When Decentralized Optimization Meets Federated Learning
IEEE NETWORK(2023)
摘要
Federated learning is a new learning paradigm for extracting knowledge from distributed data. Due to its favorable properties in preserving privacy and saving communication costs, it has been extensively studied and widely applied to numerous data analysis applications. However, most existing federated learning approaches concentrate on the centralized setting, which is vulnerable to a single-point failure. An alternative strategy for addressing this issue is the decentralized communication topology. In this article, we systematically investigate the challenges and opportunities when renovating decentralized optimization for federated learning. In particular, we discussed them from the model, data, and communication sides, respectively, which can deepen our understanding about decentralized federated learning.
更多查看译文
关键词
Optimization,Data models,Computational modeling,Adaptation models,Servers,Convergence,Stochastic processes,Federated learning,Distributed databases,Information retrieval,Knowledge acquisition,Decentralized applications,Topology,Communication systems
AI 理解论文
溯源树
样例
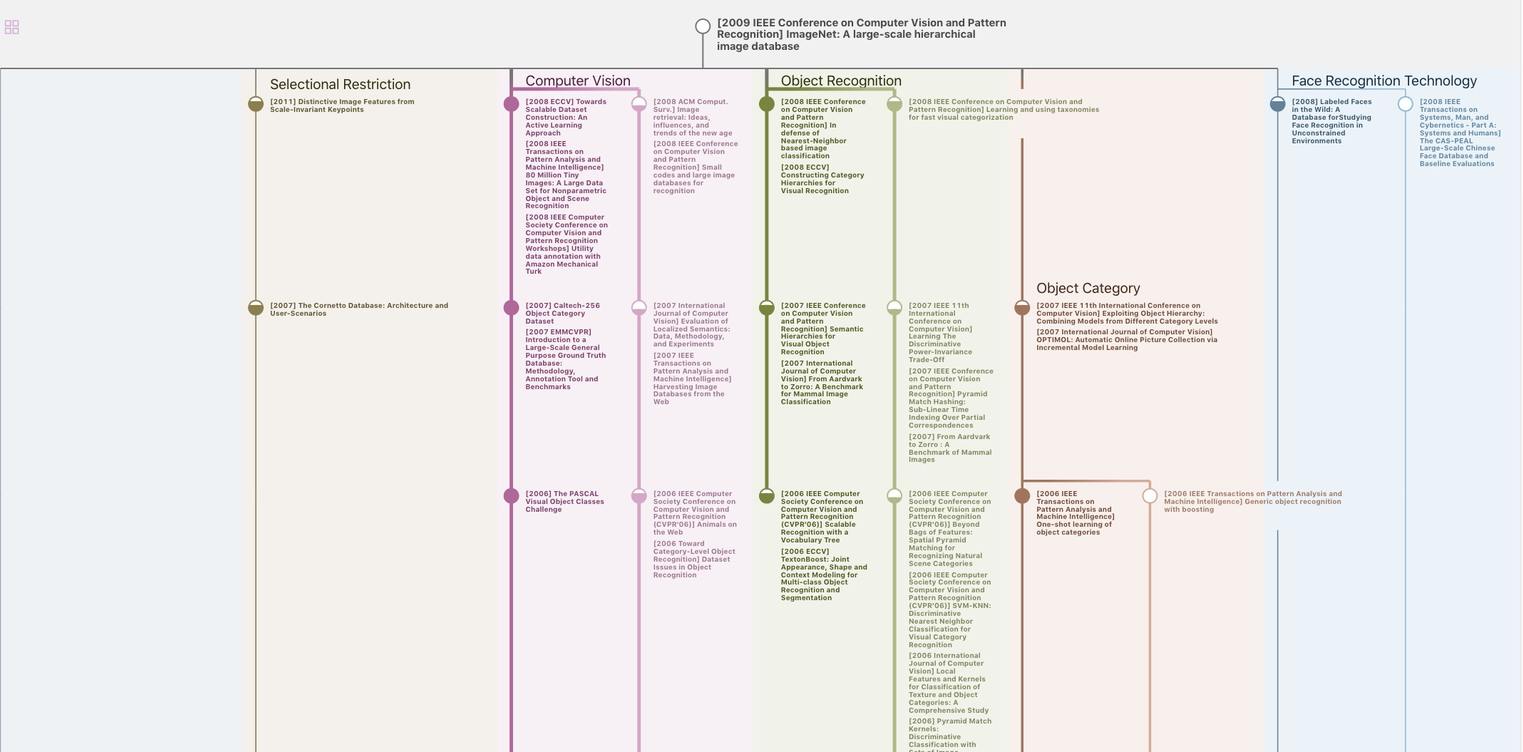
生成溯源树,研究论文发展脉络
Chat Paper
正在生成论文摘要