Handling Label Uncertainty on the Example of Automatic Detection of Shepherd's Crook RCA in Coronary CT Angiography
CoRR(2023)
摘要
Coronary artery disease (CAD) is often treated minimally invasively with a catheter being inserted into the diseased coronary vessel. If a patient exhibits a Shepherd's Crook (SC) Right Coronary Artery (RCA) - an anatomical norm variant of the coronary vasculature - the complexity of this procedure is increased. Automated reporting of this variant from coronary CT angiography screening would ease prior risk assessment. We propose a 1D convolutional neural network which leverages a sequence of residual dilated convolutions to automatically determine this norm variant from a prior extracted vessel centerline. As the SC RCA is not clearly defined with respect to concrete measurements, labeling also includes qualitative aspects. Therefore, 4.23% samples in our dataset of 519 RCA centerlines were labeled as unsure SC RCAs, with 5.97% being labeled as sure SC RCAs. We explore measures to handle this label uncertainty, namely global/model-wise random assignment, exclusion, and soft label assignment. Furthermore, we evaluate how this uncertainty can be leveraged for the determination of a rejection class. With our best configuration, we reach an area under the receiver operating characteristic curve (AUC) of 0.938 on confident labels. Moreover, we observe an increase of up to 0.020 AUC when rejecting 10% of the data and leveraging the labeling uncertainty information in the exclusion process.
更多查看译文
关键词
coronary detection angiography,label uncertainty,automatic detection,shepherd
AI 理解论文
溯源树
样例
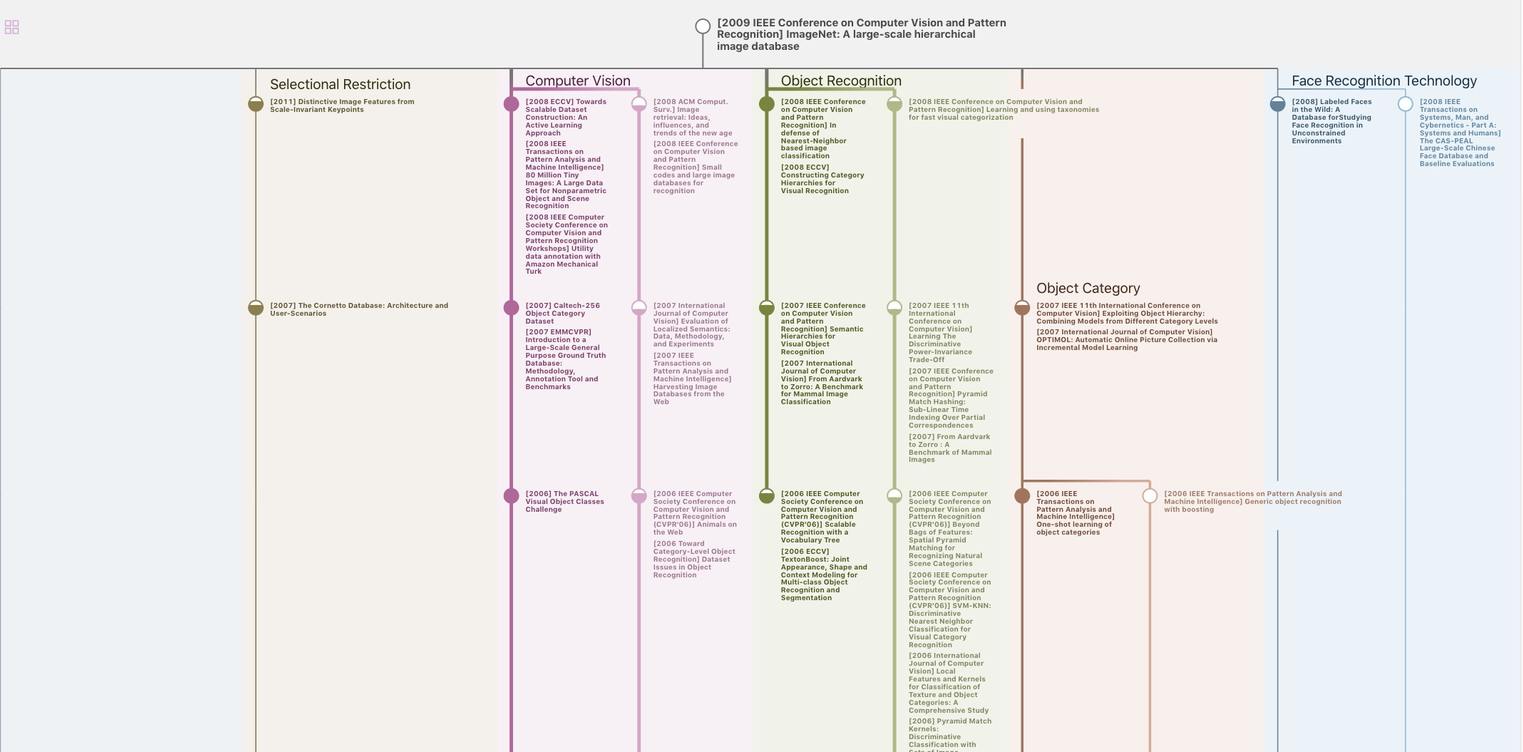
生成溯源树,研究论文发展脉络
Chat Paper
正在生成论文摘要