furthermore, we obtain an upper bound for the cross covariance to reduce the proposed filter's computational complexity and improve scalability. Within the established setting, next, the proposed filter is designed in such a way that the presented upper bounds are minimized. Finally, a numerical example is provided to illustrate the effectiveness of the proposed state estimation algorithm.
更多查看译文
关键词
scalable recursive state estimation,networks,nonlinear
AI 理解论文
溯源树
样例
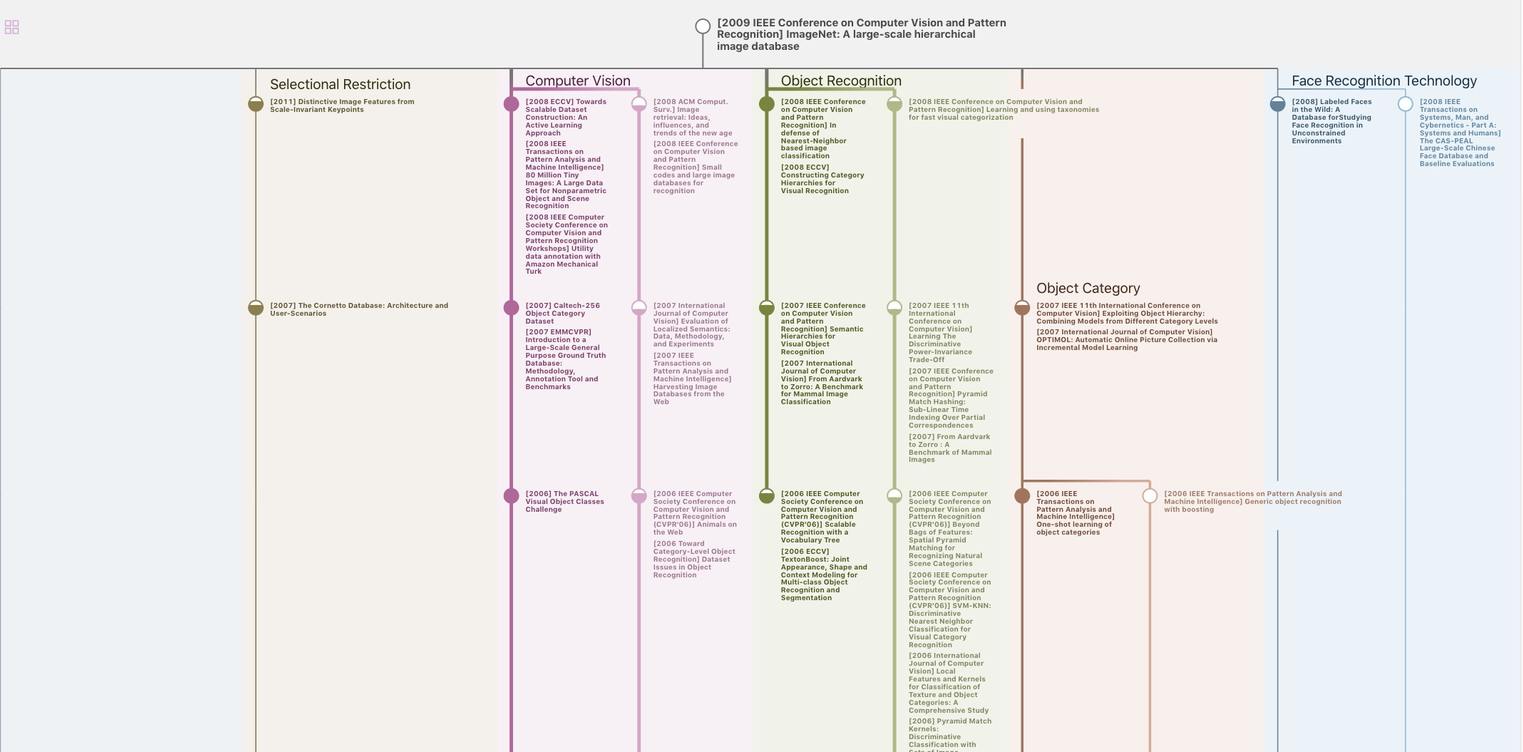
生成溯源树,研究论文发展脉络
Chat Paper
正在生成论文摘要