On the minimum information checkerboard copulas under fixed Kendall's rank correlation
arxiv(2023)
摘要
Copulas have gained widespread popularity as statistical models to represent
dependence structures between multiple variables in various applications. The
minimum information copula, given a finite number of constraints in advance,
emerges as the copula closest to the uniform copula when measured in
Kullback-Leibler divergence. In prior research, the focus has predominantly
been on constraints related to expectations on moments, including Spearman's
ρ. This approach allows for obtaining the copula through convex
programming. However, the existing framework for minimum information copulas
does not encompass non-linear constraints such as Kendall's τ. To address
this limitation, we introduce MICK, a novel minimum information copula under
fixed Kendall's τ. We first characterize MICK by its local dependence
property. Despite being defined as the solution to a non-convex optimization
problem, we demonstrate that the uniqueness of this copula is guaranteed when
the correlation is sufficiently small. Additionally, we provide numerical
insights into applying MICK to real financial data.
更多查看译文
AI 理解论文
溯源树
样例
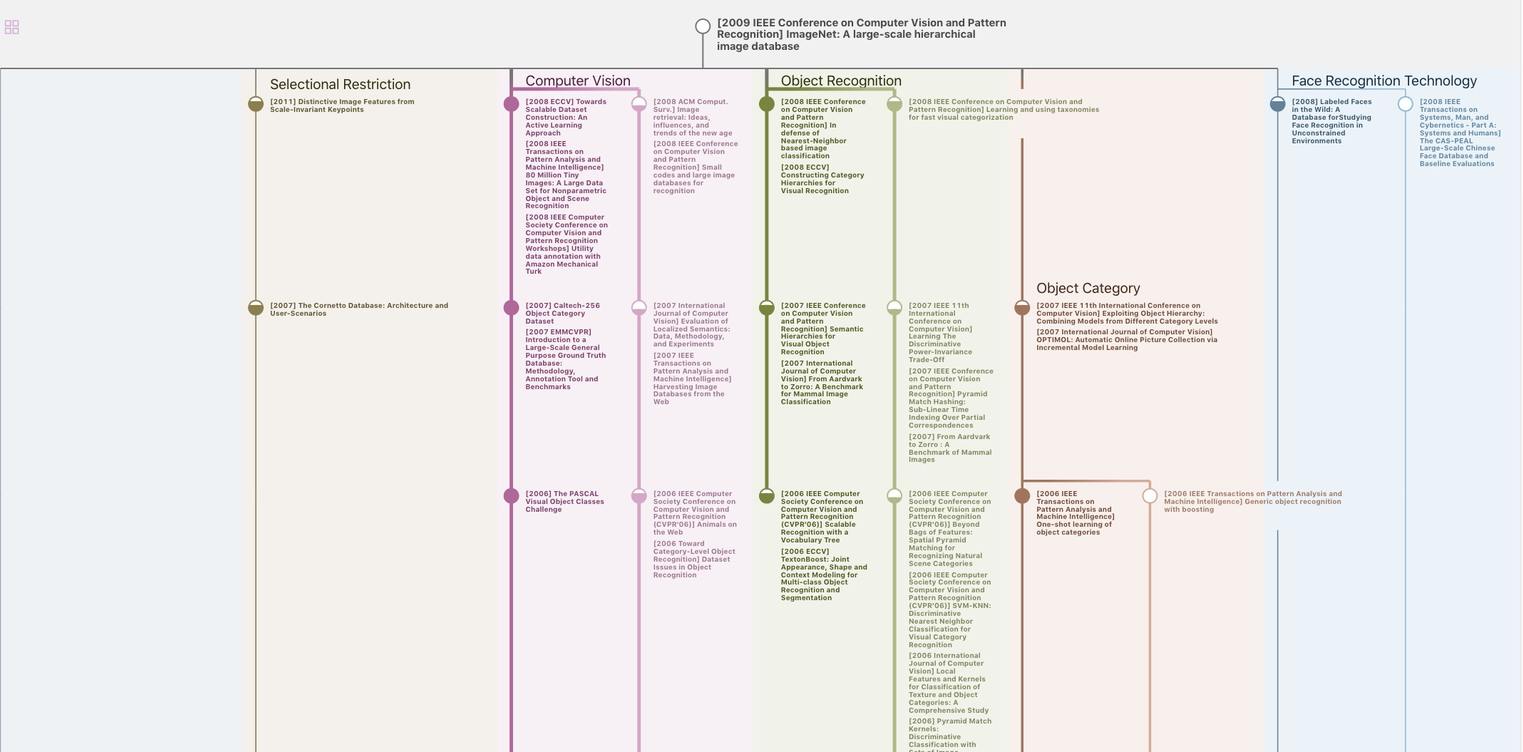
生成溯源树,研究论文发展脉络
Chat Paper
正在生成论文摘要