Streamflow Prediction in Poorly Gauged Watersheds in the United States Through Data-Driven Sparse Sensing
WATER RESOURCES RESEARCH(2023)
摘要
Many rivers and streams are ungauged or poorly gauged and predicting streamflow in such watersheds is challenging. Although streamflow signals result from processes with different frequencies, they can be "sparse" or have a "lower-dimensional" representation in a transformed feature space. In such cases, if this appropriate feature space can be identified from streamflow data in gauged watersheds by dimensionality reduction, streamflow in poorly gauged watersheds can be predicted with a few measurements taken. This study utilized this framework, named data-driven sparse sensing (DSS), to predict daily-scale streamflow in 543 watersheds across the contiguous United States. A tailored library of features was extracted from streamflow training data in watersheds within the same climatic region, and this feature space was used to reconstruct streamflow in poorly gauged watersheds and identify the optimal timings for measurement. Among different regions, streamflow in snowmelt-dominated and baseflow-dominated watersheds (e.g., Rocky Mountains) was more effectively predicted with fewer streamflow measurements taken. The prediction efficiency in some rainfall-dominated regions, for example, New England and the Pacific coast, increased significantly with an increasing number of measurements. The spatial variability of prediction efficiency can be attributed to the process-driven mechanisms and the dimensionality of watershed dynamics. Storage-dominated systems are lower-dimensional and more predictable than rainfall-dominated systems. Measurements taken during periods with large streamflow magnitudes and/or variances are more informative and lead to better predictions. This study demonstrates that DSS can be an especially useful technique to integrate ground-based measurements with remotely sensed data for streamflow prediction, sensor placement, and watershed classification.
更多查看译文
关键词
streamflow, prediction, ungauged watersheds, PUB, data-driven, signal reconstruction
AI 理解论文
溯源树
样例
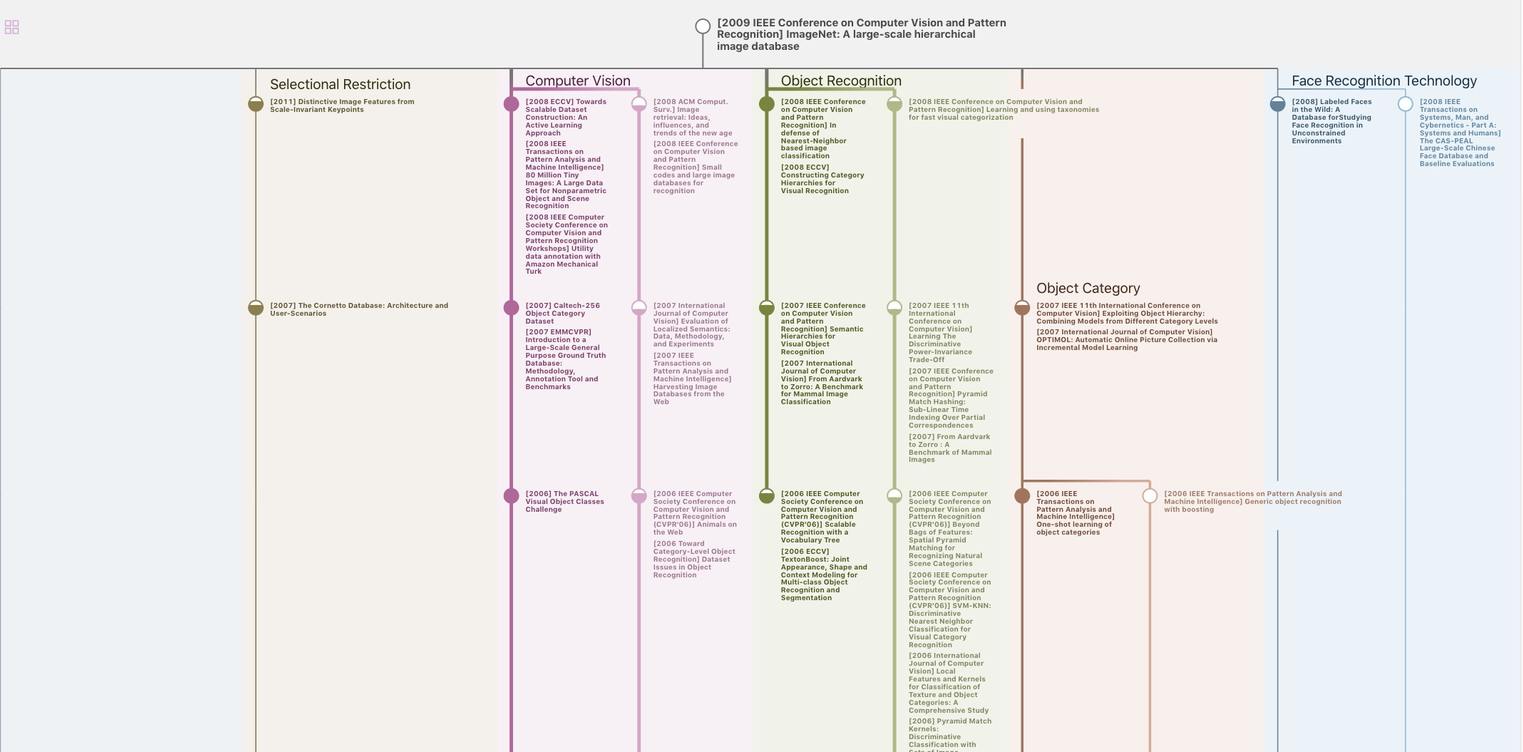
生成溯源树,研究论文发展脉络
Chat Paper
正在生成论文摘要