EdgeBOL: A Bayesian Learning Approach for the Joint Orchestration of vRANs and Mobile Edge AI
IEEE-ACM TRANSACTIONS ON NETWORKING(2023)
摘要
Future mobile networks need to support intelligent services which collect and process data streams at the network edge, so as to offer real-time and accurate inferences to users. However, the widespread deployment of these services is hindered by the unprecedented energy cost they induce to the network, and by the difficulties in optimizing their end-to-end operation. To address these challenges, we propose a Bayesian learning framework for jointly configuring the service and the Radio Access Network (RAN), aiming to minimize the total energy consumption while respecting accuracy and latency service requirements. Using a fully-fledged prototype with a software-defined base station (vBS) and a GPU-enabled edge server, we profile a typical video analytics service and identify new performance trade-offs and optimization opportunities. Accordingly, we tailor the proposed learning framework to account for the (possibly varying) network conditions, user needs, and service metrics, and apply it to a range of experiments with real traces. Our findings suggest that this approach effectively adapts to different hardware platforms and service requirements, and outperforms state-of-the-art benchmarks based on neural networks.
更多查看译文
关键词
joint orchestration,bayesian learning approach,vrans,ai
AI 理解论文
溯源树
样例
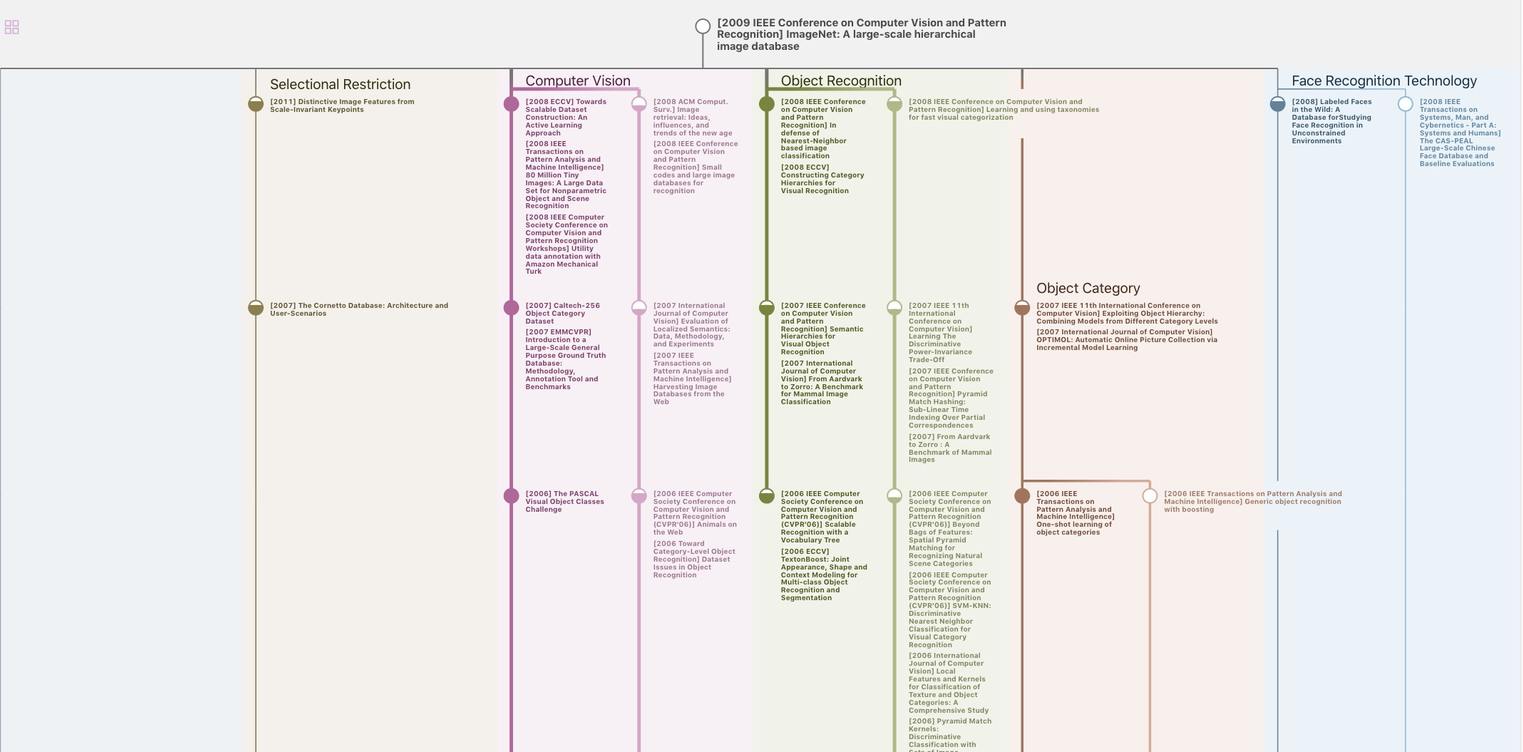
生成溯源树,研究论文发展脉络
Chat Paper
正在生成论文摘要