Predicting Workforce Engagement towards Digital Transformation through a Multi-Analytical Approach
SUSTAINABILITY(2023)
摘要
The shift towards sustainable and digital practices in organizations is transforming employees' mindsets and work performance. The digital transformation in academia is leading to meaningful changes in the behaviors and responsibilities of non-academic employees within organizations toward sustainable and responsible practices. By expounding insights into these views through social exchange theory (SET), this study aims to examine the key predictors of employee engagement (EE); namely, knowledge sharing (KS), employee mobility (EM), training and development (TD), and psychological empowerment (PE) in a digital workplace scenario. A quantitative survey based on convenience sampling was conducted to validate the research framework through partial least squares structural equation modelling (PLS-SEM). Accordingly, 205 responses were collected from the non-academic staff of universities in Klang Valley, Malaysia. Data analysis results showed that all hypotheses were significantly accepted. The impact of the model variables on employee engagement in digital transformation was found to be 75%, with employee mobility and knowledge sharing being the most prominent factors. Multigroup analysis (MGA) and importance-performance map analysis (IPMA) were additional analytical tools applied to reinforce the survey findings further and provide more comprehensive insights into employee engagement across different departments within the organization. The findings also showed the robustness of social exchange theory in digital business practices. This research offers novel and innovative perspectives on the impact of various factors (KS, EM, TD, PE) on employee engagement during digital transformation and how they mold employee behavior toward driving productive and responsible outcomes.
更多查看译文
关键词
employee engagement, digital transformation, non-academic staff, employee mobility, knowledge sharing, PLS-SEM, MGA, IPMA
AI 理解论文
溯源树
样例
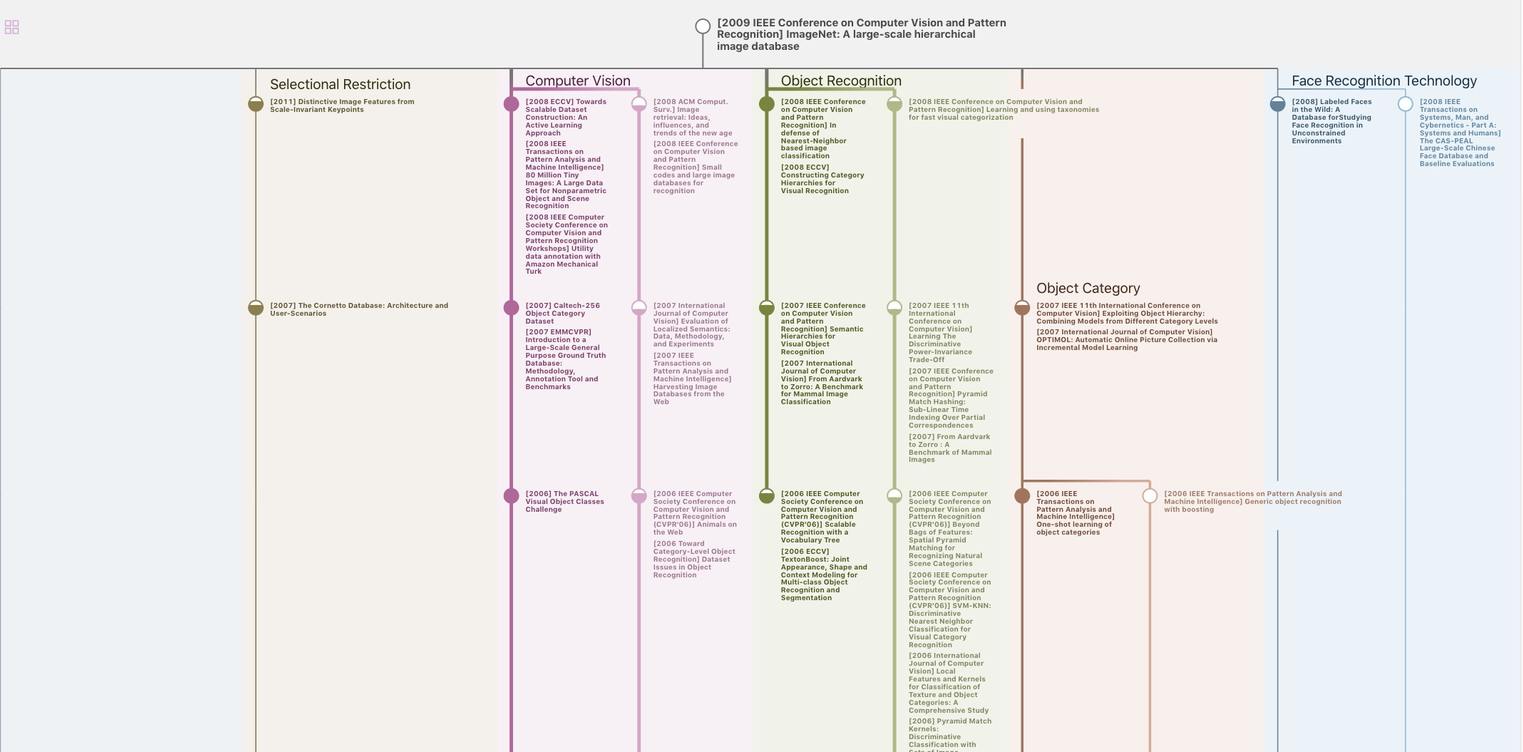
生成溯源树,研究论文发展脉络
Chat Paper
正在生成论文摘要