Cerebrovascular segmentation from mesoscopic optical images using Swin Transformer
JOURNAL OF INNOVATIVE OPTICAL HEALTH SCIENCES(2023)
摘要
Vascular segmentation is a crucial task in biomedical image processing, which is significant for analyzing and modeling vascular networks under physiological and pathological states. With advances in fluorescent labeling and mesoscopic optical techniques, it has become possible to map the whole-mouse-brain vascular networks at capillary resolution. However, segmenting vessels from mesoscopic optical images is a challenging task. The problems, such as vascular signal discontinuities, vessel lumens, and background fluorescence signals in mesoscopic optical images, belong to global semantic information during vascular segmentation. Traditional vascular segmentation methods based on convolutional neural networks (CNNs) have been limited by their insufficient receptive fields, making it challenging to capture global semantic information of vessels and resulting in inaccurate segmentation results. Here, we propose SegVesseler, a vascular segmentation method based on Swin Transformer. SegVesseler adopts 3D Swin Transformer blocks to extract global contextual information in 3D images. This approach is able to maintain the connectivity and topology of blood vessels during segmentation. We evaluated the performance of our method on mouse cerebrovascular datasets generated from three different labeling and imaging modalities. The experimental results demonstrate that the segmentation effect of our method is significantly better than traditional CNNs and achieves state-of-the-art performance.
更多查看译文
关键词
Vascular segmentation, Swin Transformer, mesoscopic optical imaging, fMOST
AI 理解论文
溯源树
样例
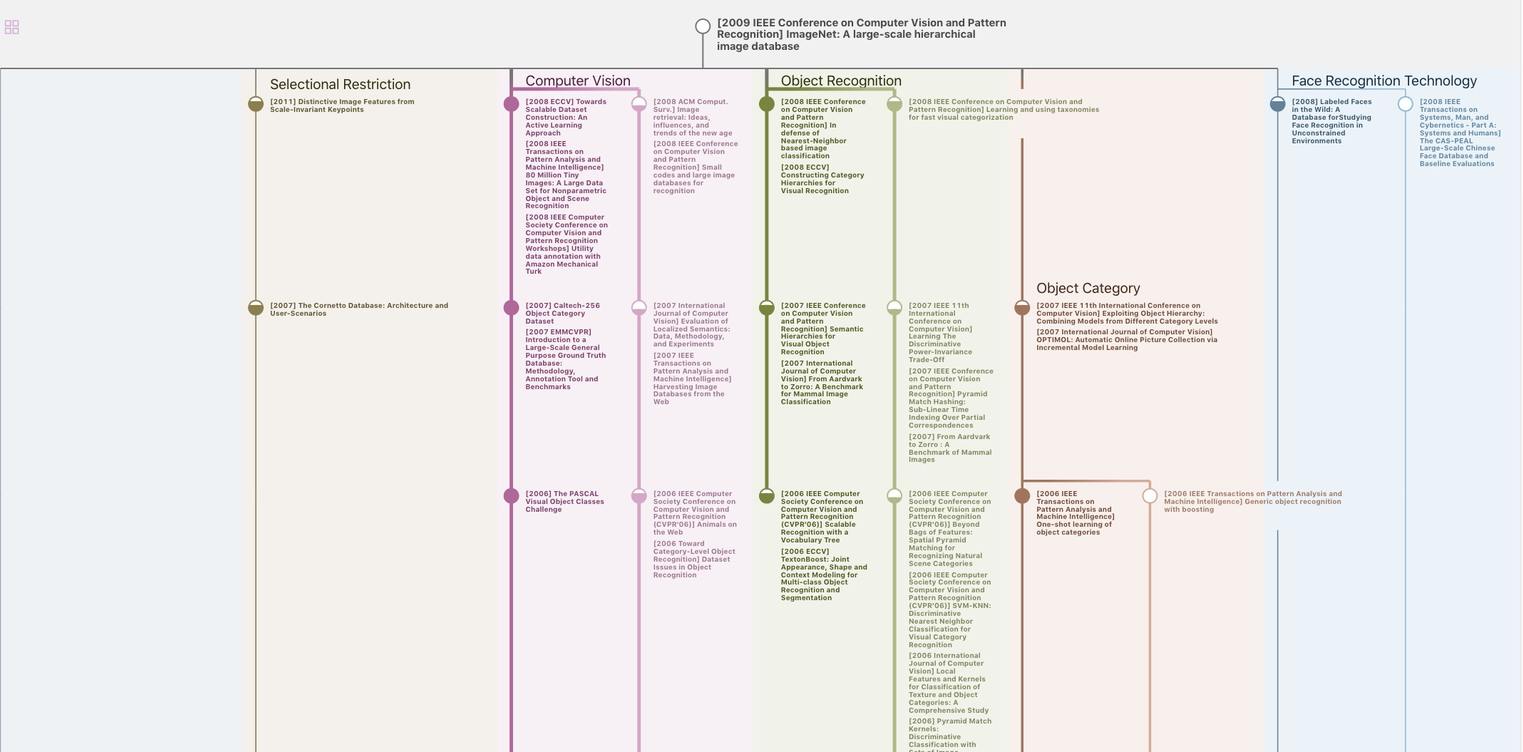
生成溯源树,研究论文发展脉络
Chat Paper
正在生成论文摘要