Adversarial Approaches to Tackle Imbalanced Data in Machine Learning
SUSTAINABILITY(2023)
摘要
Real-world applications often involve imbalanced datasets, which have different distributions of examples across various classes. When building a system that requires a high accuracy, the performance of the classifiers is crucial. However, imbalanced datasets can lead to a poor classification performance and conventional techniques, such as synthetic minority oversampling technique. As a result, this study proposed a balance between the datasets using adversarial learning methods such as generative adversarial networks. The model evaluated the effect of data augmentation on both the balanced and imbalanced datasets. The study evaluated the classification performance on three different datasets and applied data augmentation techniques to generate the synthetic data for the minority class. Before the augmentation, a decision tree was applied to identify the classification accuracy of all three datasets. The obtained classification accuracies were 79.9%, 94.1%, and 72.6%. A decision tree was used to evaluate the performance of the data augmentation, and the results showed that the proposed model achieved an accuracy of 82.7%, 95.7%, and 76% on a highly imbalanced dataset. This study demonstrates the potential of using data augmentation to improve the classification performance in imbalanced datasets.
更多查看译文
关键词
computer vision, machine learning, deep learning, imbalanced dataset
AI 理解论文
溯源树
样例
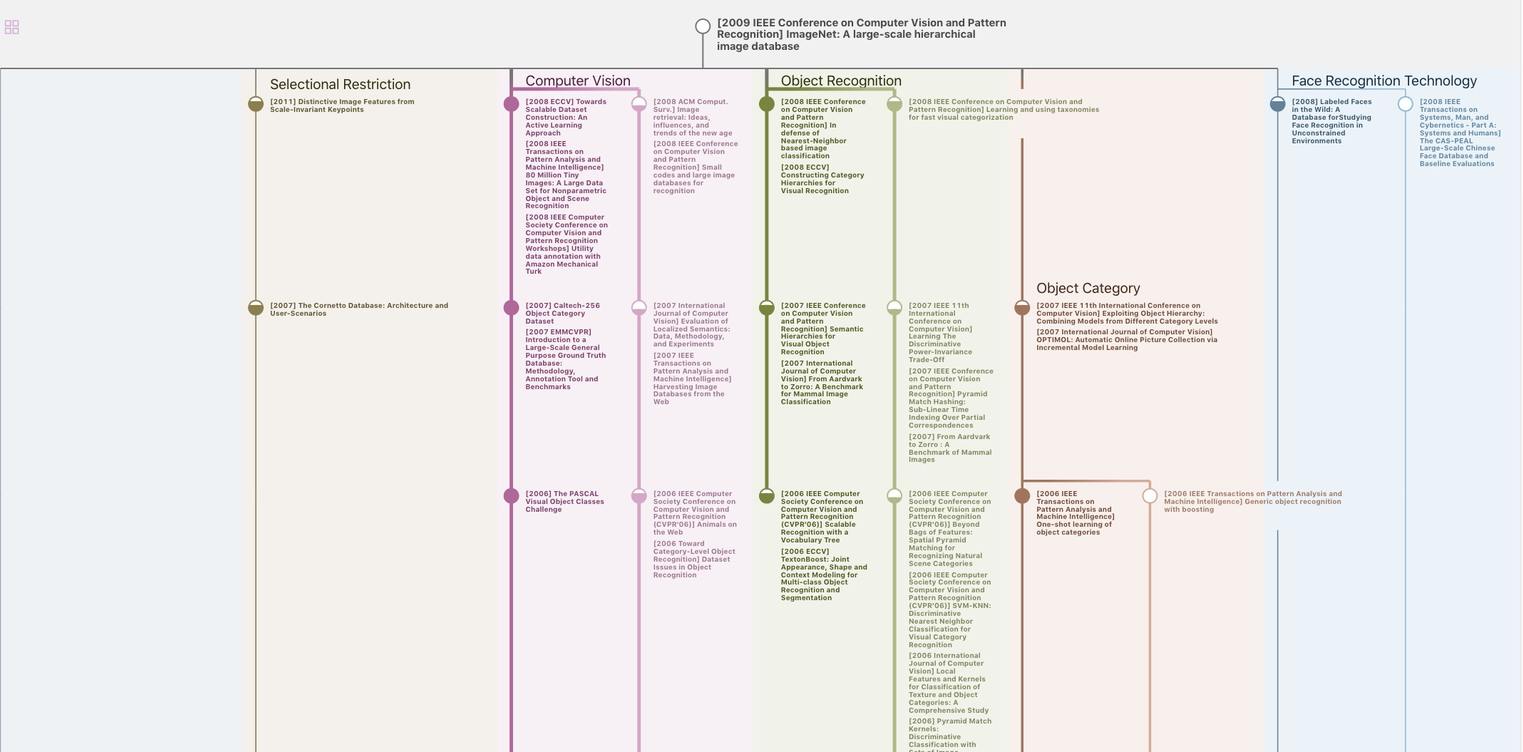
生成溯源树,研究论文发展脉络
Chat Paper
正在生成论文摘要