MSCNN-LSTM Model for Predicting Return Loss of the UHF Antenna in HF-UHF RFID Tag Antenna
CMC-COMPUTERS MATERIALS & CONTINUA(2023)
摘要
High-frequency (HF) and ultrahigh-frequency (UHF) dual-band radio frequency identification (RFID) tags with both near-field and far - field communication can meet different application scenarios. However, it is time-consuming to calculate the return loss of a UHF antenna in a dual - band tag antenna using electromagnetic (EM) simulators. To overcome this, the present work proposes a model of a multi-scale convolutional neural network stacked with long and short-term memory (MSCNN-LSTM) for predicting the return loss of UHF antennas instead of EM simulators. In the proposed MSCNN-LSTM, the MSCNN has three branches, which include three convolution layers with different kernel sizes and numbers. Therefore, MSCNN can extract fine-grain localized information of the antenna and overall features. The LSTM can effectively learn the EM characteristics of different structures of the antenna to improve the prediction accuracy of the model. Experimental results show that the mean absolute error (0.0073), mean square error (0.00032), and root mean square error (0.01814) of the MSCNN- LSTM are better than those of other prediction methods. In predicting the return loss of 100 UHF antennas, compared with the simulation time of 4800 s for High Frequency Structure Simulator (HFSS), MSCNN-LSTM takes only 0.927519 s under the premise of ensuring prediction accuracy, significantly reducing the calculation time, which provides a basis for the rapid design of HF-UHF RFID tag antenna. Then MSCNN-LSTM is used to determine the dimensions of the UHF antenna quickly. The return loss of the designed dual - band RFID tag antenna is -58.76 and -22.63 dB at 13.56 and 915 MHz, respectively, achieving the desired goal.
更多查看译文
关键词
uhf antenna,return loss,mscnn-lstm,hf-uhf
AI 理解论文
溯源树
样例
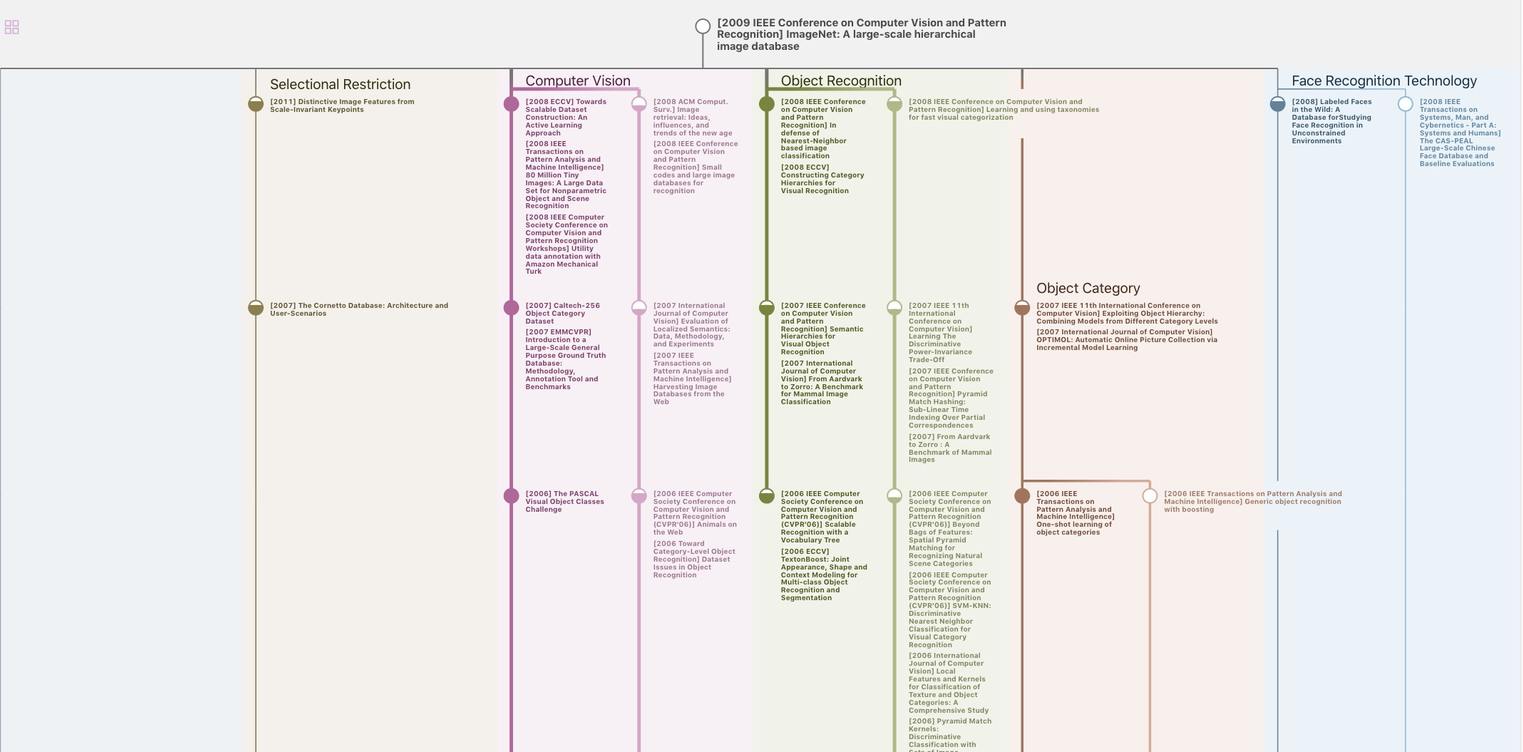
生成溯源树,研究论文发展脉络
Chat Paper
正在生成论文摘要