FPGNN-ATPG: An Efficient Fault Parallel Automatic Test Pattern Generator
GLSVLSI '23: Proceedings of the Great Lakes Symposium on VLSI 2023(2023)
摘要
The advanced multi-core technology enables parallel computing to speed up Automatic Test Pattern Generation (ATPG). The main challenge is to solve an increasing number of hard-to-solve faults effectively. In this paper, we develop an efficient parallel computing system for the ATPG program, i.e., FPGNN-ATPG, which is consisted of two parts: graph-neural-networks-based (GNN-based) fault classification and fault-driven deterministic test pattern generator (DTPG). The end-to-end GNN-based classifier can predict fault types with superior accuracy compared with classical machine learning methods. And the fault-driven DTPG can solve different types of faults in parallel without runtime overhead. According to the experimental results on an 8-core machine, our FPGNN-ATPG framework obtains an average of 7.56X speedup while reducing 14.13% pattern count ratio with full 100% fault coverage for 10 industrial instances.
更多查看译文
关键词
ATPG, graph learning, parallel technology
AI 理解论文
溯源树
样例
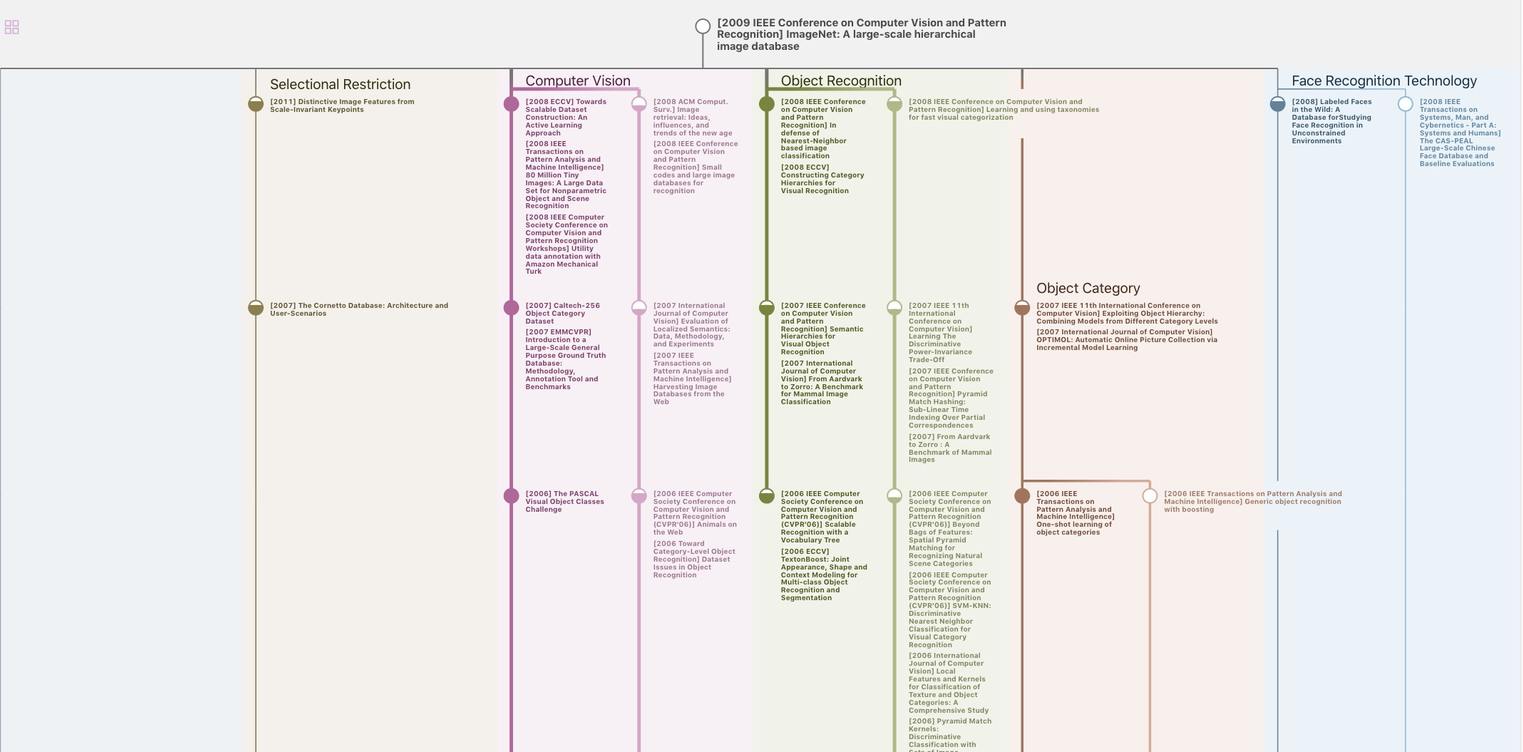
生成溯源树,研究论文发展脉络
Chat Paper
正在生成论文摘要