On size-independent sample complexity of ReLU networks
INFORMATION PROCESSING LETTERS(2024)
摘要
We study the sample complexity of learning ReLU neural networks from the point of view of generalization. Given norm constraints on the weight matrices, a common approach is to estimate the Rademacher complexity of the associated function class. Previously [9] obtained a bound independent of the network size (scaling with a product of Frobenius norms) except for a factor of the square -root depth. We give a refinement which often has no explicit depth -dependence at all.
更多查看译文
关键词
Neural networks,Rademacher complexity,Generalization,Theory of computation
AI 理解论文
溯源树
样例
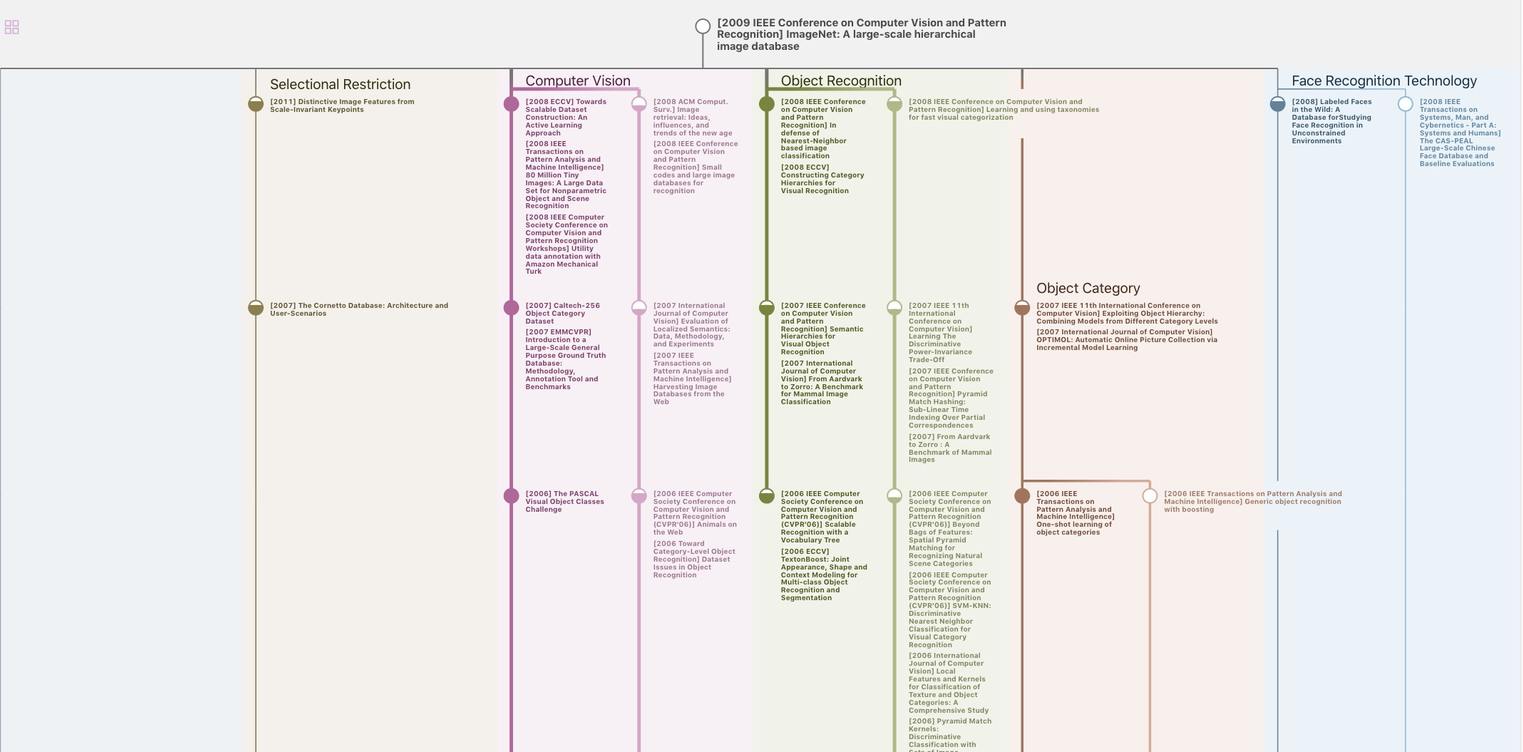
生成溯源树,研究论文发展脉络
Chat Paper
正在生成论文摘要