Correcting auto-differentiation in neural-ODE training
CoRR(2023)
摘要
Does the use of auto-differentiation yield reasonable updates to deep neural networks that represent neural ODEs? Through mathematical analysis and numerical evidence, we find that when the neural network employs high-order forms to approximate the underlying ODE flows (such as the Linear Multistep Method (LMM)), brute-force computation using auto-differentiation often produces non-converging artificial oscillations. In the case of Leapfrog, we propose a straightforward post-processing technique that effectively eliminates these oscillations, rectifies the gradient computation and thus respects the updates of the underlying flow.
更多查看译文
关键词
training,auto-differentiation,neural-ode
AI 理解论文
溯源树
样例
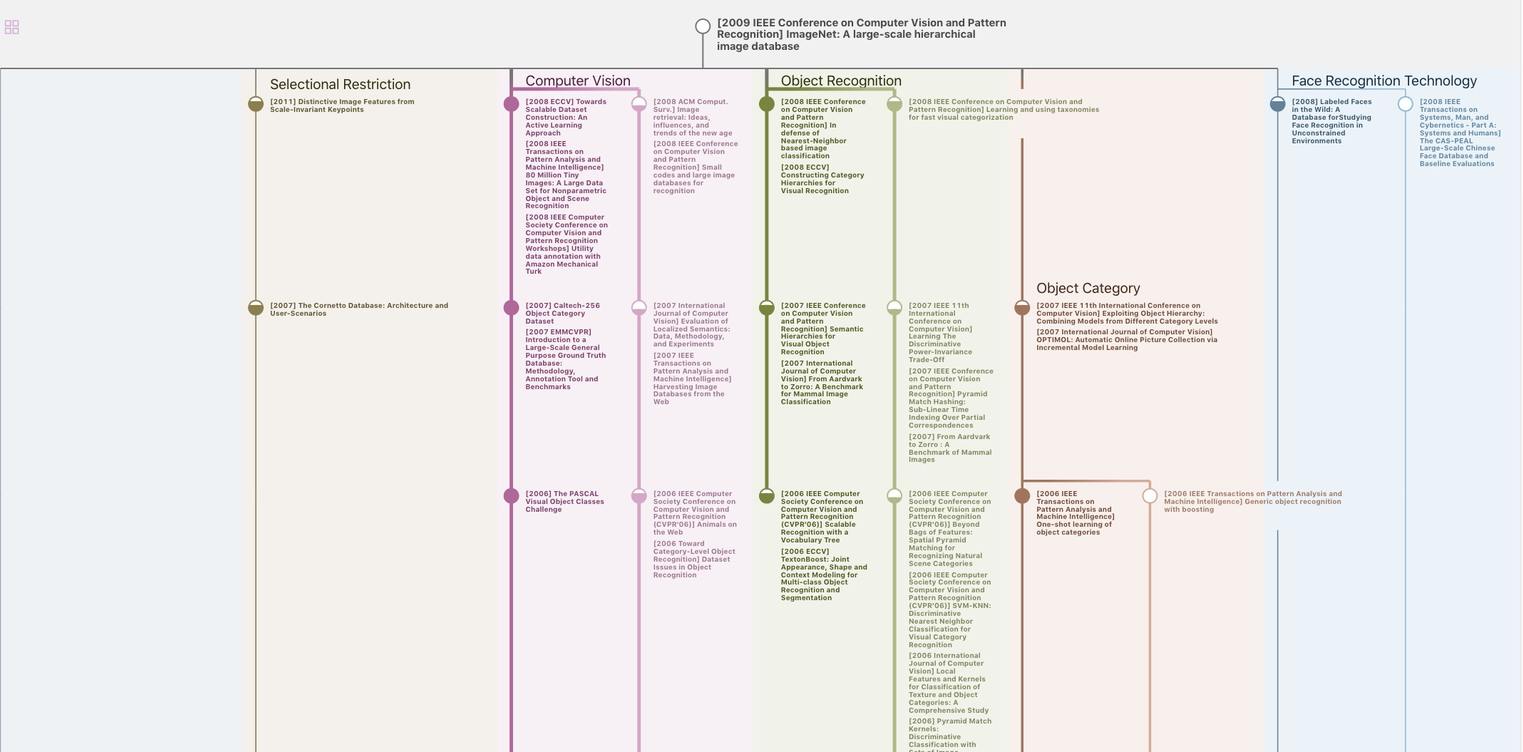
生成溯源树,研究论文发展脉络
Chat Paper
正在生成论文摘要