Aggregate-and-Excite Network for Image Super-Resolution
PROCEEDINGS OF THE WORLD CONFERENCE ON INTELLIGENT AND 3-D TECHNOLOGIES, WCI3DT 2022(2023)
摘要
Recently, convolution neural network (CNN)-based image super-resolution (SR) has achieved excellent performance. However, most existing works have ignored the importance of accurately utilizing contextual information. To solve this problem, we propose Aggregate-and-Excite block (AEB) with integration into our proposed Aggregate-and-Excite Network (AENet). AEB is functionally equivalent to feature extraction block. Each contains a global contextual information unit (GCIU) and a gate unit mechanism (GU), capturing the enriched contextual information for more powerful feature representations and emphasizing informative features according to the global contextual information. Compared with other methods, our AENet has achieved good performance through extensive experiments.
更多查看译文
关键词
super-resolution super-resolution,network,aggregate-and-excite
AI 理解论文
溯源树
样例
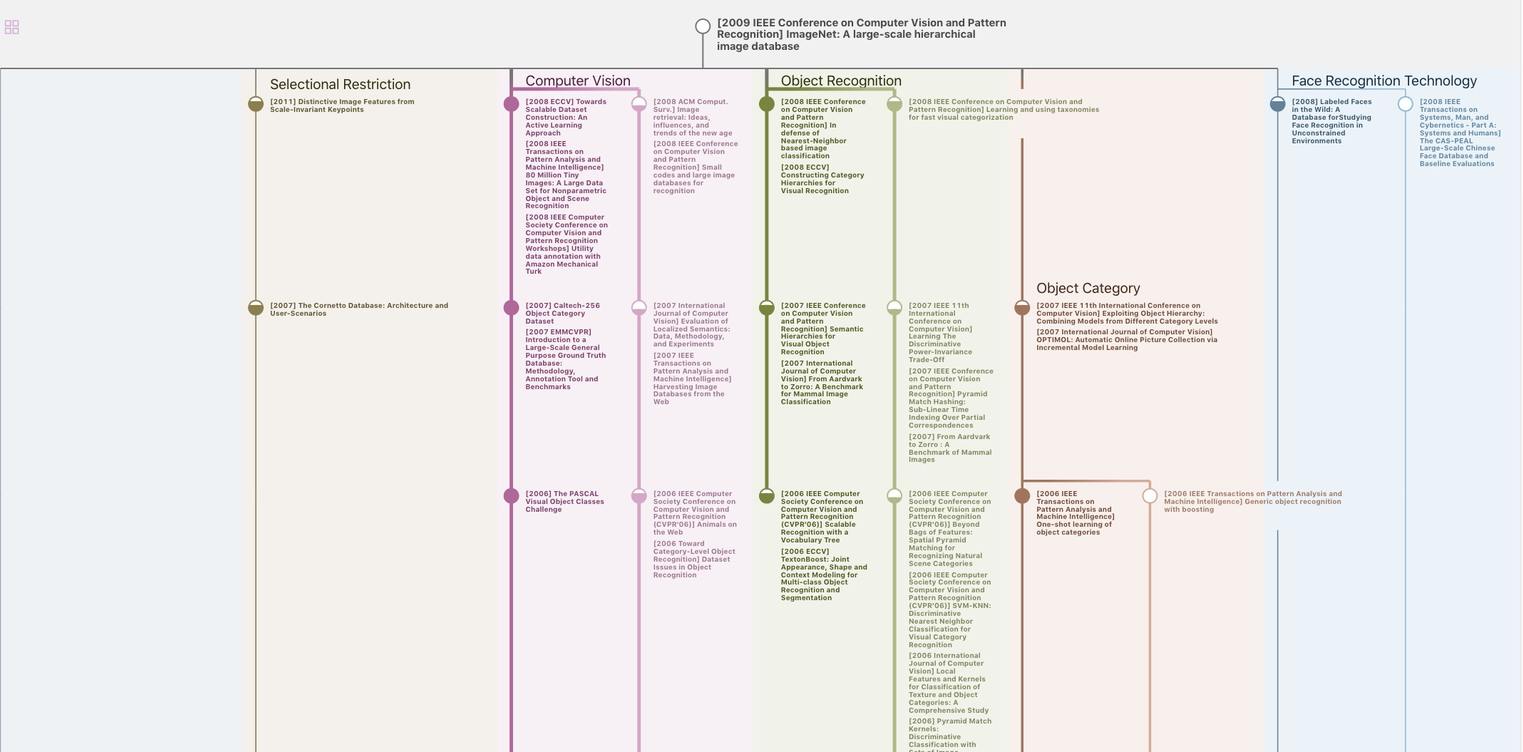
生成溯源树,研究论文发展脉络
Chat Paper
正在生成论文摘要