Data-Driven Development of Three-Dimensional Subsurface Models from Sparse Measurements Using Bayesian Compressive Sampling: A Benchmarking Study
ASCE-ASME JOURNAL OF RISK AND UNCERTAINTY IN ENGINEERING SYSTEMS PART A-CIVIL ENGINEERING(2023)
摘要
With the rapid development of computing and digital technologies recently, three-dimensional (3D) subsurface models for accurate site characterization have received increasing attention, for example, with various data-driven methods developed for 3D subsurface modeling. This leads to a need for validating the 3D modeling results obtained from each method and comparing the performance of different methods in a fair and consistent manner. To address this need, a benchmarking study, which is often used in machine learning (ML), is presented in this study to compare the performance of different 3D subsurface modeling methods in four aspects, including accuracy, uncertainty, robustness, and computational efficiency. A suite of performance metrics is proposed for the four aspects above. Multiple sets of real cone penetration test (CPT) data are compiled in the benchmarking study for quantifying performance of 3D modeling methods using sparse measurements as input, a typical scenario in geotechnical practice. The benchmarking study is illustrated using an in-house software package called Analytics of Sparse Spatial Data based on Bayesian compressive sampling/sensing (ASSD-BCS), which can directly generate high-resolution 3D random field samples (RFSs) from sparse measurements. The evaluation results show that ASSD-BCS provides accurate estimates with quantified uncertainty from sparse measurements. In addition, ASSD-BCS exhibits remarkably high computational efficiency and performs robustly under different benchmarking cases.
更多查看译文
关键词
bayesian compressive sampling,sparse measurements,models,data-driven,three-dimensional
AI 理解论文
溯源树
样例
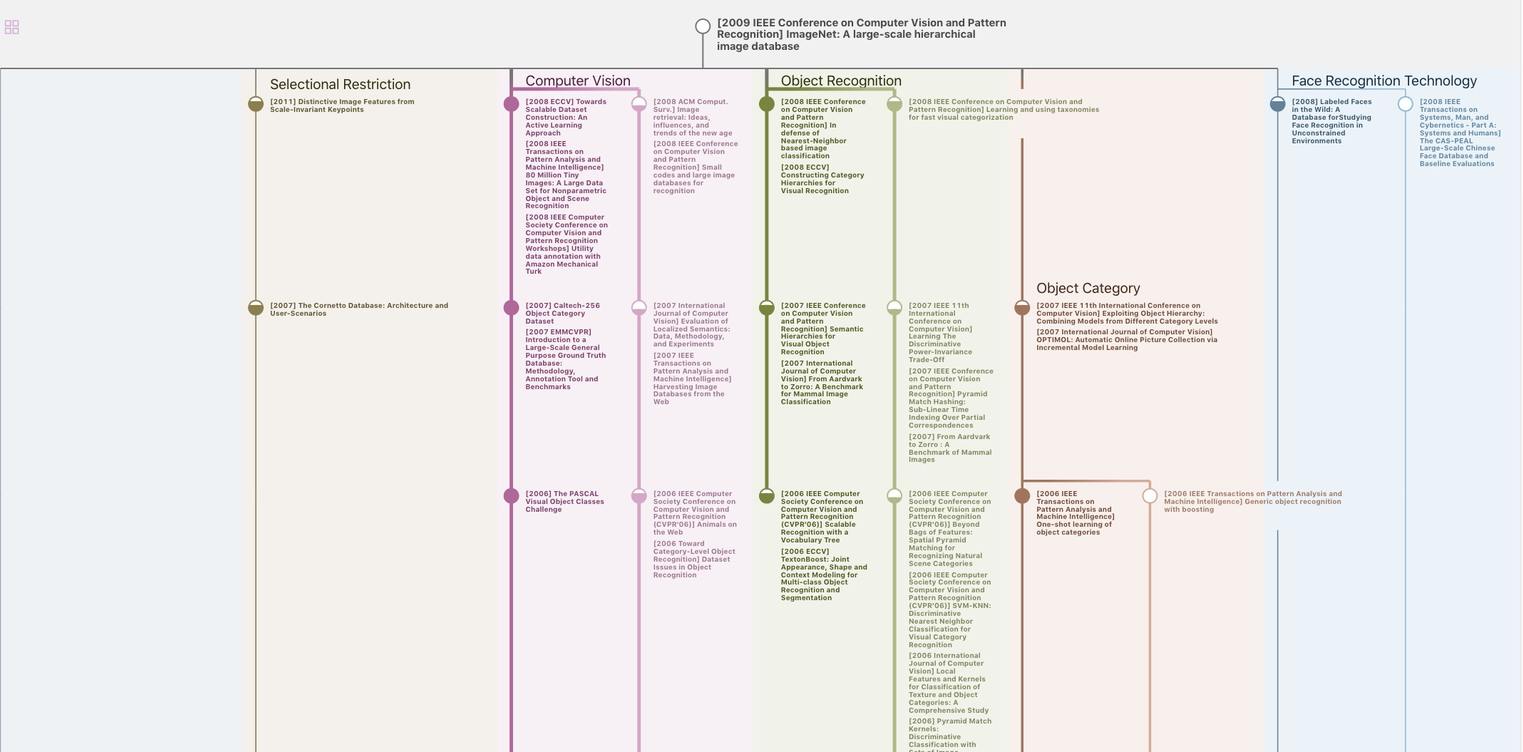
生成溯源树,研究论文发展脉络
Chat Paper
正在生成论文摘要