Deep Reinforcement Learning-Based Energy Minimization Task Offloading and Resource Allocation for Air Ground Integrated Heterogeneous Networks
IEEE SYSTEMS JOURNAL(2023)
Abstract
With the popularization of Internet of Things, a number of emerging applications all require both efficient communication and computing service, which poses enormous challenges to the computing ability and battery capacity of terminal equipment. Moreover, the ground-based 5G system cannot provide seamless service especially for hotspot and remote area. In order to deal with the mentioned issues, we first propose an air ground integrated heterogeneous networks model, which consists of multiple UAVs and GBSs equipped with edge servers. Then, by jointly taking into account terminal power control, computing resource allocation, and task offloading decision, an energy-minimization issue is formulated, which, however, is a mixed integer nonlinear programming problem due to the tight coupling between optimization variables. Therefore, we decompose it into two subproblems and design a deep actor-critic-based online offloading algorithm to solve the first offloading decision-making issue facing dimensionality curse. For the second power control and computing resource allocation subproblem, a difference-of-convex-based solution is presented. The proposed approach can achieve superior performance in terms of terminal energy consumption and convergence speed with lower complexity compared with other benchmark methods. Specifically, it outperforms DDQN, AC&Greedy, and UCB algorithm by 7.62%, 17.32%, and 23.14%, respectively.
MoreTranslated text
Key words
Task analysis, Resource management, Computational modeling, Servers, Power control, Edge computing, Optimization, Air ground integrated heterogeneous networks (AGIHN), deep reinforcement learning (RL), energy minimization, resource allocation, task offloading
AI Read Science
Must-Reading Tree
Example
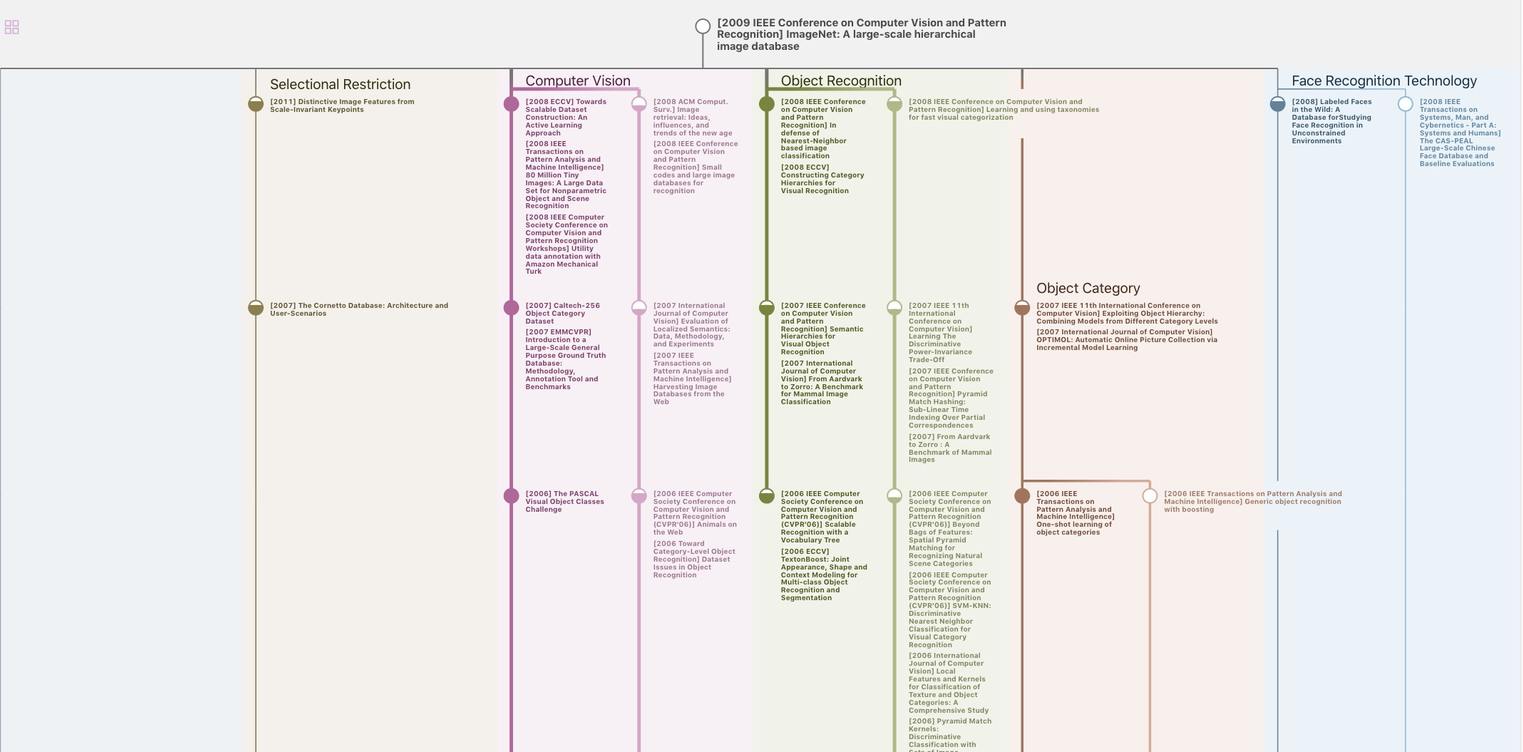
Generate MRT to find the research sequence of this paper
Chat Paper
Summary is being generated by the instructions you defined