Leveraging Auxiliary Domain Parallel Data in Intermediate Task Fine-tuning for Low-resource Translation
CoRR(2023)
摘要
NMT systems trained on Pre-trained Multilingual Sequence-Sequence (PMSS) models flounder when sufficient amounts of parallel data is not available for fine-tuning. This specifically holds for languages missing/under-represented in these models. The problem gets aggravated when the data comes from different domains. In this paper, we show that intermediate-task fine-tuning (ITFT) of PMSS models is extremely beneficial for domain-specific NMT, especially when target domain data is limited/unavailable and the considered languages are missing or under-represented in the PMSS model. We quantify the domain-specific results variations using a domain-divergence test, and show that ITFT can mitigate the impact of domain divergence to some extent.
更多查看译文
关键词
auxiliary domain parallel data,intermediate task
AI 理解论文
溯源树
样例
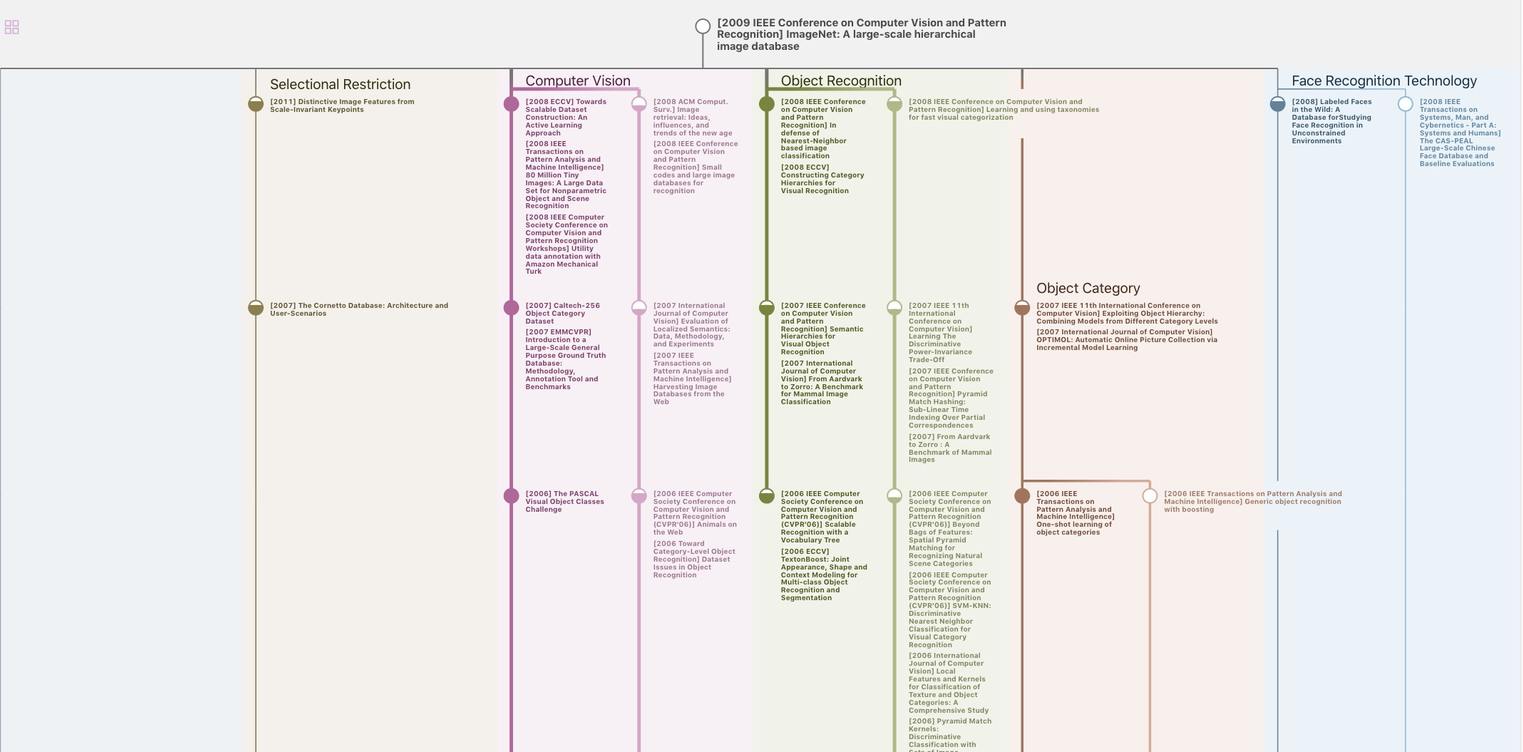
生成溯源树,研究论文发展脉络
Chat Paper
正在生成论文摘要