Clinical Validation of an Artificial Intelligence Model for Detecting Distal Radius, Ulnar Styloid, and Scaphoid Fractures on Conventional Wrist Radiographs
DIAGNOSTICS(2023)
摘要
This study aimed to assess the feasibility and performance of an artificial intelligence (AI) model for detecting three common wrist fractures: distal radius, ulnar styloid process, and scaphoid. The AI model was trained with a dataset of 4432 images containing both fractured and non-fractured wrist images. In total, 593 subjects were included in the clinical test. Two human experts independently diagnosed and labeled the fracture sites using bounding boxes to build the ground truth. Two novice radiologists also performed the same task, both with and without model assistance. The sensitivity, specificity, accuracy, and area under the curve (AUC) were calculated for each wrist location. The AUC for detecting distal radius, ulnar styloid, and scaphoid fractures per wrist were 0.903 (95% C.I. 0.887-0.918), 0.925 (95% C.I. 0.911-0.939), and 0.808 (95% C.I. 0.748-0.967), respectively. When assisted by the AI model, the scaphoid fracture AUC of the two novice radiologists significantly increased from 0.75 (95% C.I. 0.66-0.83) to 0.85 (95% C.I. 0.77-0.93) and from 0.71 (95% C.I. 0.62-0.80) to 0.80 (95% C.I. 0.71-0.88), respectively. Overall, the developed AI model was found to be reliable for detecting wrist fractures, particularly for scaphoid fractures, which are commonly missed.
更多查看译文
关键词
artificial intelligence,convolutional neural network,distal radius fracture,ulnar styloid fracture,scaphoid fracture
AI 理解论文
溯源树
样例
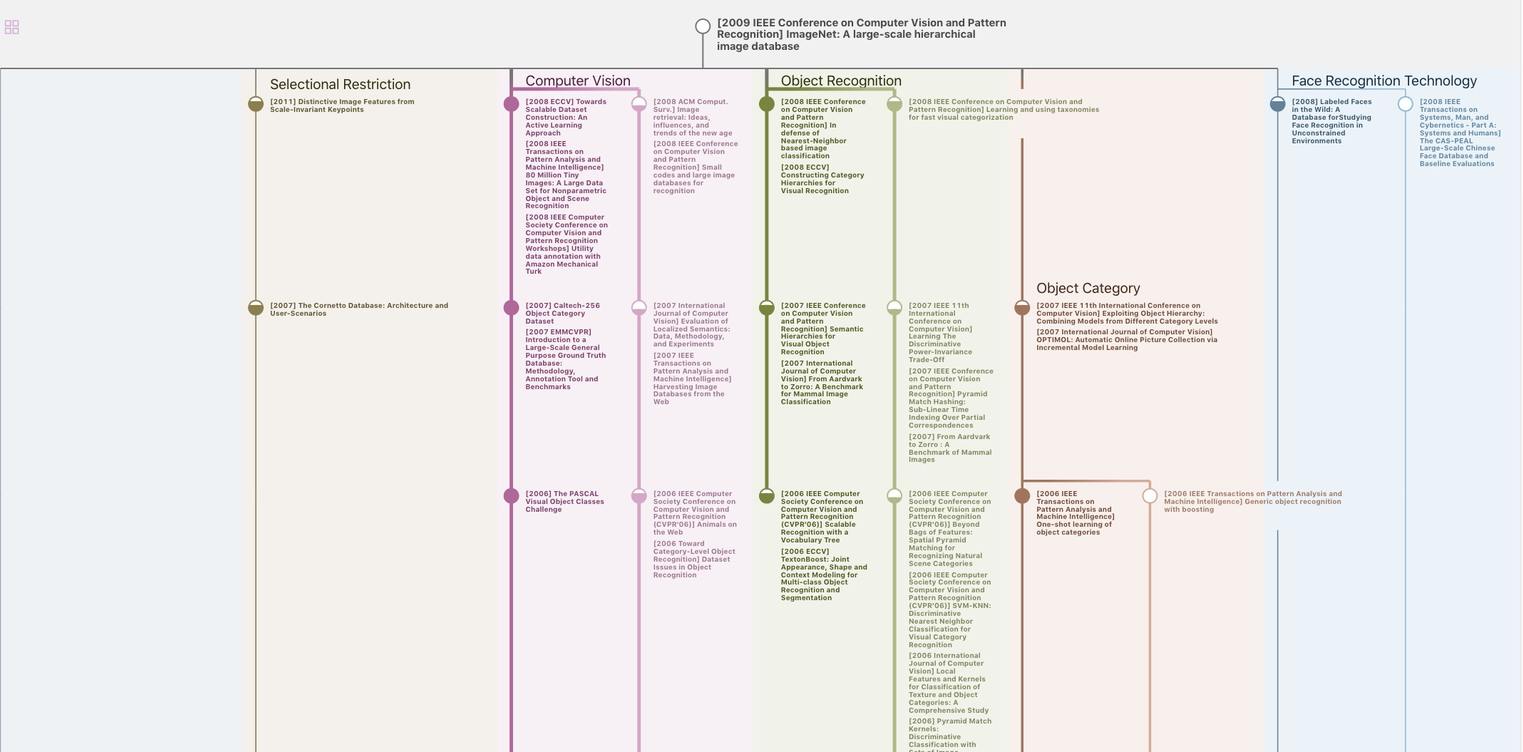
生成溯源树,研究论文发展脉络
Chat Paper
正在生成论文摘要