IonML: A physically inspired machine learning platform to directed design superionic conductors
ENERGY STORAGE MATERIALS(2023)
摘要
Finding superionic conductors (SICs) has always been an arduous task in material science, not to mention large accessible SIC databases. How to obtain SICs by directed design in the high-dimensional complex chemical space is also an unexplored challenge. To reduce experimental and computational effort associated with directed SIC design and to explore the chemical space encoded by structure, component and site simultaneously, we introduce IonML, a physically inspired machine learning (ML) platform to efficiently directed design SICs. In the case of Li+ conductors, it features an ensemble learning model that identifies SICs in 0.8 s with a high precision of 90.4% and reveals the effects of chemical factors on Li+ migration; it integrates an active learning with physically inspired model that enables directed SIC design within only 2 min. IonML possesses a database of over 19,000 SICs in which we rapidly identify 61 new stable SICs. Importantly, for the remaining non-SICs with low ionic conduc-tivities, IonML directed designs 65 new SICs by regulating the chemical space to "turn waste into treasure". IonML highlights the utility of combining fingerprint, prediction, screening, and directed design with explainable ML, and can be extended to all types of SICs (Na+, Mg2+, Al3+) or other purpose-driven material design systems.
更多查看译文
关键词
Nanostructured energy materials, Solid state electrolyte, Machine learning
AI 理解论文
溯源树
样例
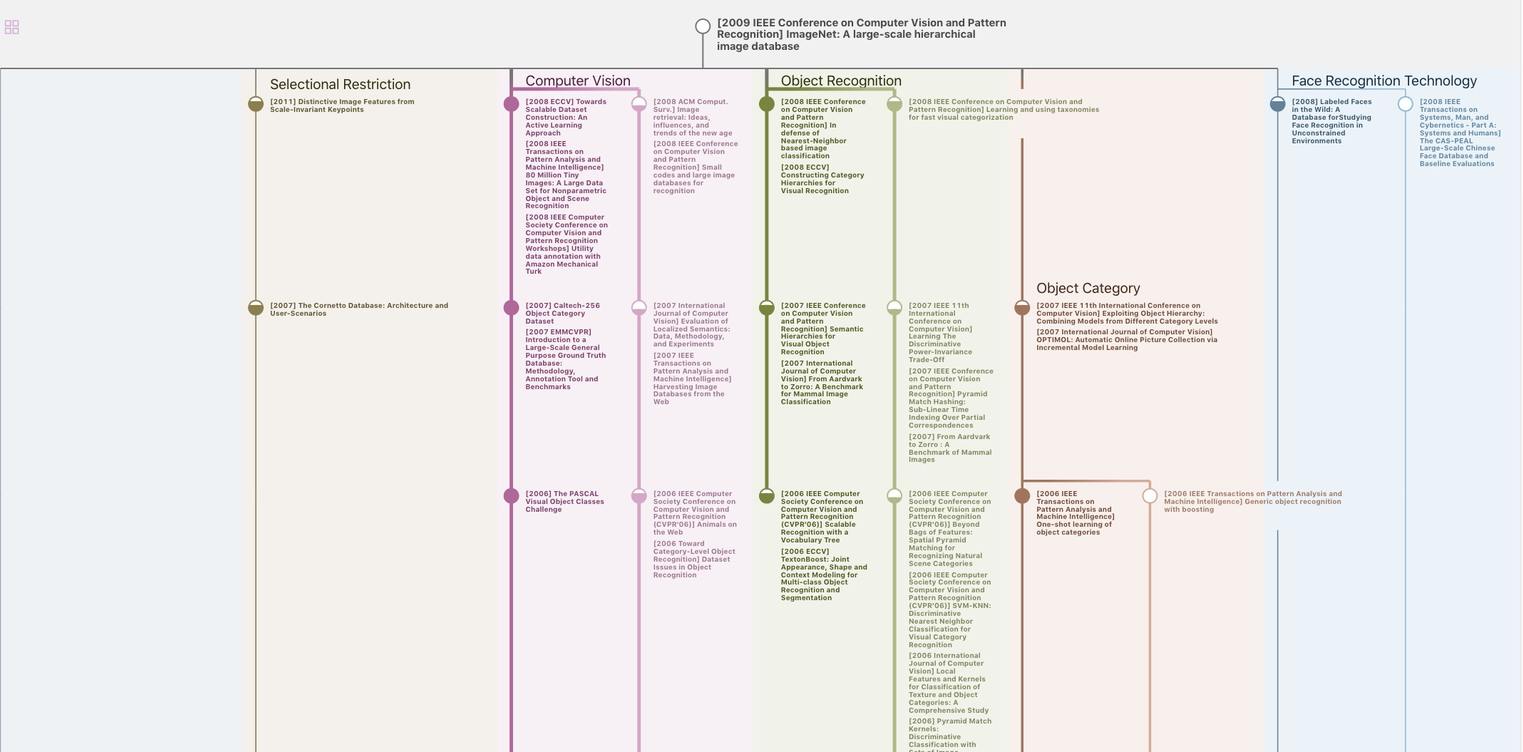
生成溯源树,研究论文发展脉络
Chat Paper
正在生成论文摘要