MCIBI plus plus : Soft Mining Contextual Information Beyond Image for Semantic Segmentation
IEEE TRANSACTIONS ON PATTERN ANALYSIS AND MACHINE INTELLIGENCE(2023)
摘要
Co-occurrent visual pattern makes context aggregation become an essential paradigm for semantic segmentation. The existing studies focus on modeling the contexts within image while neglecting the valuable semantics of the corresponding category beyond image. To this end, we propose a novel soft mining contextual information beyond image paradigm named MCIBI++ to further boost the pixel-level representations. Specifically, we first set up a dynamically updated memory module to store the dataset-level distribution information of various categories and then leverage the information to yield the dataset-level category representations during network forward. After that, we generate a class probability distribution for each pixel representation and conduct the dataset-level context aggregation with the class probability distribution as weights. Finally, the original pixel representations are augmented with the aggregated dataset-level and the conventional image-level contextual information. Moreover, in the inference phase, we additionally design a coarse-to-fine iterative inference strategy to further boost the segmentation results. MCIBI++ can be effortlessly incorporated into the existing segmentation frameworks and bring consistent performance improvements. Also, MCIBI++ can be extended into the video semantic segmentation framework with considerable improvements over the baseline. Equipped with MCIBI++, we achieved the state-of-the-art performance on seven challenging image or video semantic segmentation benchmarks.
更多查看译文
关键词
Image segmentation, Semantics, Memory modules, Task analysis, Iterative methods, Benchmark testing, Transformers, Semantic segmentation, context aggregation, dataset statistics, memory module, iterative testing, video semantic segmentation
AI 理解论文
溯源树
样例
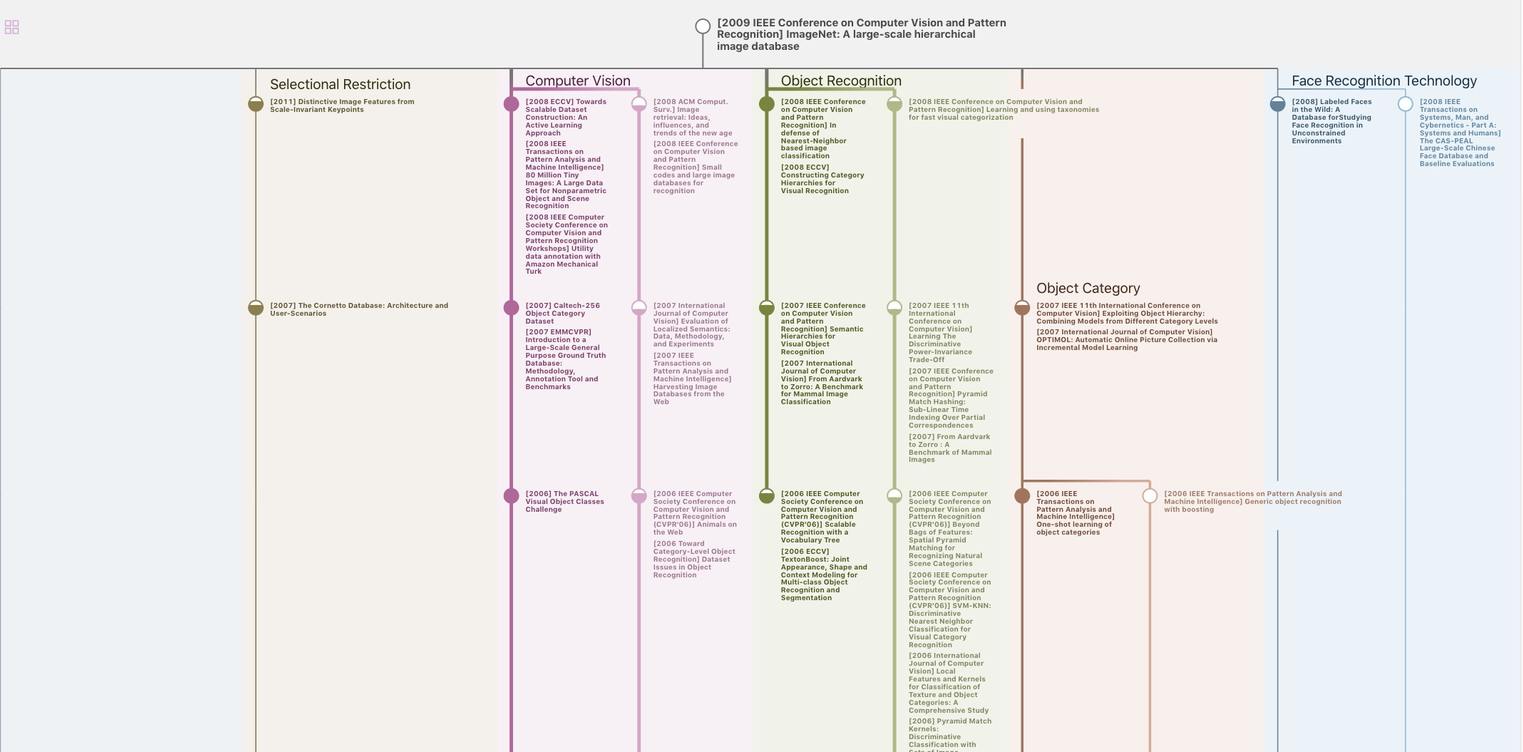
生成溯源树,研究论文发展脉络
Chat Paper
正在生成论文摘要