A Flow Feedback Traffic Prediction Based on Visual Quantified Features
IEEE TRANSACTIONS ON INTELLIGENT TRANSPORTATION SYSTEMS(2023)
摘要
Traffic flow prediction methods commonly rely on historical traffic data, such as traffic volume and speed, but may not be suitable for high-capacity expressways or during peak traffic hours. Furthermore, downstream flow can have significant impacts on traffic flow. To address these challenges, our study proposes a novel traffic flow prediction model, V-STF, which integrates visual methods to quantify macroscopic traffic flow indicators, as well as density features in temporal and flow feedback in spatio features. The contribution of our proposed model lies in its ability to improve prediction accuracy during non-periodic peak hours, by taking into account the impact of congested road conditions on traffic flow. Our experiments using the STREETS dataset demonstrate that V-STF outperforms state-of-the-art methods, especially in predicting sudden changes in traffic flow, resulting in more accurate predictions.
更多查看译文
关键词
Roads,Predictive models,Data models,Feature extraction,Visualization,Correlation,Computational modeling,Visual quantization,flow prediction,traffic flow density,flow feedback,spatio-temporal features
AI 理解论文
溯源树
样例
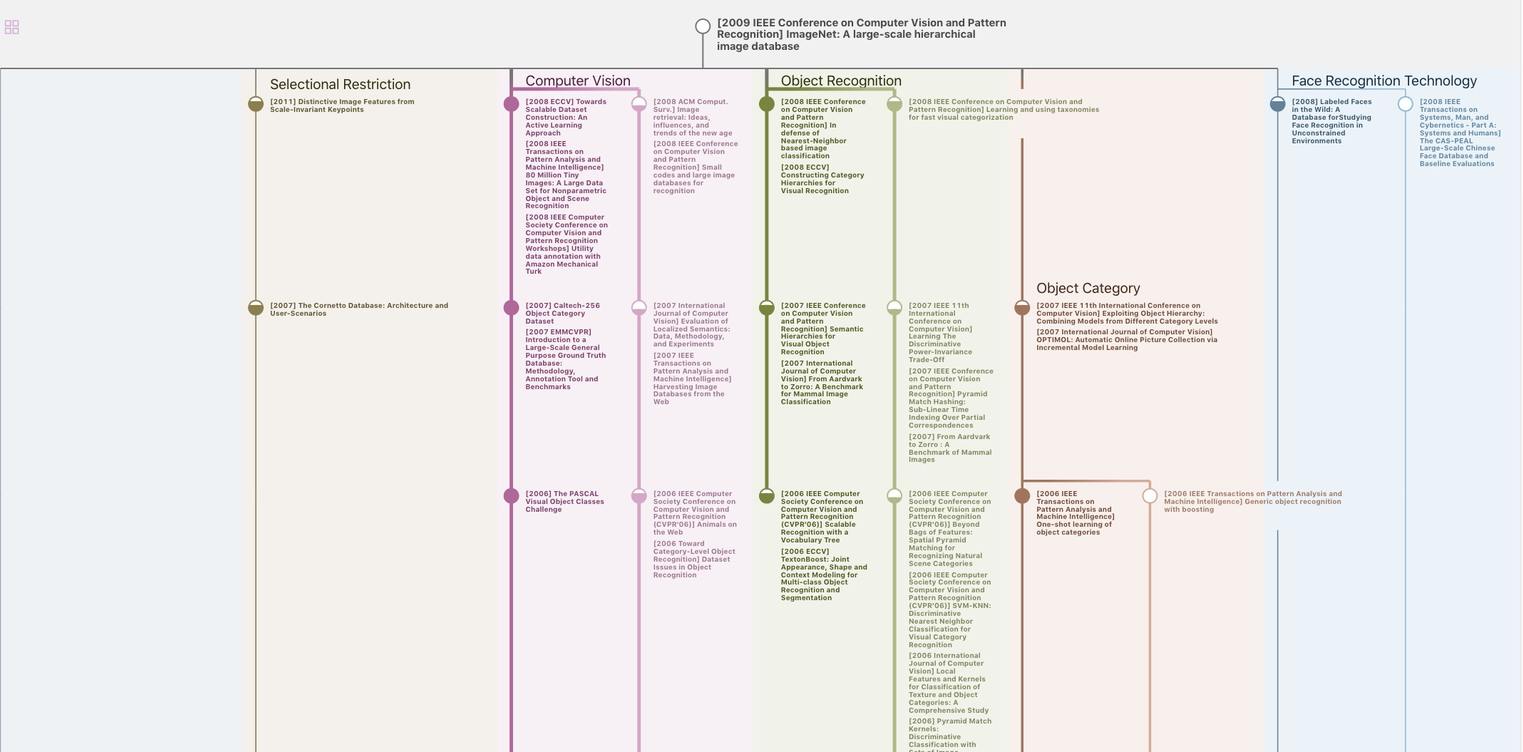
生成溯源树,研究论文发展脉络
Chat Paper
正在生成论文摘要