Bayesian Model Updating for Structural Dynamic Applications Combing Differential Evolution Adaptive Metropolis and Kriging Model
JOURNAL OF STRUCTURAL ENGINEERING(2023)
摘要
The Bayesian model updating approach has attracted much attention by providing the most probable values (MPVs) of physical parameters and their uncertainties. However, the Bayesian approach has challenges in high-dimensional problems and requires high computational costs in large-scale engineering structures dealing with structural dynamics. This study proposes a new Bayesian updating framework using the Differential Evolution Adaptive Metropolis (DREAM) algorithm to enhance the Bayesian approach's performance and computational efficiency. In addition, two time-saving strategies are proposed. Firstly, variance-based global sensitivity analysis is used to eliminate insignificant parameters to model responses and reduce model dimensionality. Secondly, a fast-running kriging model is employed as a surrogate of the time-consuming finite-element (FE) model to alleviate the computational burden. DREAM essentially is a multichain sampling method that runs different paths to seek all possible solutions and accurately approximate the posterior probability distribution function in the Bayesian approach. The proposed updating framework was demonstrated using one numerical example and a real-world cable-stayed pedestrian bridge. The results showed that the proposed method enables rationally identifying structural parameters and recovering dynamic responses. Compared with the traditional Bayesian approach without a surrogate model, the computational cost is orders of magnitude lower.
更多查看译文
关键词
evolution
AI 理解论文
溯源树
样例
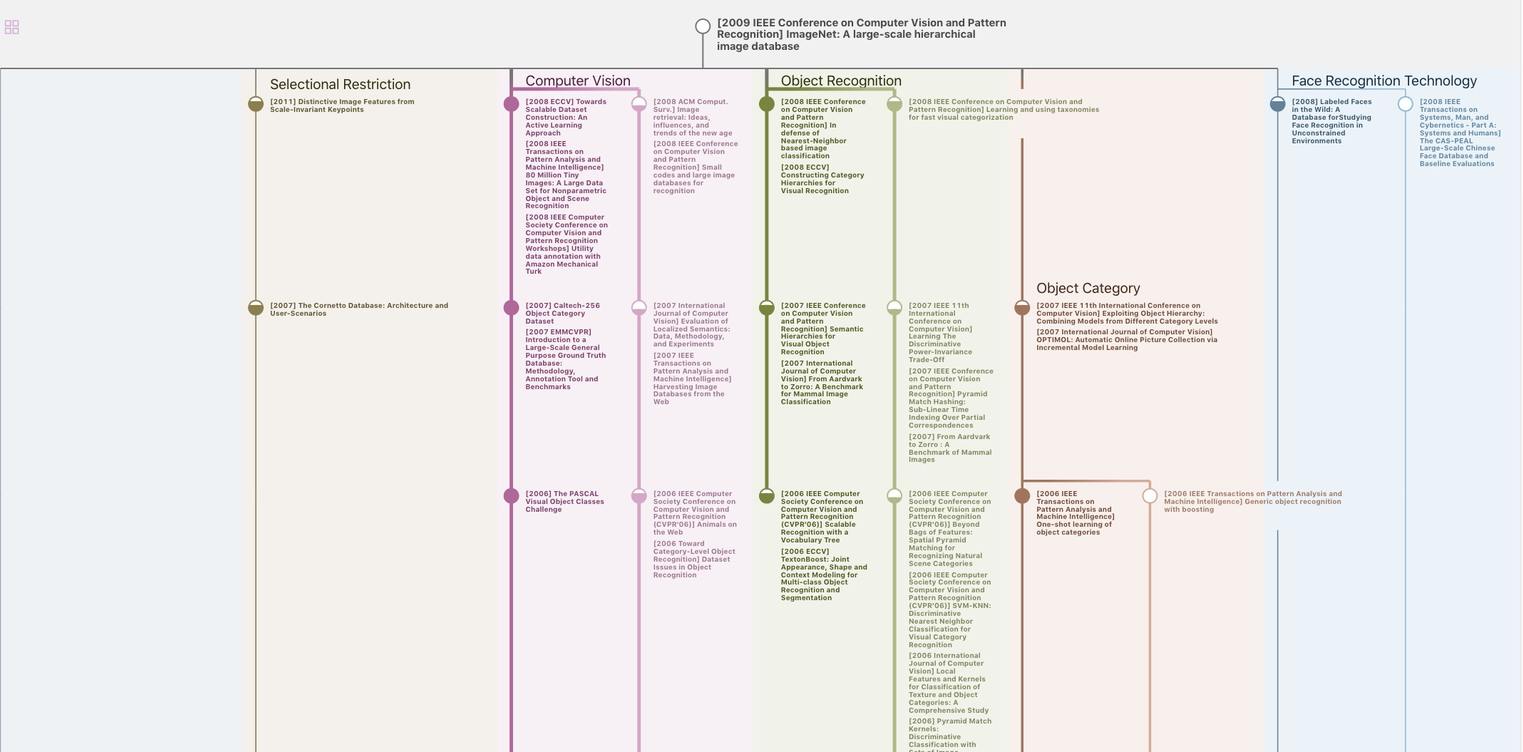
生成溯源树,研究论文发展脉络
Chat Paper
正在生成论文摘要