Local Boosting for Weakly-Supervised Learning
PROCEEDINGS OF THE 29TH ACM SIGKDD CONFERENCE ON KNOWLEDGE DISCOVERY AND DATA MINING, KDD 2023(2023)
摘要
Boosting is a commonly used technique to enhance the performance of a set of base models by combining them into a strong ensemble model. Though widely adopted, boosting is typically used in supervised learning where the data is labeled accurately. However, in weakly supervised learning, where most of the data is labeled through weak and noisy sources, it remains nontrivial to design effective boosting approaches. In this work, we show that the standard implementation of the convex combination of base learners can hardly work due to the presence of noisy labels. Instead, we propose $\textit{LocalBoost}$, a novel framework for weakly-supervised boosting. LocalBoost iteratively boosts the ensemble model from two dimensions, i.e., intra-source and inter-source. The intra-source boosting introduces locality to the base learners and enables each base learner to focus on a particular feature regime by training new base learners on granularity-varying error regions. For the inter-source boosting, we leverage a conditional function to indicate the weak source where the sample is more likely to appear. To account for the weak labels, we further design an estimate-then-modify approach to compute the model weights. Experiments on seven datasets show that our method significantly outperforms vanilla boosting methods and other weakly-supervised methods.
更多查看译文
关键词
Boosting Methods,Weak Supervision,Classification
AI 理解论文
溯源树
样例
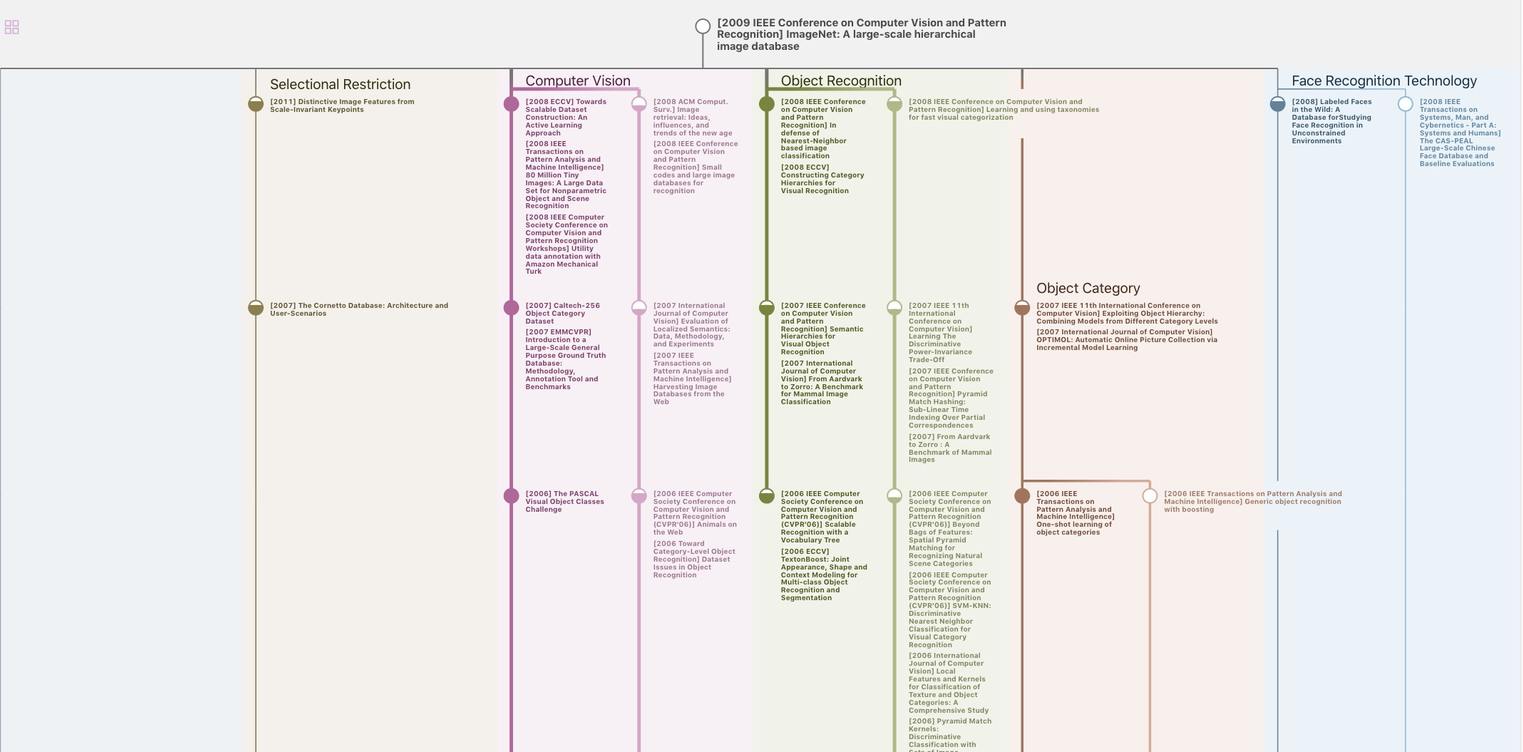
生成溯源树,研究论文发展脉络
Chat Paper
正在生成论文摘要