Systematic analysis of transcriptome signature for improving outcomes in lung adenocarcinoma
JOURNAL OF CANCER RESEARCH AND CLINICAL ONCOLOGY(2023)
摘要
Purpose The updated guidelines highlight gene expression-based multigene panel as a critical tool to assess overall survival (OS) and improve treatment for lung adenocarcinoma (LUAD) patients. Nevertheless, genome-wide expression signatures are still limited in real clinical utility because of insufficient data utilization, a lack of critical validation, and inapposite machine learning algorithms. Methods 2330 primary LUAD samples were enrolled from 11 independent cohorts. Seventy-six algorithm combinations based on ten machine learning algorithms were applied. A total of 108 published gene expression signatures were collected. Multiple pharmacogenomics databases and resources were utilized to identify precision therapeutic drugs. Results We comprehensively developed a robust machine learning-derived genome-wide expression signature (RGS) according to stably OS-associated RNAs (OSRs). RGS was an independent risk element and remained robust and reproducible power by comparing it with general clinical parameters, molecular characteristics, and 108 published signatures. RGS-based stratification possessed different biological behaviors, molecular mechanisms, and immune microenvironment patterns. Integrating multiple databases and previous studies, we identified that alisertib was sensitive to the high-risk group, and RITA was sensitive to the low-risk group. Conclusion Our study offers an appealing platform to screen dismal prognosis LUAD patients to improve clinical outcomes by optimizing precision therapy.
更多查看译文
关键词
Lung adenocarcinoma,Genome-wide expression signature,Machine learning,Overall survival,Precision therapy
AI 理解论文
溯源树
样例
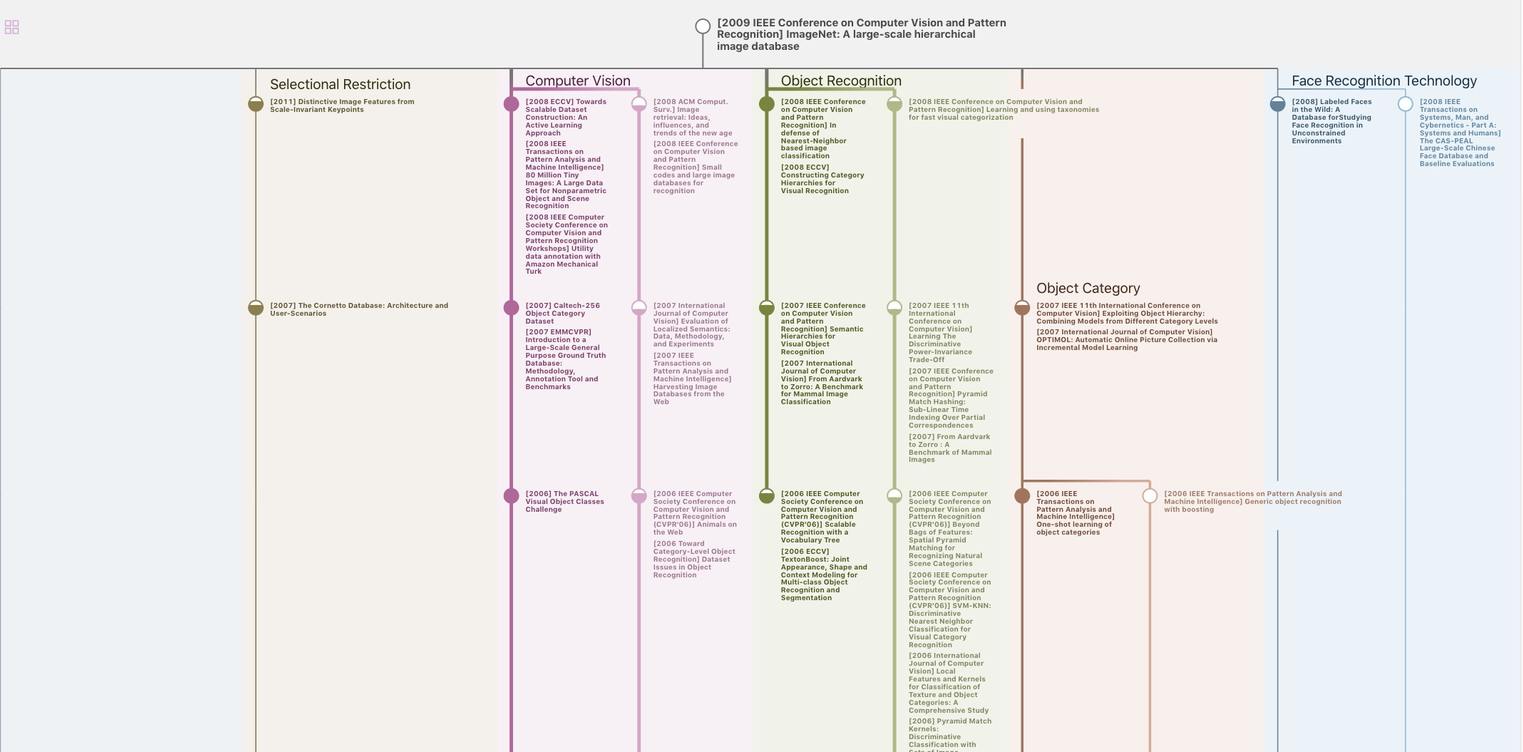
生成溯源树,研究论文发展脉络
Chat Paper
正在生成论文摘要