A Method for Complex Question-Answering over Knowledge Graph
APPLIED SCIENCES-BASEL(2023)
摘要
Knowledge Graph Question-Answering (KGQA) has gained popularity as an effective approach for information retrieval systems. However, answering complex questions involving multiple topic entities and multi-hop relations presents a significant challenge for model training. Moreover, existing KGQA models face difficulties in extracting constraint information from complex questions, leading to reduced accuracy. To overcome these challenges, we propose a three-part pipelined framework comprising question decomposition, constraint extraction, and question reasoning. Our approach employs a novel question decomposition model that uses dual encoders and attention mechanisms to enhance question representation. We define temporal, spatial, and numerical constraint types and propose a constraint extraction model to mitigate the impact of constraint interference on downstream question reasoning. The question reasoning model uses beam search to reduce computational effort and enhance exploration, facilitating the identification of the optimal path. Experimental results on the ComplexWebQuestions dataset demonstrate the efficacy of our proposed model, achieving an F1 score of 72.0% and highlighting the effectiveness of our approach in decomposing complex questions into simple subsets and improving the accuracy of question reasoning.
更多查看译文
关键词
knowledge graph, question-answering over knowledge graph, complex question, deep neural networks
AI 理解论文
溯源树
样例
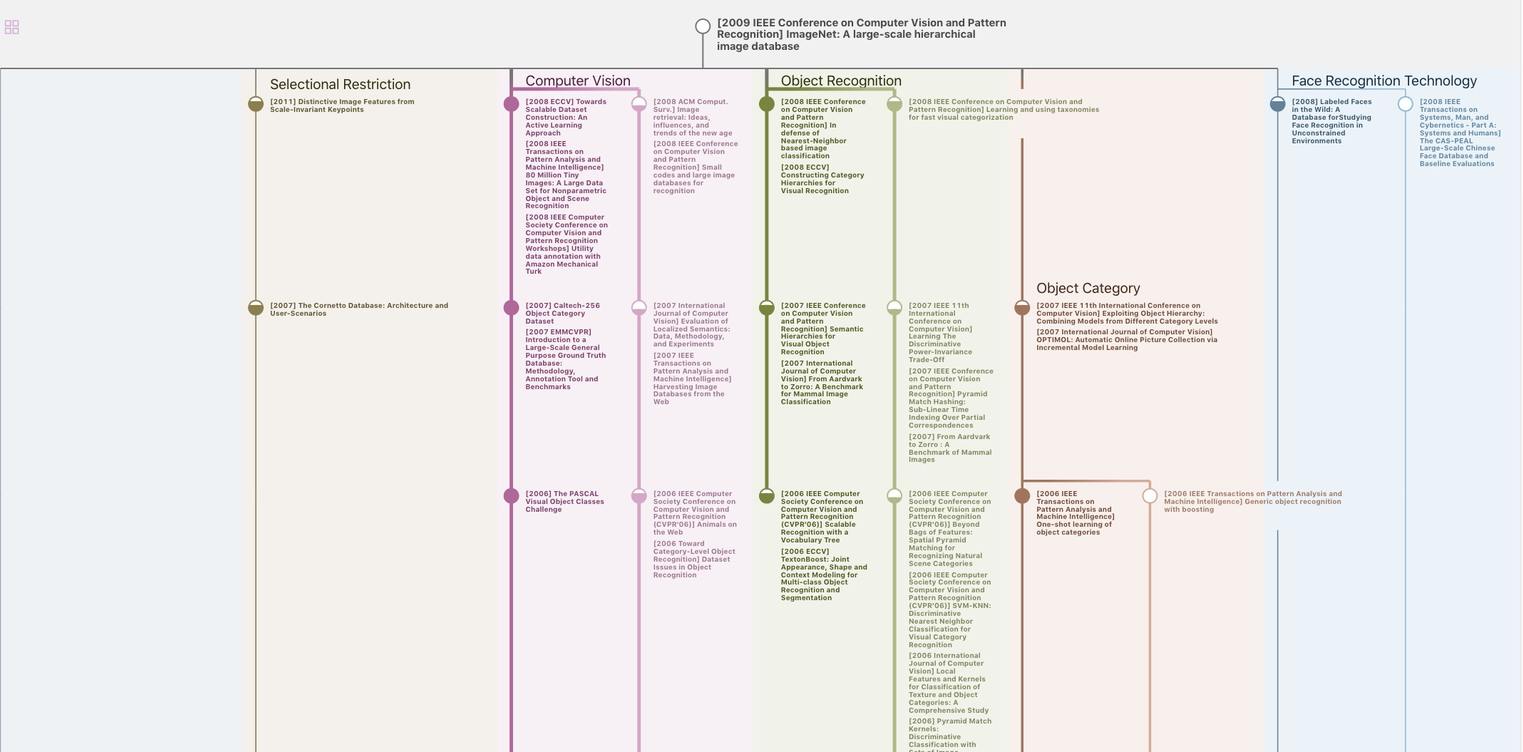
生成溯源树,研究论文发展脉络
Chat Paper
正在生成论文摘要