From predictive to energy-based maintenance paradigm: Achieving cleaner production through functional-productiveness
JOURNAL OF CLEANER PRODUCTION(2023)
摘要
The introduction of the Energy-Based Maintenance (EBM) practice in Sustainable Manufacturing attracted sig-nificant academic attention, especially considering imposed European initiatives (e.g., Green Deal). Although traditional Predictive Maintenance practice uses Machine Learning tools, it still relies on secondary (waste) energy indicators of a p -f curve (e.g., vibration, temperature). We introduce the notion of Functional-Productiveness (FP) for setting thresholds in detecting "Quasi-fault" events considering hydraulic power signal. Discretised hydraulic signal with Recursive Feature Elimination (RFE) is used for feature extraction. Support Vector Machine (SVM), Random Forest (RF), Partial Least Square Discriminant Analysis (PLS-DA) and t-Distributed Stochastic Network Embedding (t-SNE) algorithms are used for the feature selection process. The extracted features show latent degradation of a hydraulic control system of a Rubber Mixing Machine performed by binary classification {None, Quasi-Fault} with SVM, RF, PLS-DA and Logistic Regression (LR). The results show that latent degradation led to a 26% drop in hydraulic power output compared to the initial state, while the existing diagnostic practice of Lubricant Condition Monitoring failed to provide such insights. Consequently, the study suggests that traditional monitoring practices that rely on static p -f indicators are becoming obsolete, leading to unnecessary energy waste and power loss.
更多查看译文
关键词
Energy-based maintenance,Hydraulic system,t-SNE,Support vector machine,Random forest,Partial least square discriminant analysis,Logistic regression
AI 理解论文
溯源树
样例
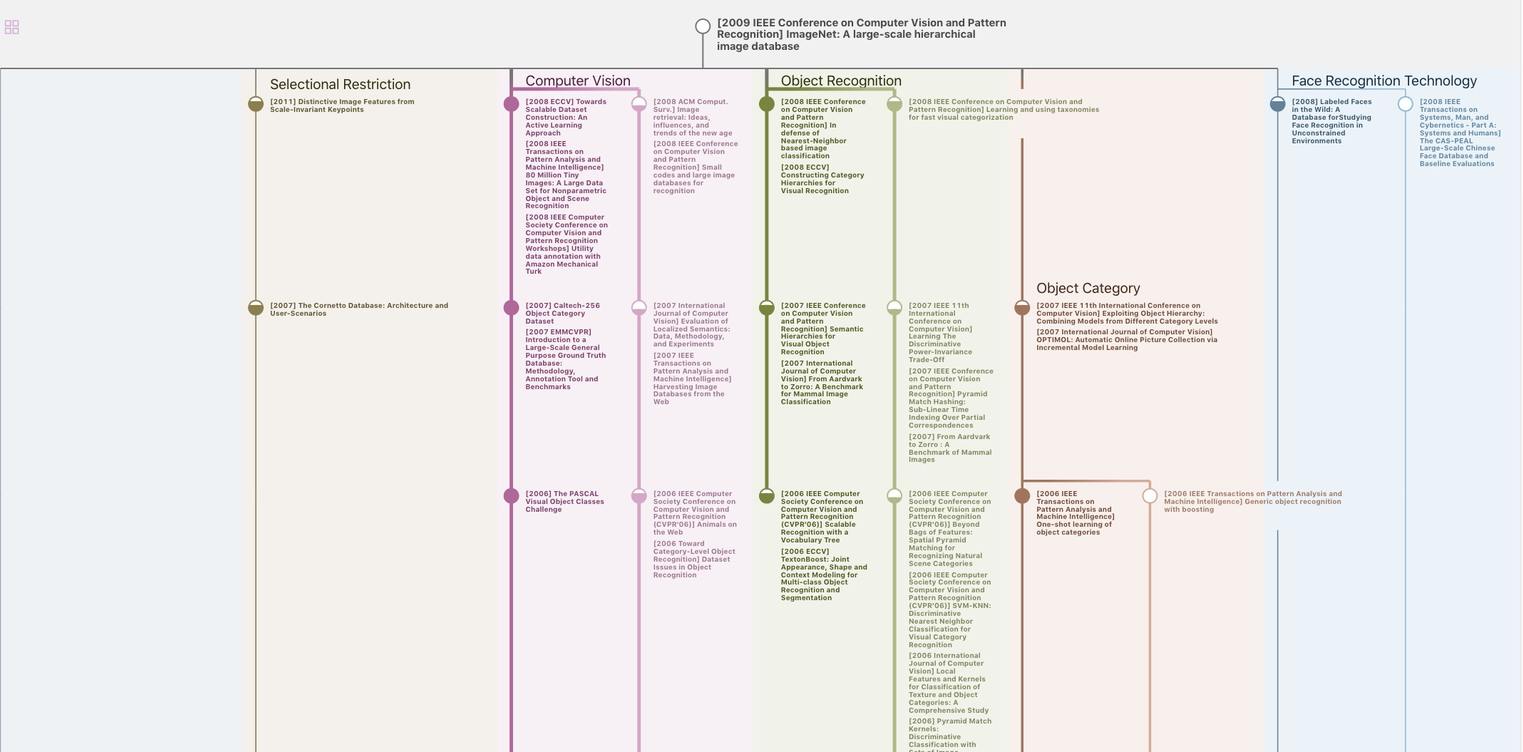
生成溯源树,研究论文发展脉络
Chat Paper
正在生成论文摘要