Learning Local to Global Feature Aggregation for Speech Emotion Recognition
CoRR(2023)
摘要
Transformer has emerged in speech emotion recognition (SER) at present. However, its equal patch division not only damages frequency information but also ignores local emotion correlations across frames, which are key cues to represent emotion. To handle the issue, we propose a Local to Global Feature Aggregation learning (LGFA) for SER, which can aggregate longterm emotion correlations at different scales both inside frames and segments with entire frequency information to enhance the emotion discrimination of utterance-level speech features. For this purpose, we nest a Frame Transformer inside a Segment Transformer. Firstly, Frame Transformer is designed to excavate local emotion correlations between frames for frame embeddings. Then, the frame embeddings and their corresponding segment features are aggregated as different-level complements to be fed into Segment Transformer for learning utterance-level global emotion features. Experimental results show that the performance of LGFA is superior to the state-of-the-art methods.
更多查看译文
关键词
global feature aggregation,speech emotion
AI 理解论文
溯源树
样例
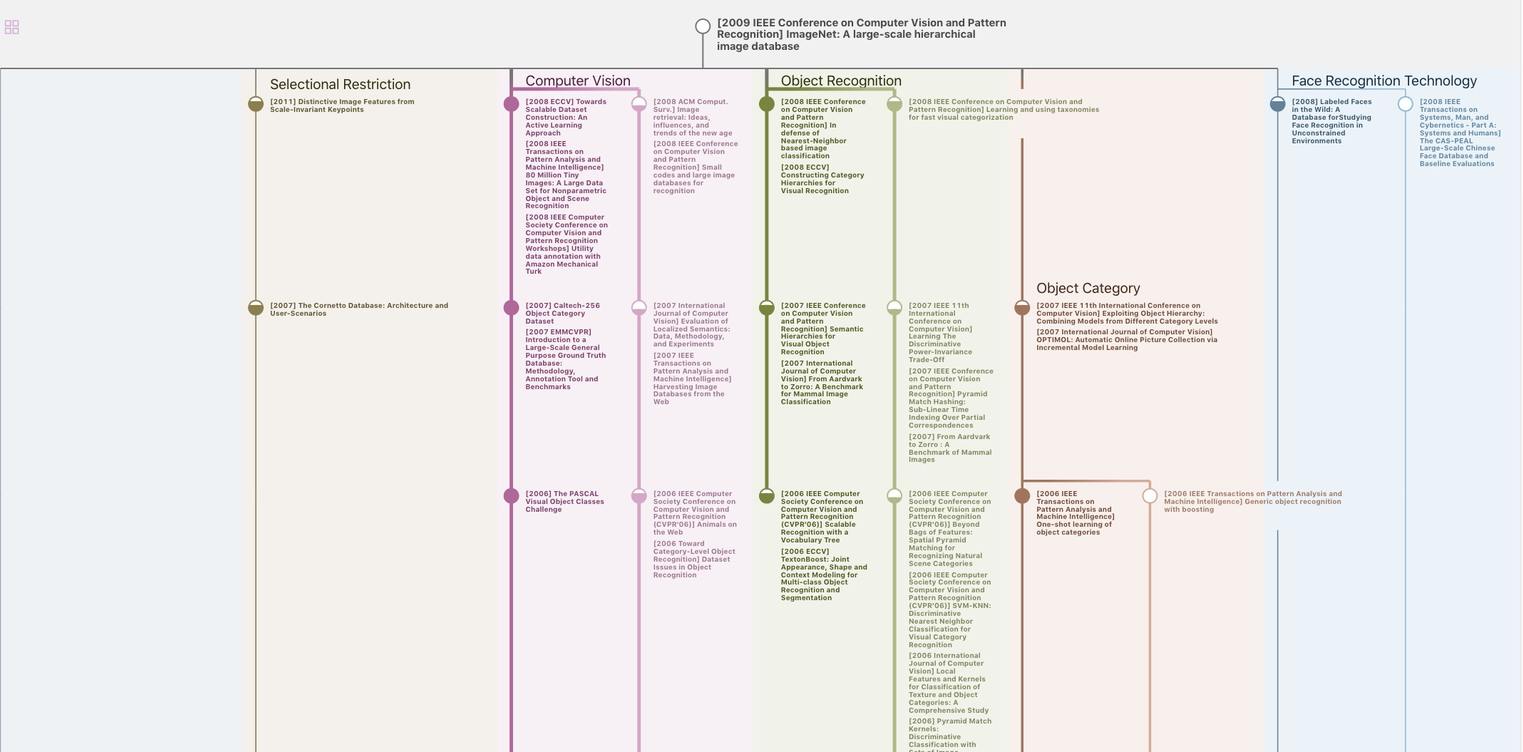
生成溯源树,研究论文发展脉络
Chat Paper
正在生成论文摘要