Artificial Neural Network (ANN)-Based Determination of Fractional Contributions from Mixed Fluorophores using Fluorescence Lifetime Measurements
JOURNAL OF FLUORESCENCE(2024)
摘要
Here we present an artificial neural network (ANN)-approach to determine the fractional contributions P-i from fluorophores to a multi-exponential fluorescence decay in time-resolved lifetime measurements. Conventionally, P-i are determined by extracting two parameters (amplitude and lifetime) for each underlying mono-exponential decay using non-linear fitting. However, in this case parameter estimation is highly sensitive to initial guesses and weighting. In contrast, the ANN-based approach robustly gives the P-i without knowledge of amplitudes and lifetimes. By experimental measurements and Monte-Carlo simulations, we comprehensively show that accuracy and precision of P-i determination with ANNs and hence the number of distinguishable fluorophores depend on the fluorescence lifetimes' differences. For mixtures of up to five fluorophores, we determined the minimum uniform spacing Delta tau(min) between lifetimes to obtain fractional contributions with a standard deviation of 5%. In example, five lifetimes can be distinguished with a respective minimum uniform spacing of approx. 10 ns even when the fluorophores' emission spectra are overlapping. This study underlines the enormous potential of ANN-based analysis for multi-fluorophore applications in fluorescence lifetime measurements.
更多查看译文
关键词
Fractional contributions,Fluorescence lifetime,Artificial neural networks,Monte Carlo
AI 理解论文
溯源树
样例
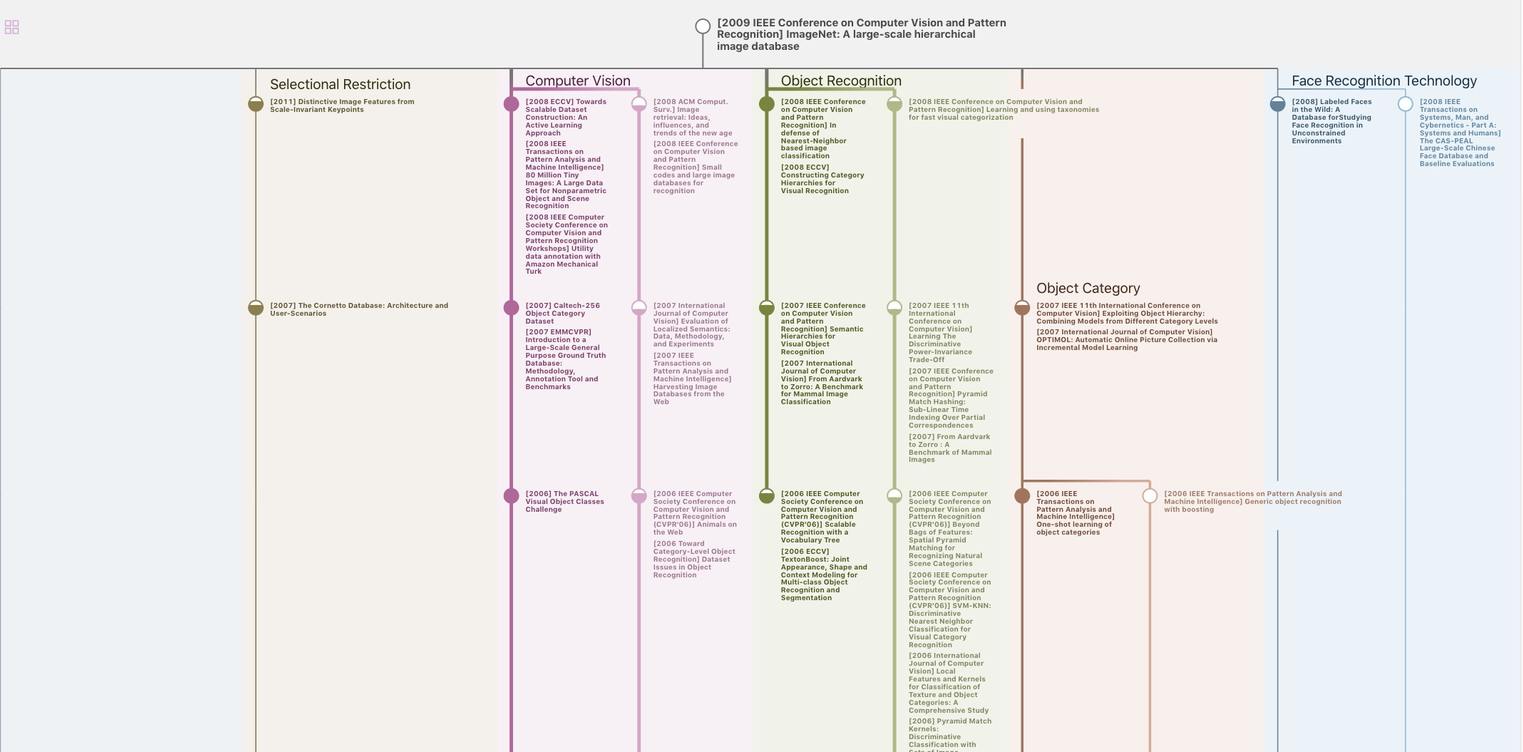
生成溯源树,研究论文发展脉络
Chat Paper
正在生成论文摘要