Machine learning for shock compression of solids using scarce data
JOURNAL OF APPLIED PHYSICS(2023)
摘要
Data-driven machine learning techniques can be useful for the rapid evaluation of material properties in extreme environments, particularly in cases where direct access to the materials is not possible. Such problems occur in high-throughput material screening and material design approaches where many candidates may not be amenable to direct experimental examination. In this paper, we perform an exhaustive examination of the applicability of machine learning for the estimation of isothermal shock compression properties, specifically the shock Hugoniot, for diverse material systems. A comprehensive analysis is conducted where effects of scarce data, variances in source data, feature choices, and model choices are systematically explored. New modeling strategies are introduced based on feature engineering, including a feature augmentation approach, to mitigate the effects of scarce data. The findings show significant promise of machine learning techniques for design and discovery of materials suited for shock compression applications.
更多查看译文
关键词
shock compression,machine learning,solids
AI 理解论文
溯源树
样例
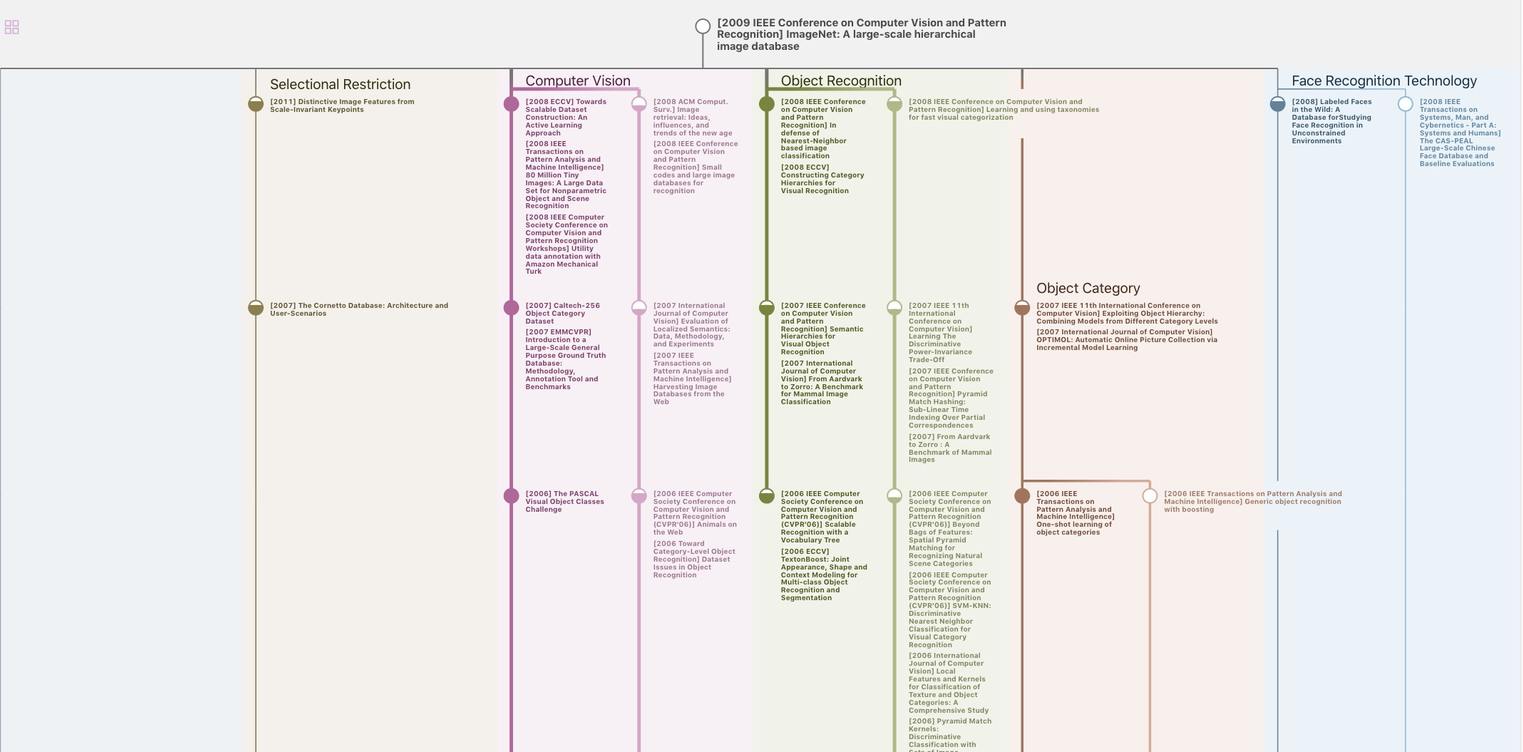
生成溯源树,研究论文发展脉络
Chat Paper
正在生成论文摘要