Calibrated Stackelberg Games: Learning Optimal Commitments Against Calibrated Agents
NeurIPS(2023)
摘要
In this paper, we introduce a generalization of the standard Stackelberg Games (SGs) framework: Calibrated Stackelberg Games (CSGs). In CSGs, a principal repeatedly interacts with an agent who (contrary to standard SGs) does not have direct access to the principal's action but instead best-responds to calibrated forecasts about it. CSG is a powerful modeling tool that goes beyond assuming that agents use ad hoc and highly specified algorithms for interacting in strategic settings and thus more robustly addresses real-life applications that SGs were originally intended to capture. Along with CSGs, we also introduce a stronger notion of calibration, termed adaptive calibration, that provides fine-grained any-time calibration guarantees against adversarial sequences. We give a general approach for obtaining adaptive calibration algorithms and specialize them for finite CSGs. In our main technical result, we show that in CSGs, the principal can achieve utility that converges to the optimum Stackelberg value of the game both in finite and continuous settings, and that no higher utility is achievable. Two prominent and immediate applications of our results are the settings of learning in Stackelberg Security Games and strategic classification, both against calibrated agents.
更多查看译文
关键词
optimal commitments,agents,games,learning
AI 理解论文
溯源树
样例
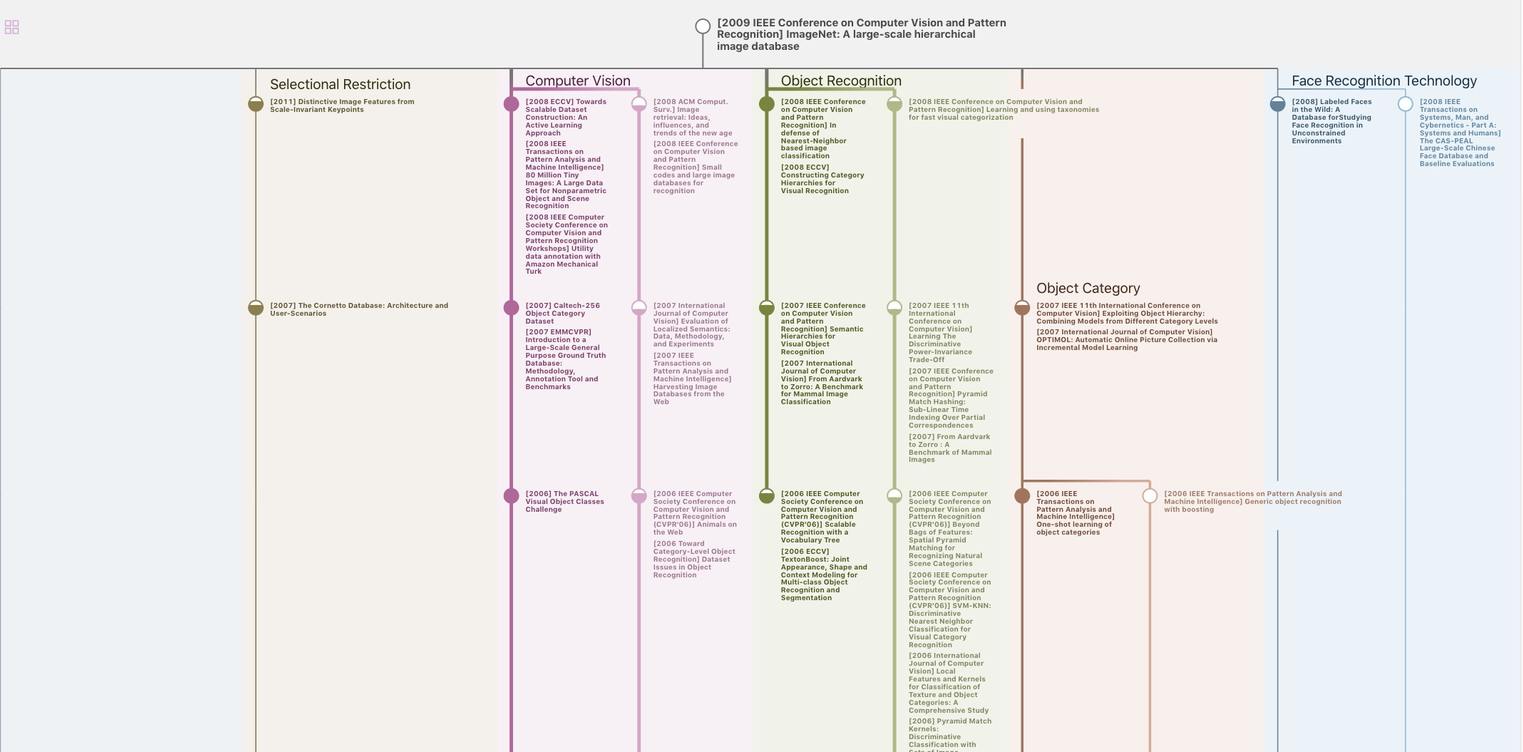
生成溯源树,研究论文发展脉络
Chat Paper
正在生成论文摘要