DSHGT: Dual-Supervisors Heterogeneous Graph Transformer -- A pioneer study of using heterogeneous graph learning for detecting software vulnerabilities
CoRR(2023)
摘要
Vulnerability detection is a critical problem in software security and attracts growing attention both from academia and industry. Traditionally, software security is safeguarded by designated rule-based detectors that heavily rely on empirical expertise, requiring tremendous effort from software experts to generate rule repositories for large code corpus. Recent advances in deep learning, especially Graph Neural Networks (GNN), have uncovered the feasibility of automatic detection of a wide range of software vulnerabilities. However, prior learning-based works only break programs down into a sequence of word tokens for extracting contextual features of codes, or apply GNN largely on homogeneous graph representation (e.g., AST) without discerning complex types of underlying program entities (e.g., methods, variables). In this work, we are one of the first to explore heterogeneous graph representation in the form of Code Property Graph and adapt a well-known heterogeneous graph network with a dual-supervisor structure for the corresponding graph learning task. Using the prototype built, we have conducted extensive experiments on both synthetic datasets and real-world projects. Compared with the state-of-the-art baselines, the results demonstrate promising effectiveness in this research direction in terms of vulnerability detection performance (average F1 improvements over 10\% in real-world projects) and transferability from C/C++ to other programming languages (average F1 improvements over 11%).
更多查看译文
关键词
heterogeneous graph,dual-supervisors
AI 理解论文
溯源树
样例
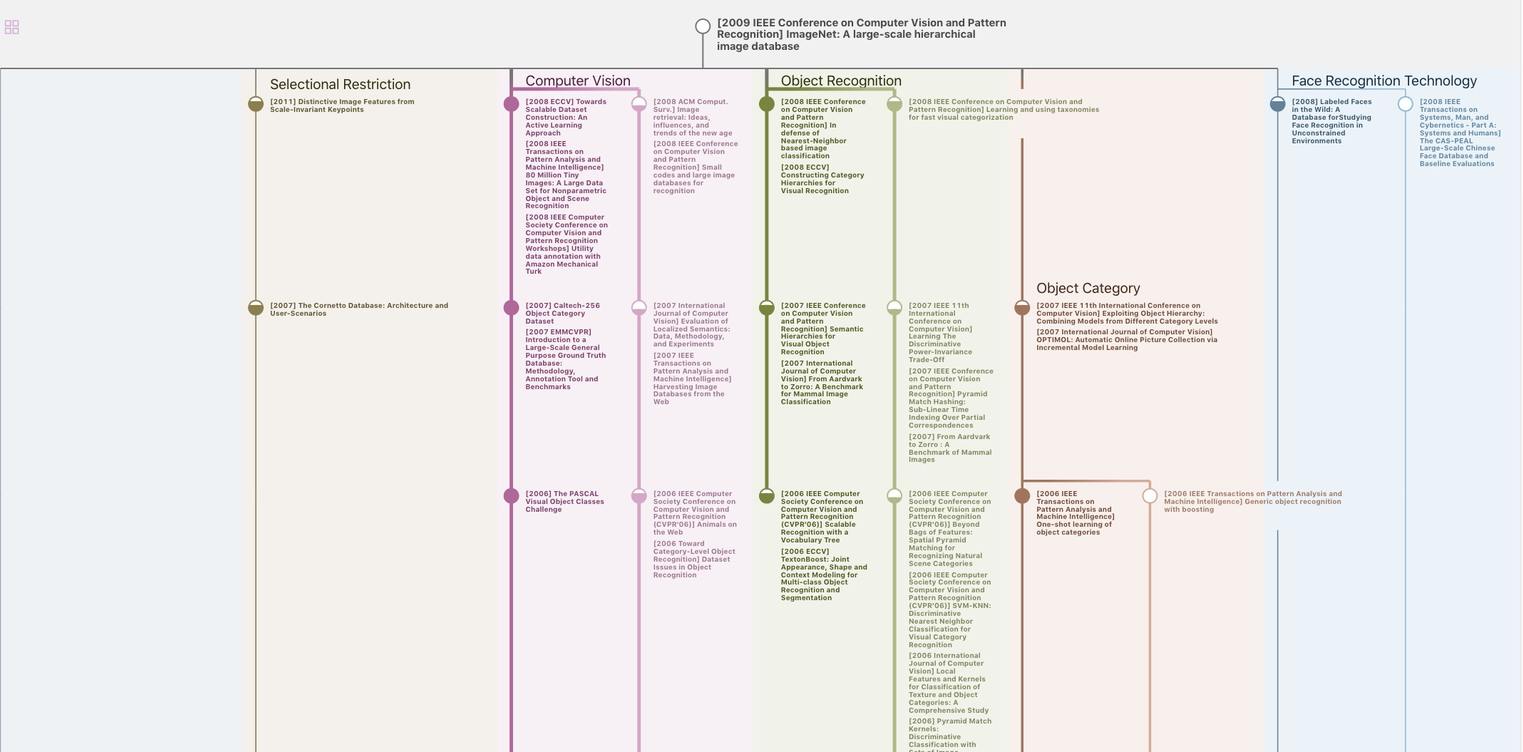
生成溯源树,研究论文发展脉络
Chat Paper
正在生成论文摘要