Large-scaled detection of COVID-19 from X-ray using transfer learning.
INTERNATIONAL JOURNAL OF IMAGING SYSTEMS AND TECHNOLOGY(2023)
摘要
The pandemic cause as a result of the outbreak of COVID-19 disease continues to burden the healthcare system despite several interventions using vaccines and other preventive measures. Healthcare settings adopted the use of reverse transcription-polymerase chain reaction (RT-PCR) which is hampered by so many challenges such as miss-diagnosis, false positive results, high cost, especially for those in remote and rural areas, the need for trained medical pathologists, the use of chemicals, and a lack of point-of-care detection. The use of radiographic images as an alternative or confirmatory approach has offered medical experts another option, but has some limitations, such as misinterpretation, and can be tedious for analyzing thousands of cases. In order to bridge this gap, we applied two AlexNet models for the classification of different types of pneumonia, including COVID-19 using X-ray. Considering the fact that the majority of articles in the literature reported binary classifications of radiographic images. This article utilizes X-ray images for classification of COVID-19, non-COVID-19 viral pneumonia, bacterial pneumonia, and normal cases using the AlexNet-SoftMax classifier and the AlexNet-SVM classifier. The research also evaluates performance based on 5k-fold and 10k fold cross validation (CV). The results achieved in terms of accuracy, sensitivity, and specificity based on 70:30 partition, 5k, and 10k CV have shown that the models outperformed the majority of the state-of-the-art deep learning architectures.
更多查看译文
关键词
AlexNet,COVID-19,CT-scan,deep learning,SARS-CoV-2,SVM,X-ray
AI 理解论文
溯源树
样例
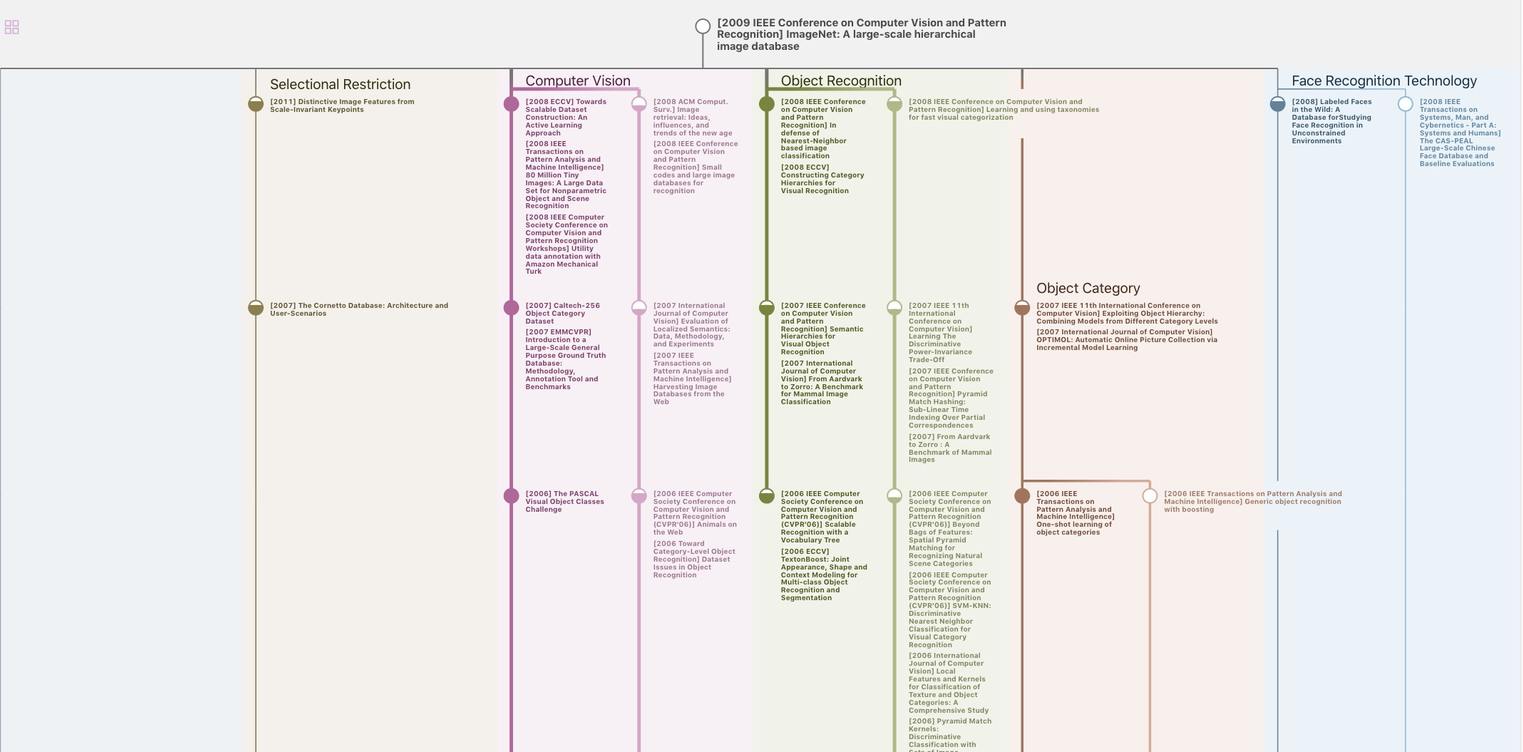
生成溯源树,研究论文发展脉络
Chat Paper
正在生成论文摘要