Self-organizing maps on “what-where” codes towards fully unsupervised classification
BIOLOGICAL CYBERNETICS(2023)
摘要
Interest in unsupervised learning architectures has been rising. Besides being biologically unnatural, it is costly to depend on large labeled data sets to get a well-performing classification system. Therefore, both the deep learning community and the more biologically-inspired models community have focused on proposing unsupervised techniques that can produce adequate hidden representations which can then be fed to a simpler supervised classifier. Despite great success with this approach, an ultimate dependence on a supervised model remains, which forces the number of classes to be known beforehand, and makes the system depend on labels to extract concepts. To overcome this limitation, recent work has been proposed that shows how a self-organizing map (SOM) can be used as a completely unsupervised classifier. However, to achieve success it required deep learning techniques to generate high quality embeddings. The purpose of this work is to show that we can use our previously proposed What-Where encoder in tandem with the SOM to get an end-to-end unsupervised system that is Hebbian. Such system, requires no labels to train nor does it require knowledge of which classes exist beforehand. It can be trained online and adapt to new classes that may emerge. As in the original work, we use the MNIST data set to run an experimental analysis and verify that the system achieves similar accuracies to the best ones reported thus far. Furthermore, we extend the analysis to the more difficult Fashion-MNIST problem and conclude that the system still performs.
更多查看译文
关键词
Unsupervised classification,Self-organizing maps,What-Where codes,Biologically-inspired models,Visual pattern recognition
AI 理解论文
溯源树
样例
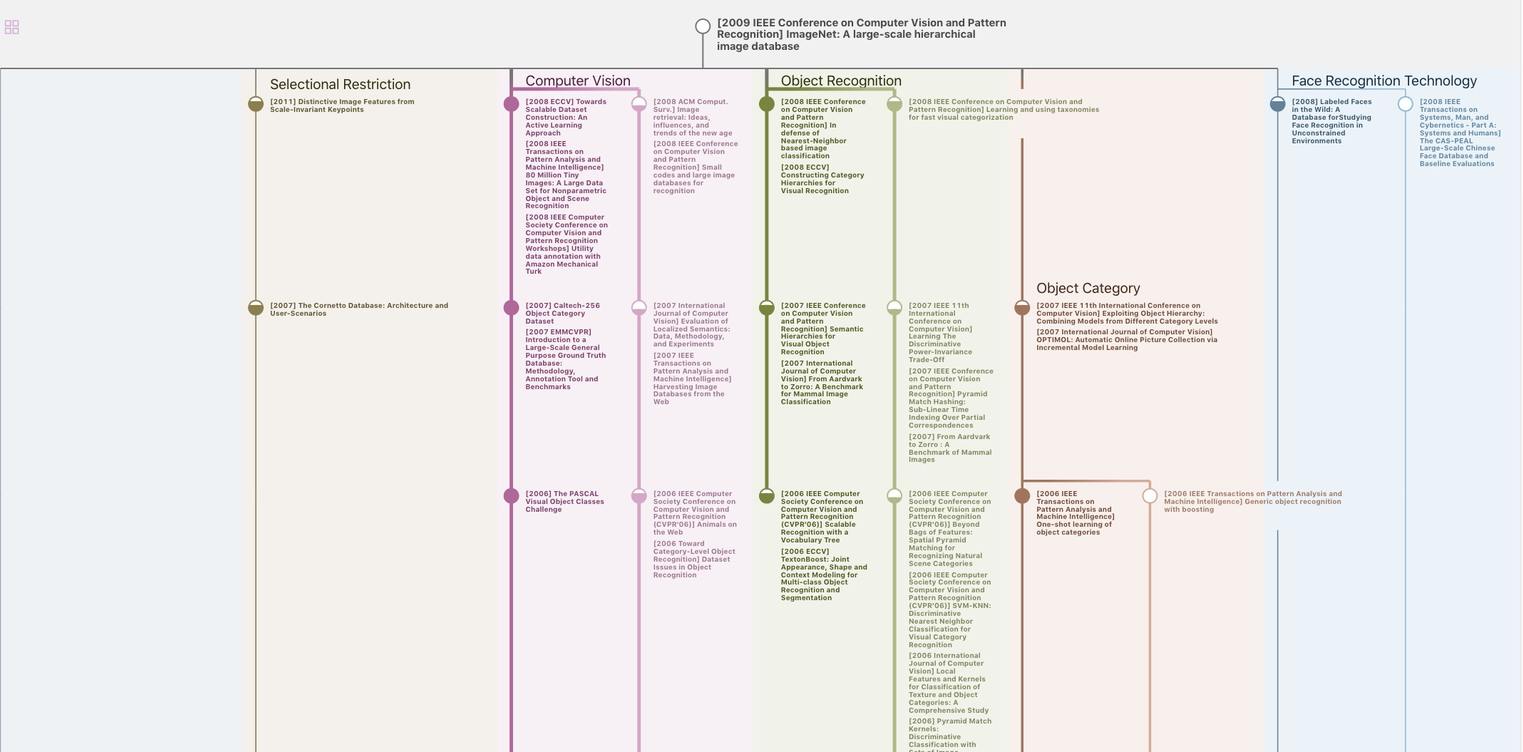
生成溯源树,研究论文发展脉络
Chat Paper
正在生成论文摘要