Can Contextual Biasing Remain Effective with Whisper and GPT-2?
CoRR(2023)
摘要
End-to-end automatic speech recognition (ASR) and large language models, such as Whisper and GPT-2, have recently been scaled to use vast amounts of training data. Despite the large amount of training data, infrequent content words that occur in a particular task may still exhibit poor ASR performance, with contextual biasing a possible remedy. This paper investigates the effectiveness of neural contextual biasing for Whisper combined with GPT-2. Specifically, this paper proposes integrating an adapted tree-constrained pointer generator (TCPGen) component for Whisper and a dedicated training scheme to dynamically adjust the final output without modifying any Whisper model parameters. Experiments across three datasets show a considerable reduction in errors on biasing words with a biasing list of 1000 words. Contextual biasing was more effective when applied to domain-specific data and can boost the performance of Whisper and GPT-2 without losing their generality.
更多查看译文
关键词
contextual biasing,whisper
AI 理解论文
溯源树
样例
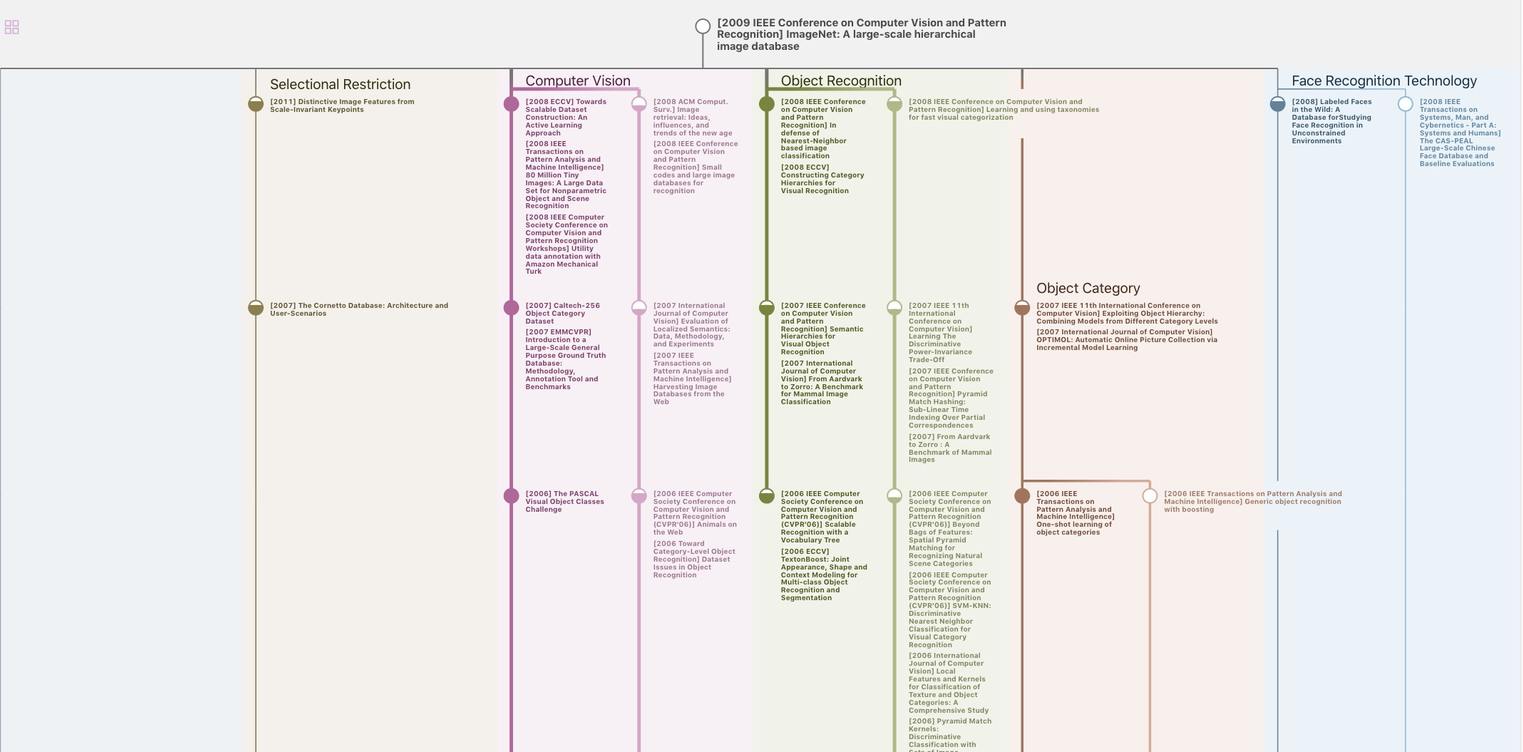
生成溯源树,研究论文发展脉络
Chat Paper
正在生成论文摘要