CNN-Based Surrogate for the Phase Field Damage Model: Generalization across Microstructure Parameters for Composite Materials
JOURNAL OF ENGINEERING MECHANICS(2023)
摘要
We investigate the generalization of a convolutional neural network (CNN)-based surrogate for the phase field model in predicting both damage and peak load under uniaxial tension, given the two-dimensional (2D) microstructure image of a unidirectional fiber-reinforced composite. We first discuss the phase field model and the numerical procedure to generate training and test data from synthetic microstructures with different volume fractions and fiber radiuses. We next present a two-stage approach for predicting peak load, achieved by first transforming a given fiber-encoded microstructure image to a continuous damage field; and second, predicting peak load from the damage field. A key finding is that the direct approach for predicting peak load from the microstructure image using a standard regression model fails to generalize. Instead, the damage field, even if imperfectly predicted, provides valuable cues for the CNN in generalizing across new microstructures within the range of parameters used in training. We describe several case studies to demonstrate the capability of the surrogate model to predict damage and peak load, and to interpolate over fiber radiuses and volume fractions.
更多查看译文
关键词
phase field damage model,microstructure parameters,composite materials,cnn-based
AI 理解论文
溯源树
样例
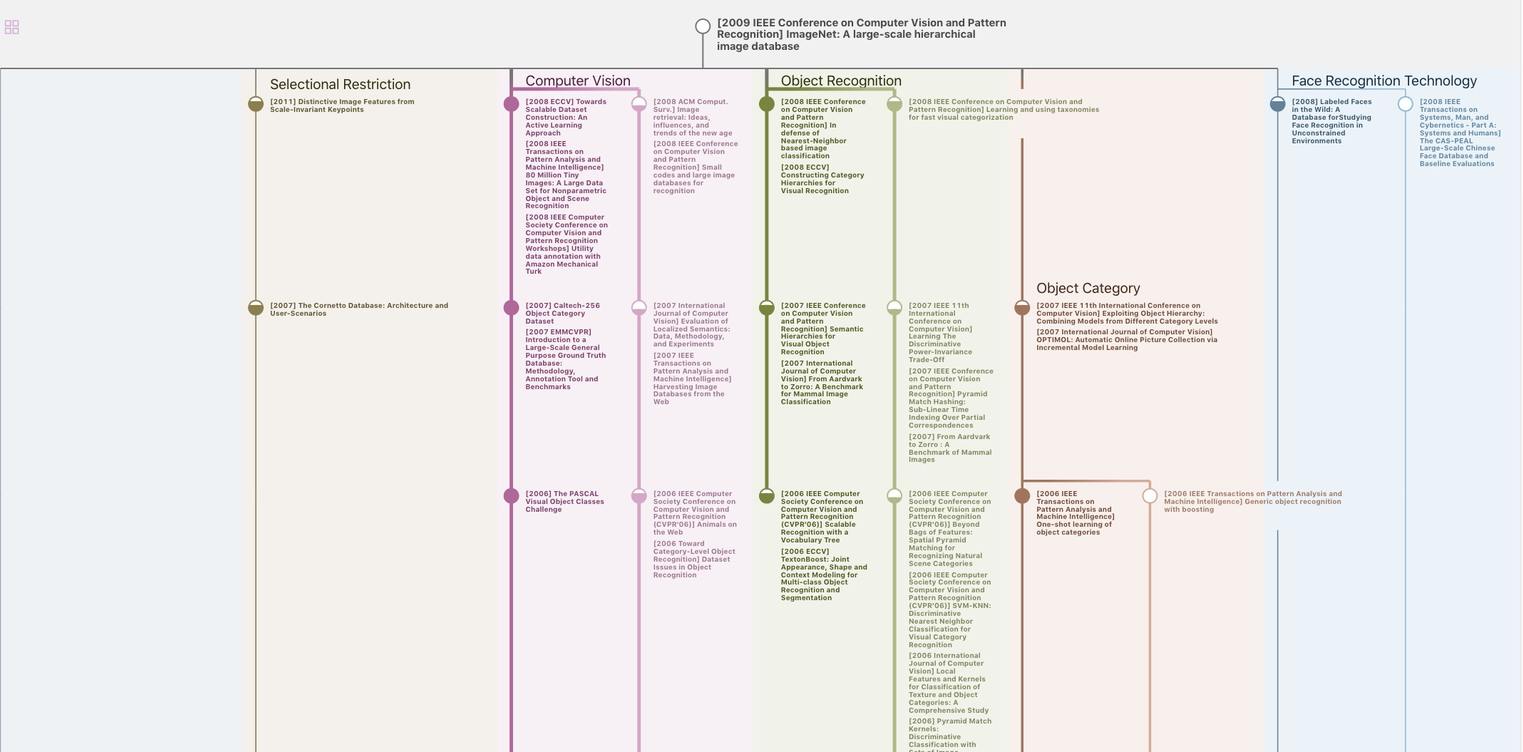
生成溯源树,研究论文发展脉络
Chat Paper
正在生成论文摘要