Leveraging Generative Models to Recover Variable Names from Stripped Binary
arxiv(2023)
摘要
Decompilation aims to recover the source code form of a binary executable. It
has many security applications such as malware analysis, vulnerability
detection and code hardening. A prominent challenge in decompilation is to
recover variable names. We propose a novel technique that leverages the
strengths of generative models while suppressing potential hallucinations and
overcoming the input token limitation. We build a prototype, GenNm, from a
pre-trained generative model Code-Llama. We fine-tune GenNm on decompiled
functions, and leverage program analysis to validate the results produced by
the generative model. GenNm includes names from callers and callees while
querying a function, providing rich contextual information within the model's
input token limitation. Our results show that GenNm improves the
state-of-the-art from 48.1
query function is not seen in the training dataset.
更多查看译文
AI 理解论文
溯源树
样例
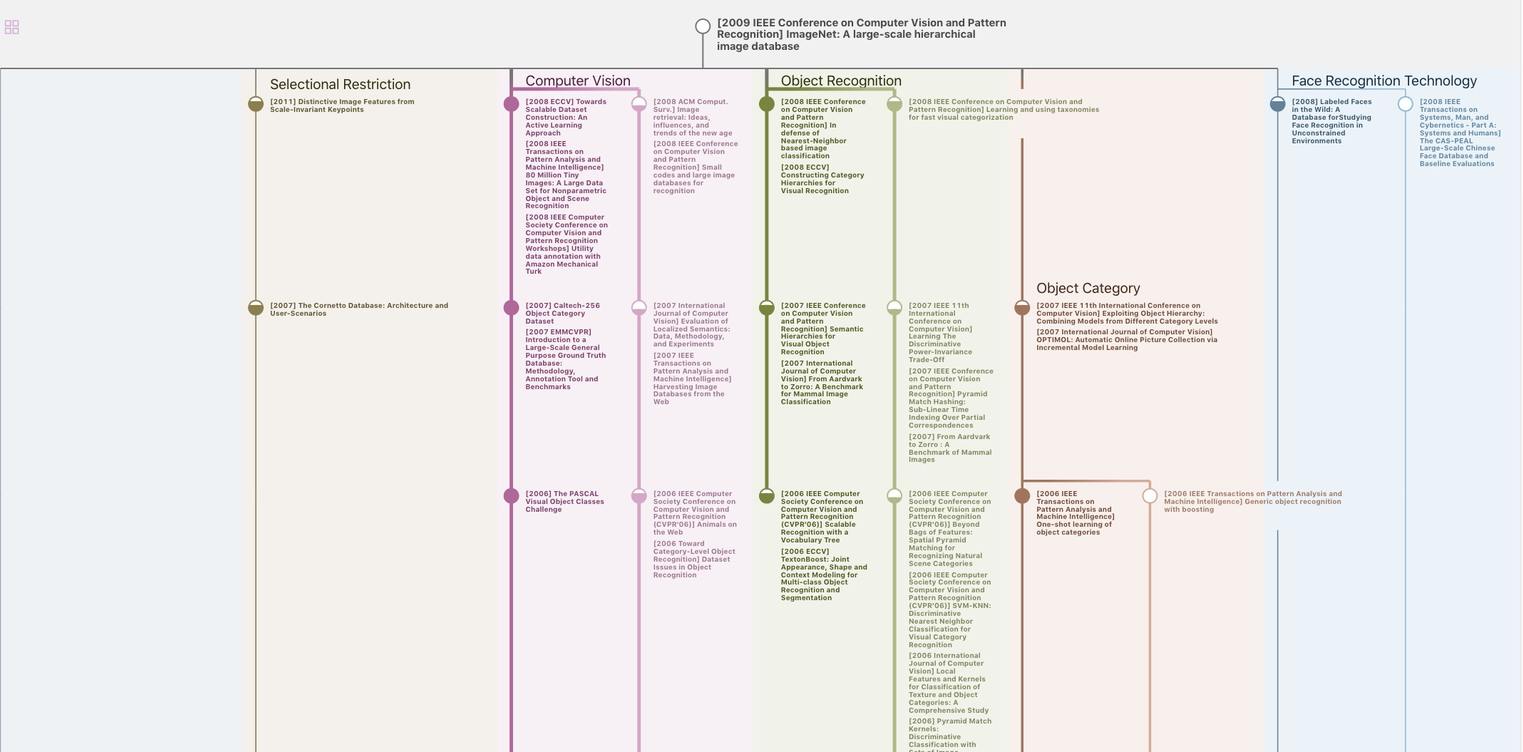
生成溯源树,研究论文发展脉络
Chat Paper
正在生成论文摘要